Business Analytics & Reporting
MINE CUSTOMER CALL DATA TO GAIN 100X MORE VALUABLE INSIGHTS INTO YOUR BUSINESS
Our leading AI engine automatically extracts a wide range of analytics from your unstructured omnichannel customer data; from call center data and QA reports to valuable business insights like customer and product analytics.
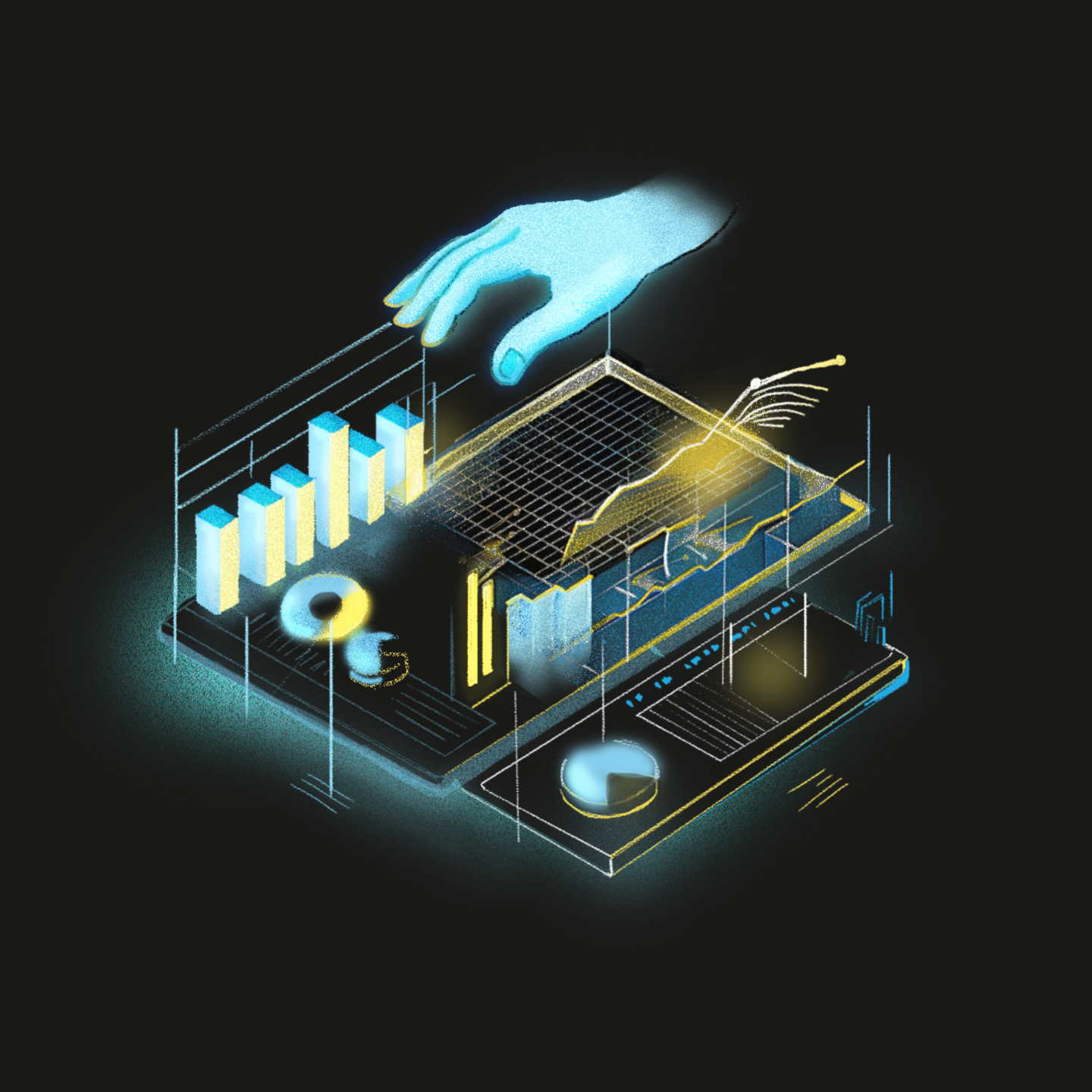
The Need For Unified Contact Center Analytics
Level AI has been a pleasure to work with. The user interface and tool are easy to use with practical features built-in. Their conversation intelligence platform is unlike anything we have seen in this space. We truly see Level AI as a partner.
Key Features
Omnichannel CX Insights
See exactly what’s happening across voice calls, email, and chat conversations – from agent performance to QA scores of individual agents or teams

Uncover Critical Insights With Custom Reports
Generate custom reports, track your most important metrics, apply filters, group data, and much more with Level AI’s Chart Builder

Keep Your Stakeholders On The Same Page
Level AI's VoC (Voice of Customer) can automatically deliver custom reports to contact center leaders, as well as other business teams, such as product and compliance

Integrate With Your Favorite Data Analytics Tools
Combine your contact center data with your favorite analytics and business intelligence tools. The Level AI API allows you to seamlessly push large amounts of data to and from the platform.

More Resources

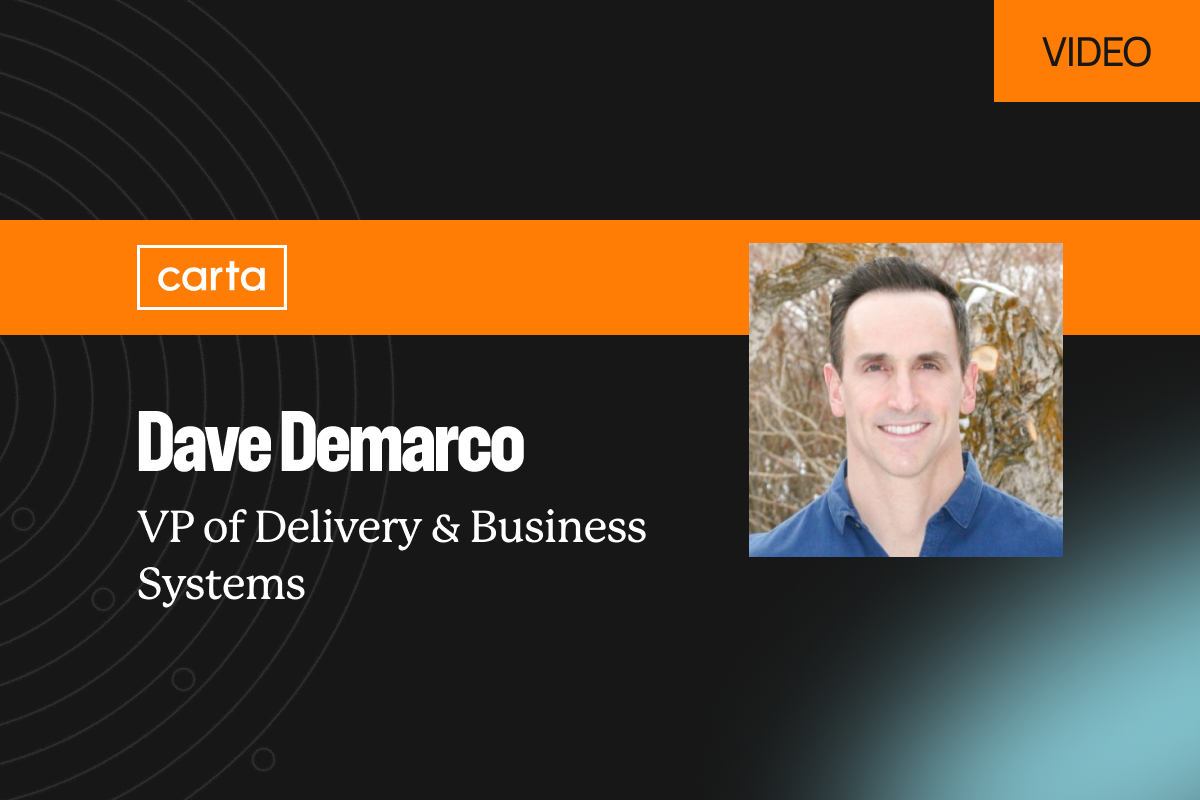
Frequently Asked Questions
Conversation analytics is the process of analyzing and understanding the data generated from conversations between people. It involves using various techniques such as natural language processing and understanding (NLP/NLU), machine learning (ML), and data analytics to extract insights from conversations, typically those that occur in customer service interactions or sales calls.
The goal of conversation analytics is to uncover patterns and trends in customer behavior and sentiment that can inform business decisions. For example, by analyzing call transcripts and customer feedback, businesses can identify common issues or concerns that customers have and take steps to address them, improving overall customer satisfaction and loyalty.
Conversation analytics can also be used to measure the effectiveness of tele-marketing campaigns, identify areas for improvement in sales processes, and optimize customer support operations. It relies heavily on automated tools to process large volumes of data quickly and accurately, making it a valuable tool for businesses looking to gain insights from customer interactions.
Contact Center Analytics are the analysis of standard contact center metrics to identify trends, impacts, causes, and results. Contact centers regularly need to record, measure, and analyze different types of data.
The four most common contact center analytics consist of the following:
- Contact Center Analytics
- Customer Analytics
- Speech Analytics
- Predictive Analytics
Contact center team analytics measure the performance of agents and quality assurance personnel. Internal contact center teams focus on different metrics that are relevant to the industry of their product or service.
For contact centers, common performance metrics for internal teams include:
- ASA = Average Speed of Answering
- FCR = First-Call Resolution
- AHT = Average Handle Time
- CSAT = Customer Satisfaction Score
- NPS = Net Promoter ScoreSM
- CES = Customer Effort Score
Customer analytics is the analysis of multiple customer-related data sources to identify customer trends, interaction opportunities, and to serve as a source for modeling.
Customer analytics can be historical or predictive. Data sources include the voice of the customer, behavior data, demographics, and purchase data.
Speech analytics are the analysis of transcribed voice and ingested text engagements, along with metadata including CRM (customer relationship management) and notes to identify trends, the voice of the customer insights, performance drivers, and other insights.
Advanced speech analytics are derived from both NLP (natural language processing) and NLU (natural language understanding) technologies.
Predictive analytics is the use of big data, artificial intelligence, advanced algorithms, and intuitive machine learning techniques to create predictions about future results based on historical data.
In addition, predictive analytics allows you to calculate expected (CLTV) customer lifetime value, anticipate future consumer behavior, and identify customers likely to churn.
Call Center Performance Management is a program, generally led by a contact center operations manager, dedicated to calculating and improving the performance of contact center agents.
Performance Management generally requires a number of tools like call recording, quality monitoring, coaching, and gamification.
Contact center managers can rely on KPIs (key performance indicators) to track metrics such as net promoter score (NPS), customer satisfaction score (CSAT), and average handle time (AHT) to both measure and improve contact center performance.
However, advanced software is essential to improving the performance of a modern contact center at scale.
Here are 6 of the most effective ways to improve contact center performance:
- Advanced AI and NLU: Upgrade your speech analytics software with modern AI and NLU (natural language understanding) that recognizes customer intent and doesn’t rely on outdated keyword matching.
- Omnichannel Analytics: Take an omnichannel approach to your call center’s data with a platform such as Level AI.
- Contact Center Management Trends: Keep up on the latest trends for contact centers, such as adopting machine learning platforms able to provide 100% coverage of support conversations.
- Smart Data: Use conversational intelligence data to identify your best scripts and incorporate them into your overall quality assurance and agent performance strategy.
- Accurately Monitor and Score Communications: Use CCaaS (contact center as a service) platforms that can accurately monitor 100% of interactions based on real scenarios and QA rubrics. Legacy QA software is only able to accurately monitor 1-2% of customer interactions on average.
- Augmented Quality Assurance: Take advantage of augmented QA to save QA managers time. Augmented QA allows agents to review their own performance and to learn autonomously.
The best way to assess your contact center’s performance is through a combination of quantitative and qualitative KPIs. Modern contact center technology, such as Level AI, is well equipped to give you all the data and conversational intelligence you need.
There are common contact center performance metrics, such as average handle time, customer wait time, schedule adherence and more. These kinds of call center metrics can help you to make informed decisions about workforce management and service level.
However, measuring customer satisfaction and the overall quality of the customer experience is more difficult, as CSAT and NPS scores are limited in scope.
A high CSAT score might come from someone who felt that their customer interaction was hurried and would have preferred more friendly service. A low NPS score could come from a caller who experienced wonderful agent engagement but didn’t get their issue resolved.
Fortunately, call center AI technologies such as real-time speech analytics and NLU (natural language understanding) that understand customer intent are available and should be taken advantage of. CCaaS platforms make it far easier to collect data on subjective factors such as a caller’s emotional state.
How do you incorporate AI and machine learning into a call center? AI now plays a major role in the most advanced QA teams. The key areas where AI technology in a contact center include quality assurance, agent monitoring and training, and analytics.
AI in customer service refers mostly to three core technologies:
- Machine learning or AI (artificial intelligence)
- NLP (natural language processing)
- NLU (natural language understanding)
The most advanced contact center software platforms for QA and agent training are using NLU (natural language understanding), as opposed to NLP (natural language processing) alone.
For example, Level AI is using NLU to uncover customer intent and to provide support agents with the information they need to communicate to customers in real-time.
In fact, in 2022, the best AI-powered contact center software outperforms legacy systems, which rely on keyword matching (text mining), with an average 2X (+100%) lift in accuracy.
The most advanced AI and NLU platforms for contact center QA teams will quickly learn how to best handle nearly any scenario your customer support agents encounter in real-time.