A Detailed Guide to Customer Sentiment Analysis

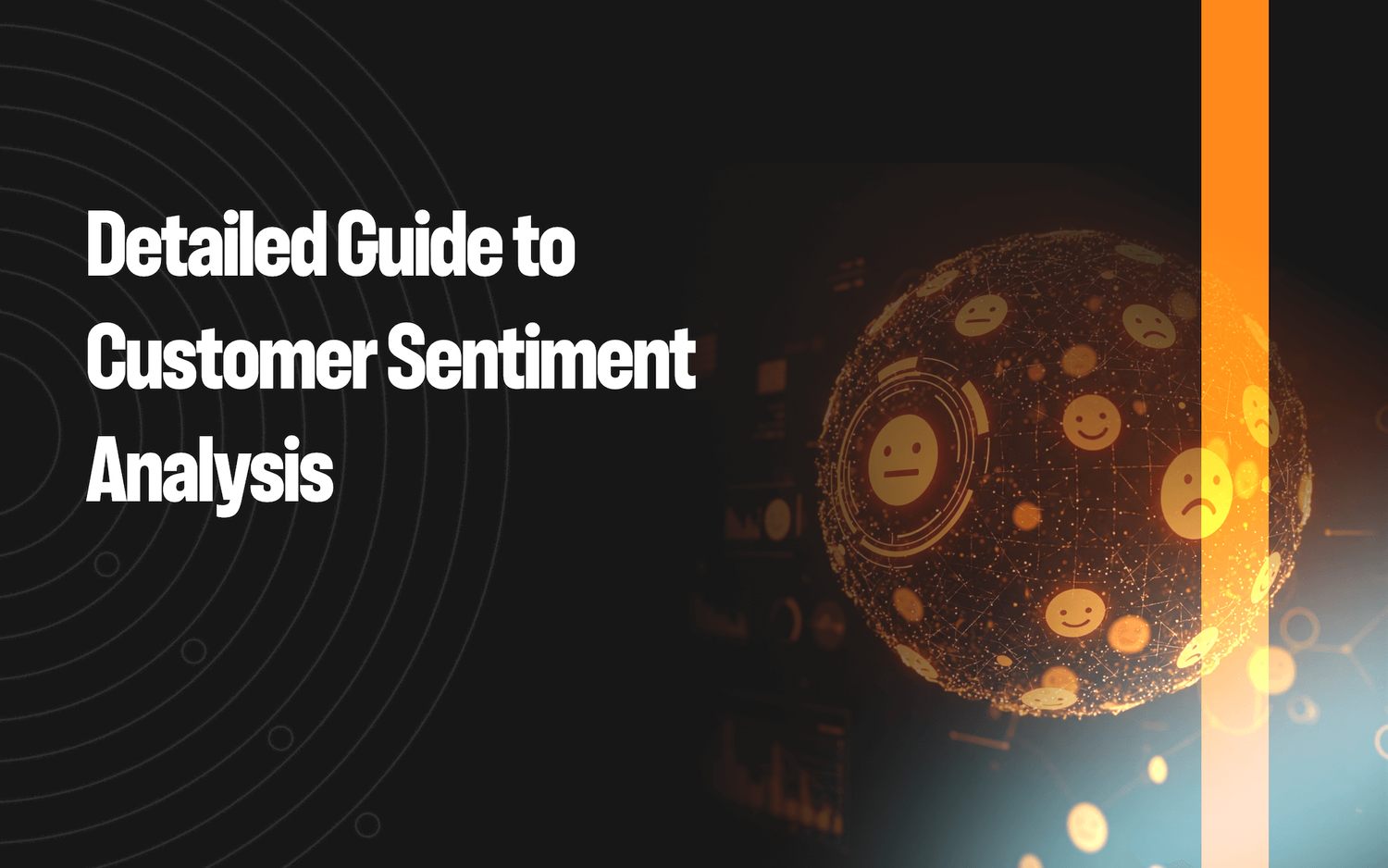
Customer sentiment analysis is the process of evaluating customers’ attitudes, opinions, and feelings toward a brand, product, or service across various touchpoints, including phone, email, chat, social media, etc.
It provides businesses with insights that marketers can analyze for upcoming marketing campaigns, product teams can apply to enhancements, and customer support teams can use to elevate service quality.
The data highlights customer needs and expectations, identifies recurring issues or agent training gaps, and points out what's working or not with your product or service.
Fortunately, there are several ways to tap into customer sentiment and perceptions, each offering unique insights into the customer experience.
In this guide, we'll explain how customer sentiment analysis works and how it’s measured, including how to evaluate sentiment using customer interactions, with features and examples from our own customer experience platform, Level AI.
How Does Customer Sentiment Analysis Work?
You start by gathering data across all possible channels to see what customers are saying. That includes sending out surveys, listening to social media posts, reading online reviews or support interactions, or listening to calls.
You then analyze the feedback using manual or automated methods. Evaluating feedback manually can be slow and time-consuming, not to mention incomplete, inaccurate, and sometimes biased. Automated methods include using text analysis, natural language processing (NLP), artificial intelligence, and machine learning to recognize and interpret customer sentiment within the data.
AI algorithms use NLP to identify and classify sentiment — such as positive, negative, or neutral — in specific feedback or interaction data, and to gauge the intensity of this sentiment.
There are various types of sentiment analysis to deliver more targeted and actionable insights. These include:
- Aspect based: Isolates specific elements, such as a product's price or service response time, and assesses sentiment towards each to guide focused improvements.
- Fine grained: Refines sentiment into levels, such as strongly positive or slightly negative, for a nuanced understanding of customer reactions.
- Emotion detection: Pinpoints emotions like anger or admiration within feedback, helping businesses address the feelings driving customer opinions.
- Intent analysis: Analyzes what customers plan to do, like buy, complain, or leave, enabling proactive steps to influence outcomes.
How Is Customer Sentiment Measured?
Direct Customer Feedback
Companies create surveys to ask targeted questions and gather specific insights about particular aspects of their products or services.
While surveys gather focused data, they face notable limitations. Survey recipients won’t always respond, and answers may be biased toward strongly satisfied or dissatisfied customers while missing valuable insights from customers in the middle range of satisfaction.
This leaves you with incomplete or skewed data and may only offer a partial view of how your customers feel about their interactions with your brand. As a result, opportunities to identify pain points and improve customer satisfaction and retention can be missed.
Survey software like SurveyMonkey is nonetheless extensively used and under many circumstances can be a good way of getting answers to questions you want to know about.
Read more about top SurveyMonkey alternatives.
Social Media Monitoring
Monitoring social media platforms is another way businesses keep tabs on brand reputation and what customers are saying about them. It’s possible to detect emotional trends and sentiment by tracking and reviewing comments, mentions, and customer reviews across different platforms.
It’s certainly possible to do this manually, but once your brand grows or you start monitoring multiple platforms this becomes incredibly difficult to track. Instead, you can turn to an AI-powered social monitoring tool (such as Meltwater or Brandwatch) that automatically scans posts for brand mentions and identifies positive, neutral, and negative sentiments.
The primary advantage of social media monitoring is capturing authentic, unsolicited feedback from a broad customer base. However, social data often comes with significant noise and can be skewed by vocal minorities (including non-customers) or viral events that don't represent overall customer sentiment.
Customer Interaction Data
Many companies are sitting on a trove of unstructured data from daily interactions across customer support channels and sales conversations.
Buried within this data are indications of what your customers are naturally expressing about your product, service, or brand, and how they feel about it.
However, this wealth of data has historically been difficult to analyze. Traditional methods relied on manually sampling just 1–2% of customer interactions, resulting in missed opportunities to address customer concerns.
Below, we show examples of how software, like Level AI's customer experience platform, analyzes sentiments expressed during customer interactions. We’ll also explore how sentiment analysis powers key features and AI use cases in contact center to improve agent performance and efficiency.
Understand What Customers Are Saying
Understanding what customers truly mean begins with accurately understanding both their words as well as the context in which these are expressed.
Some legacy customer sentiment analysis tools rely on simple keyword matching to detect intent, but this fails to capture the true meaning and context of customer communications. For instance, when a customer says "I love waiting on hold," a basic system might flag this as positive while completely missing the customer's sarcasm.
However, the challenges of keyword matching extend beyond misinterpreting customer frustrations. The systems require extensive manual effort to capture various ways customers might express the same sentiment.
Consider product feedback as an example. Customers might say "This isn't what I expected," "It doesn't match the photos," or "The quality is poor." All potential keywords or phrases related to an intent would need to be manually entered into the system to capture every variation of how customers might express dissatisfaction with a product they’ve received.
Sophisticated artificial intelligence systems like Level AI’s Scenario Engine can understand what customers and agents are saying to accurately identify intent.
Our system classifies a detected intent as a scenario (e.g., Account Issue, Billing Question, or Refund Request):
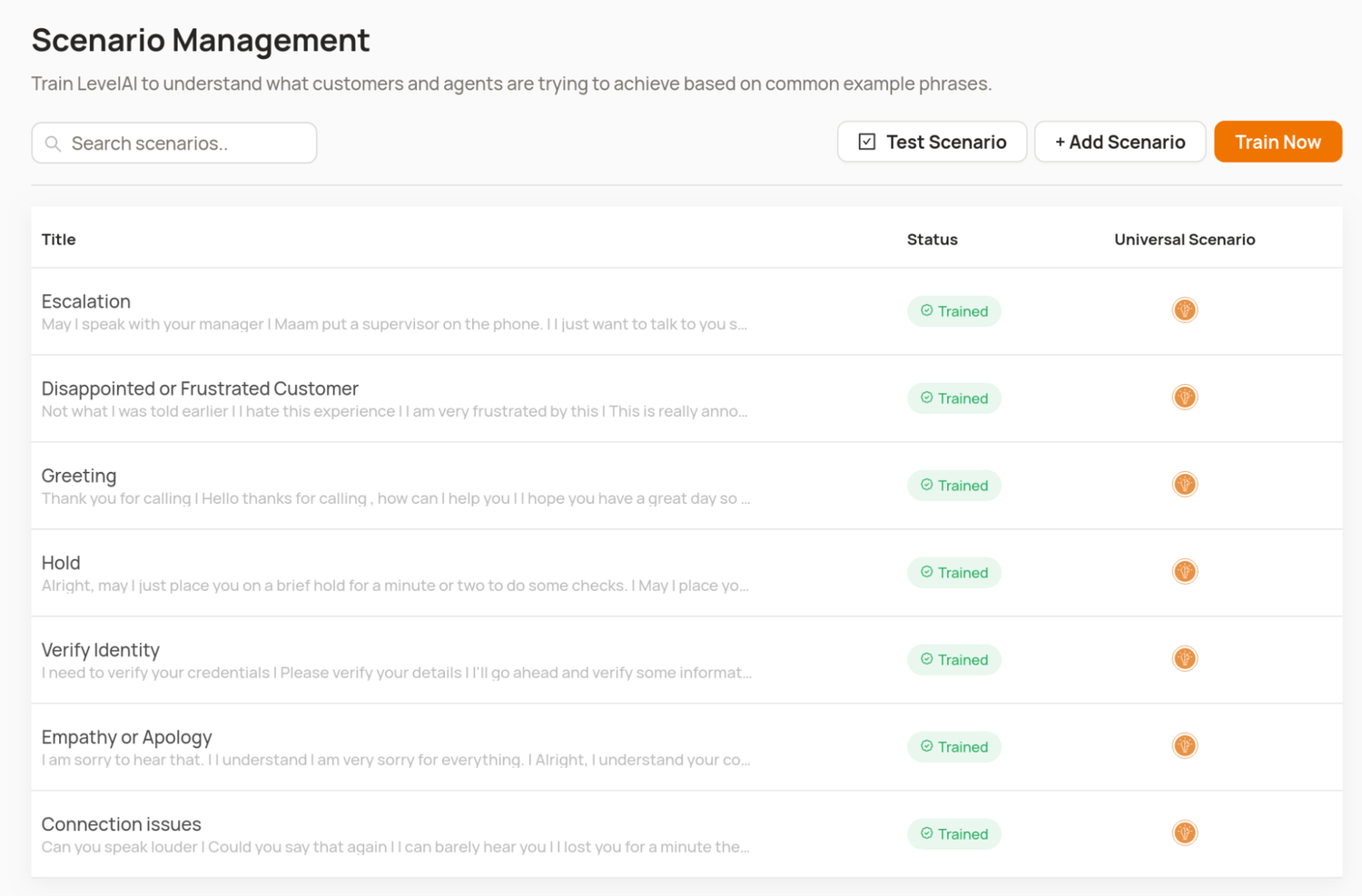
The software comes with several predefined out-of-the-box scenarios, but you can also create custom business-specific scenarios by providing example phrases that customers might use for a given intent.
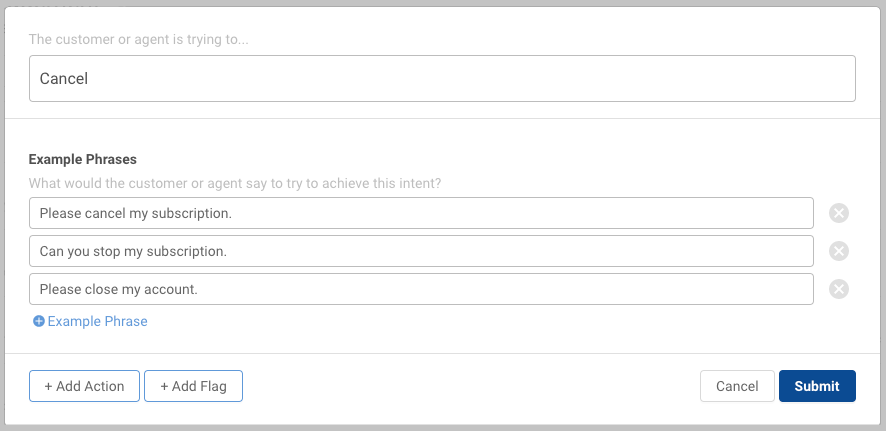
When our AI speech analytics software detects scenarios during interactions, Level AI labels these moments in the transcript with conversation tags.
These tags are searchable and filterable, making them especially useful for reporting. You can easily pull up conversations where a particular intent was expressed, such as instances where customers complained about a delayed delivery.
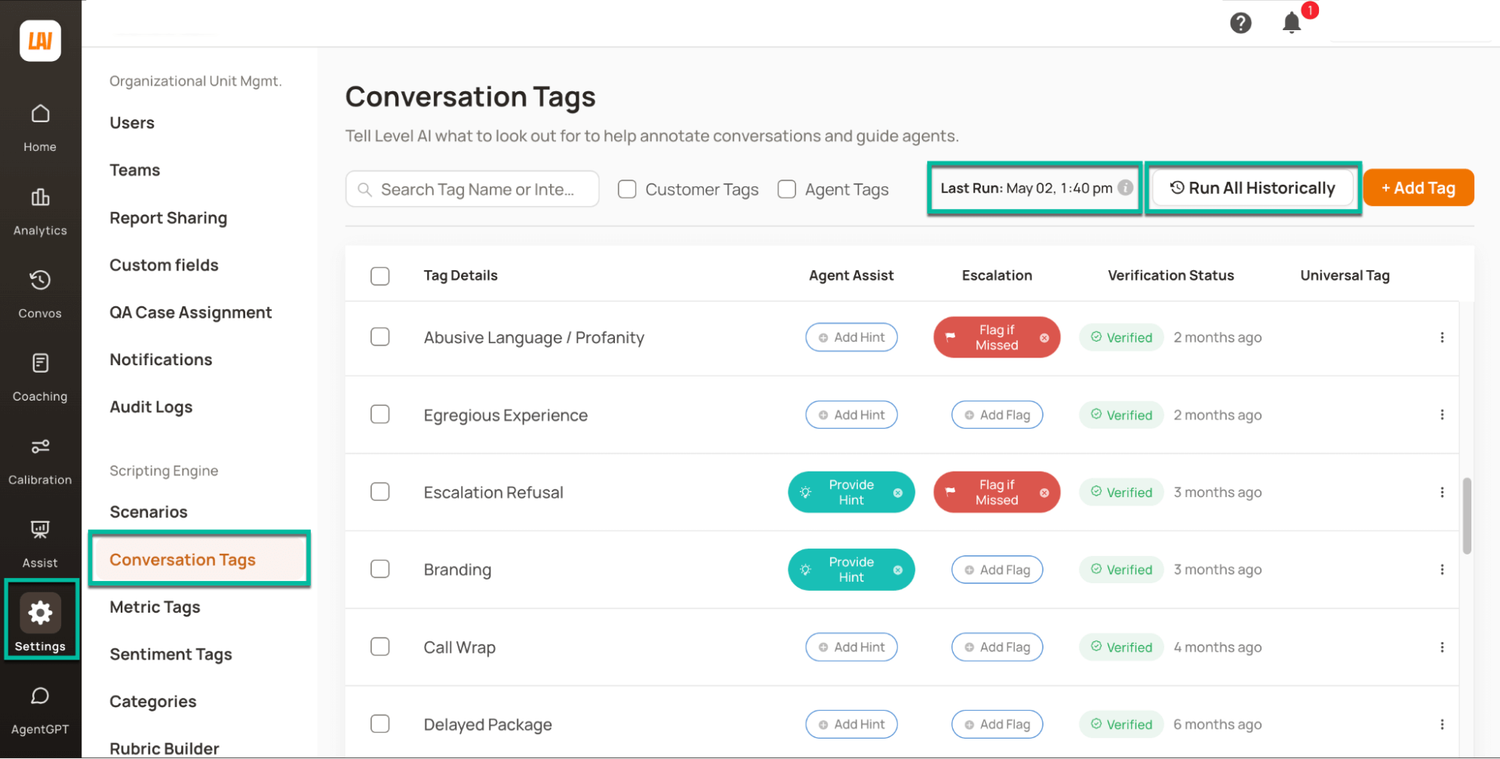
Accurately Gauge How Customers Feel
Our AI-powered software can detect the broadest range of customer emotions of any other product available today:
- Anger
- Annoyance
- Disapproval
- Disappointment
- Worry
- Happiness
- Admiration
- Gratitude
All interactions receive an overall Sentiment Score on a scale from 0 to 10, with 0 being strongly negative and 10 being strongly positive. The score includes all sentiments expressed, but different parts of the conversation are weighted more heavily In the calculation.
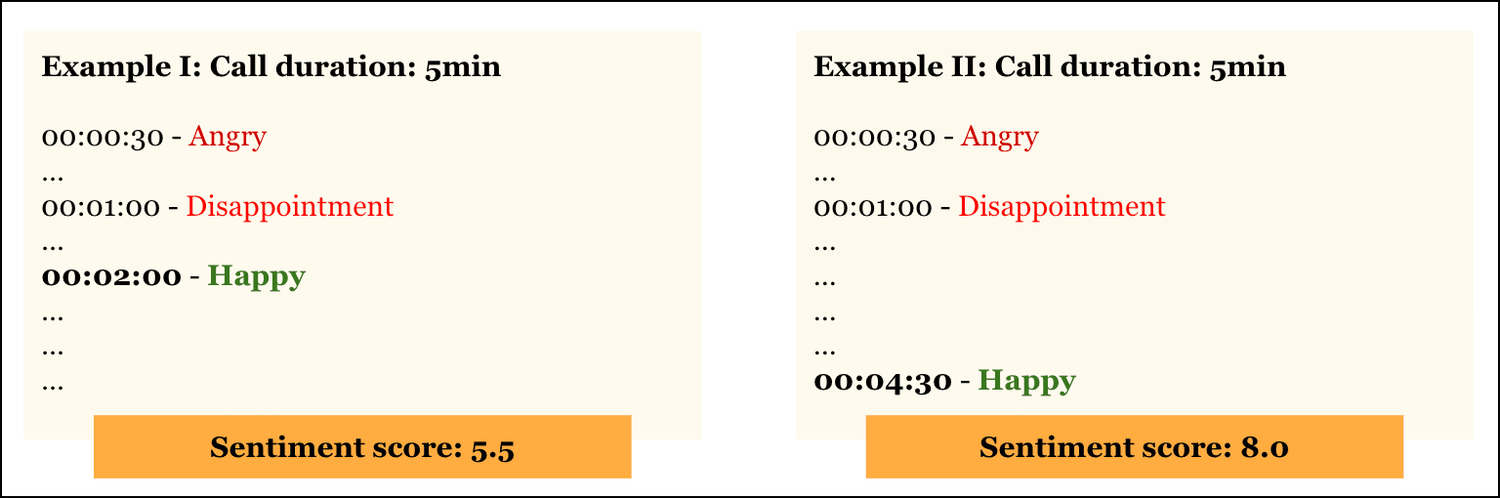
For example, sentiments that occur at the end of an interaction carry more weight in the overall score because this tends to better represent how the customer feels about the agent’s resolution to their problem.
By detecting a wide range of emotions during interactions, the system uncovers insights into how customers in the “silent” middle feel (i.e., those who probably wouldn't respond to a survey) rather than just those who are extremely happy or unhappy. This creates a more complete picture of how customers feel about a product or service.
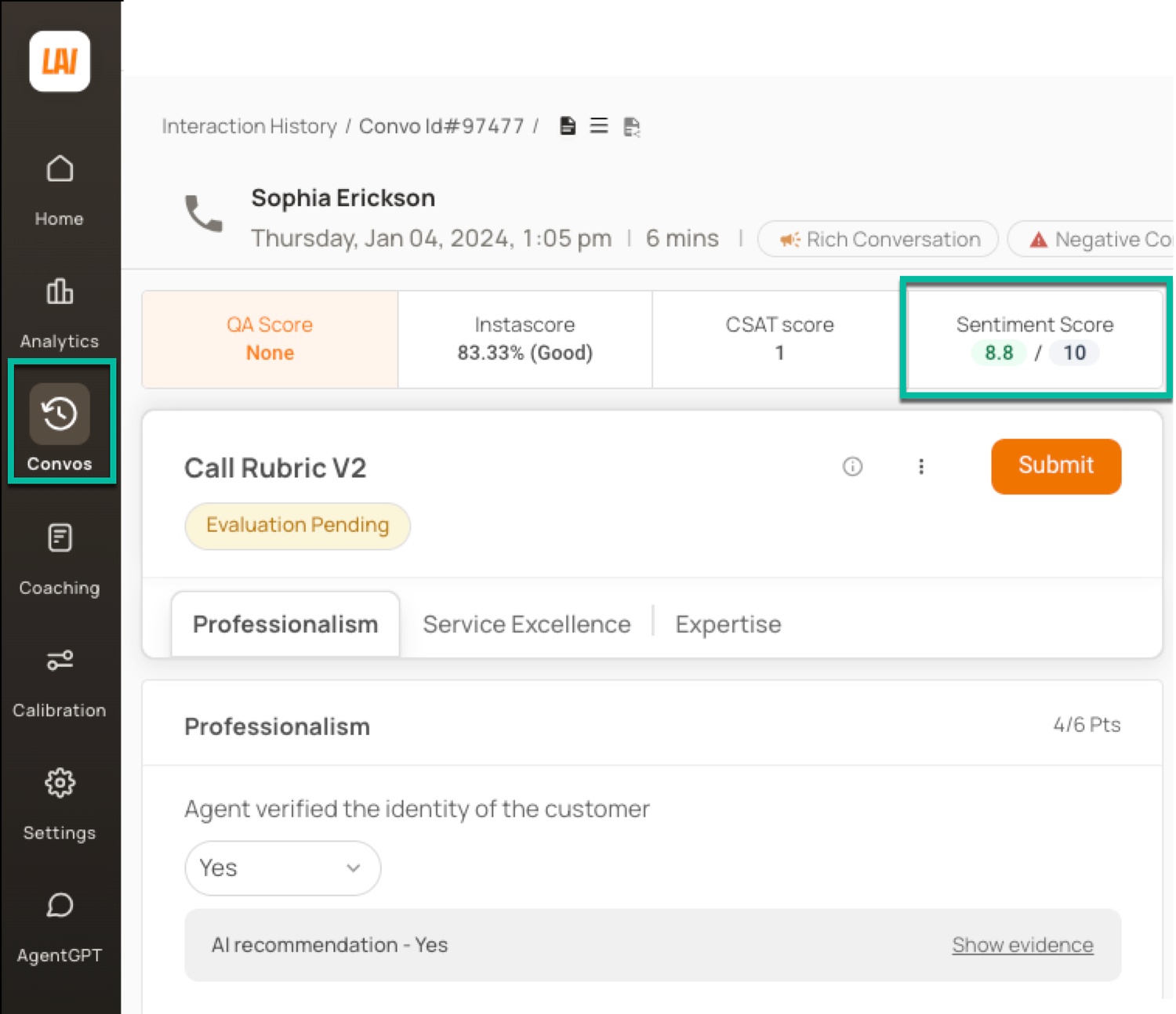
It's also important to track customer sentiment during live interactions to effectively guide agents on the spot while handling customer issues.
Level AI’s Real-Time Manager Assist provides a dashboard showing the Sentiment Score for ongoing calls, letting you gauge customer feelings during live conversations in your contact center. This gives you better guidance on whether to intervene or not (and gives you an early warning of potential churn) than simpler KPIs like call duration:

Additionally, it provides various features for intervening like Call Whisper and Call Barge to help agents during a call.
Other features of Real-Time Manager Assist allow you to gauge both the potential win or loss of helping a customer, as well as the current performance of the agent.
Find Coaching Opportunities for QA
Businesses measuring customer sentiment also typically follow call center quality assurance best practices such as listening to call recordings to find potential coaching opportunities for their agents.
Many of these are only able to randomly sample around 1–2% of calls with the assumption that the small sample size will be representative of all interactions. This narrow approach can overlook key patterns in agent behavior (both positive and negative), which may not accurately represent an agent's overall performance. This can lead to frustration for agents flagged for one-off mistakes that don't reflect their typical behavior.
Level AI evaluates 100% of conversations to deliver a complete view of recurring issues and provides fairer agent assessments than limited, random sampling.
With every conversation analyzed, our dashboards provide a complete overview and zero in on calls meriting further investigation using sentiment tags. These mark moments when sentiment shifts occur during interactions:

Since these tags are both searchable and filterable, you can create reports that zero in on occurrences of particular customer sentiment and observe how agents actually handled any negative sentiments and where improvements can be made.
Level AI also offers features beyond sentiment data to help managers analyze agent performance and find additional coachable moments for training:
InstaScore evaluates an agent’s performance during a customer conversation based on predefined rubrics, such as whether the agent:
- Used the customer’s name during the conversation
- Followed company procedure to resolve an issue
- Expressed empathy to the customer
- Offered a relevant solution to the issue
You can find InstaScore in the same All Interactions dashboard next to Sentiment Score. It’s displayed for every interaction as a percentage value, allowing managers to quickly see which conversations merit further evaluation:
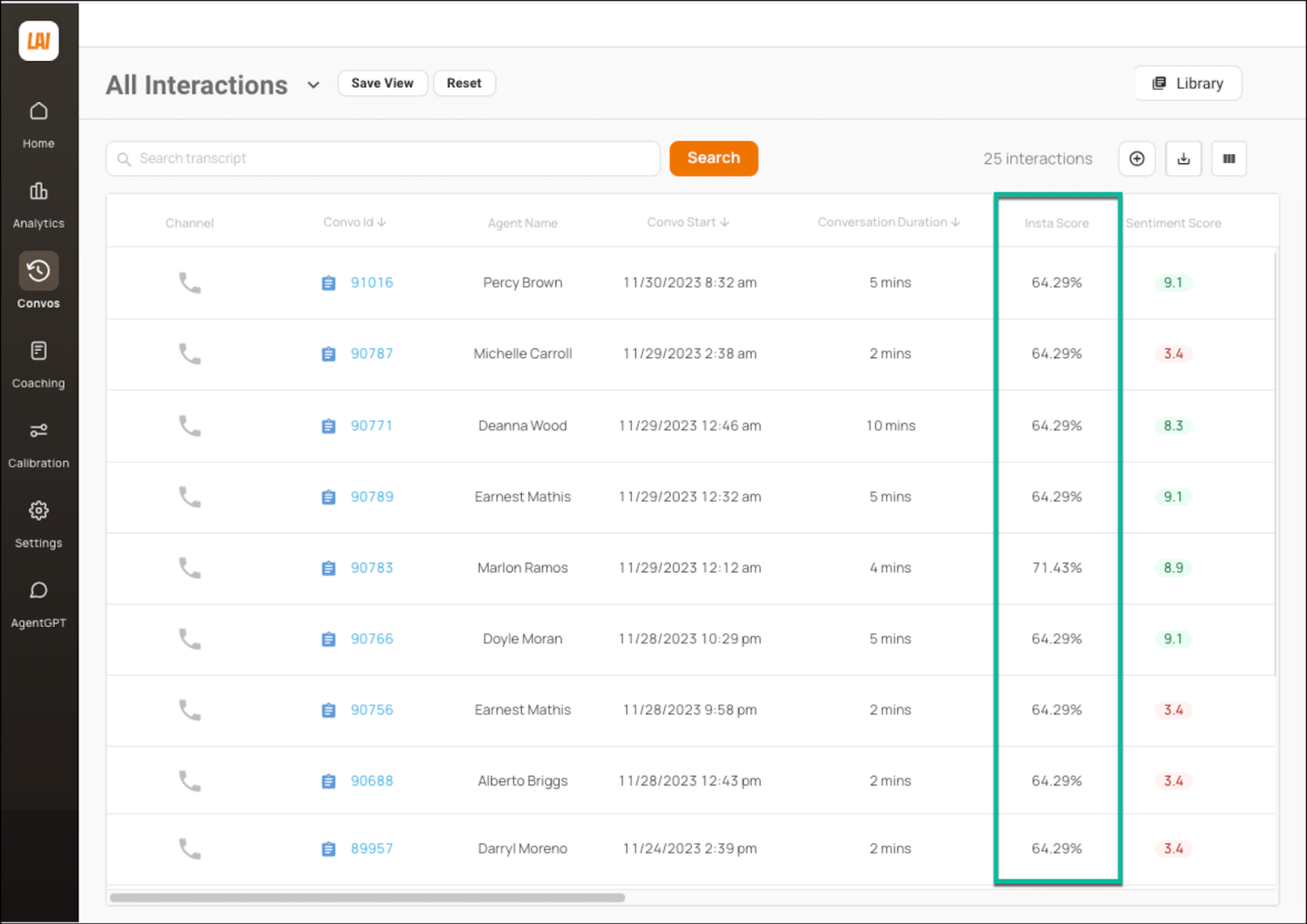
Another feature that can save managers and QA staff enormous amounts of time when reviewing interactions is InstaReview, which automatically tags conversations containing certain characteristics that require attention from managers.
Potential criteria for flags include:
- High number of requested assists
- Longer than average duration
- Low customer satisfaction
The system automatically tags conversations as rich, positive, or negative to indicate which calls are worth reviewing. You can locate these conversations and start a coaching session directly from the dashboard:
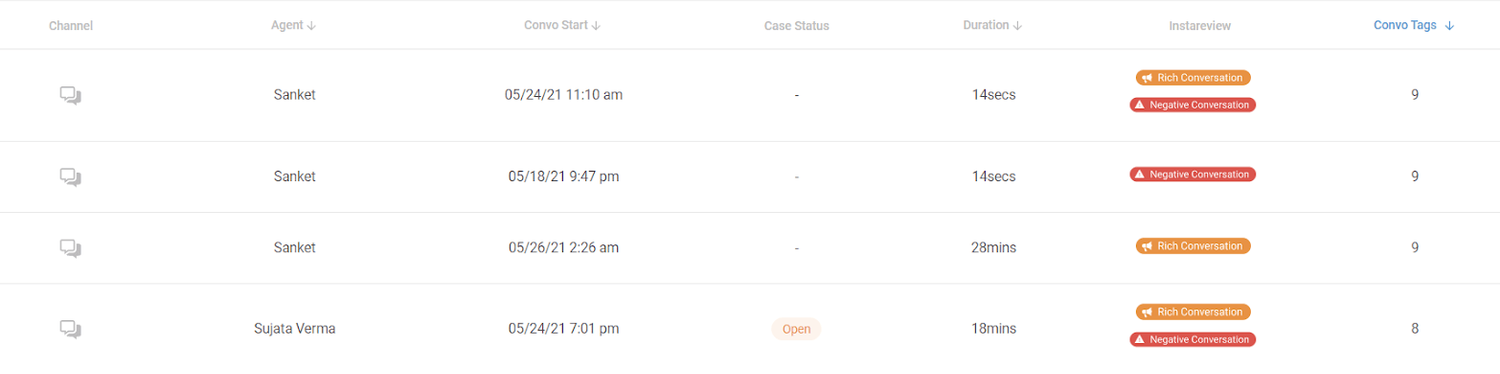
Identify Emerging Customer Sentiment Issues or Trends
As we've discussed earlier, traditional ways of gathering Voice of the Customer (VoC) data like using surveys often provide incomplete insights due to low response rates and inherent biases. That makes it harder for businesses to truly understand customer needs and feelings about their brand.
Level AI’s VoC Insights automatically collects and analyzes interaction data to provide a more complete and natural view of customer sentiment. Besides deriving standard metrics like CSAT, NPS, and CES, the system reveals both obvious and subtle patterns in customer feedback such as:
- Growing frustration with specific product features or user experience issues.
- Negative reactions to recent policy changes or price adjustments.
- Consistent confusion about billing processes or pricing structures.
- Increasing demand for self-service options or improved documentation.
VoC Insights displays such patterns in intuitive dashboards that track customer satisfaction and other trends over time. Users can also customize metrics, filter data, and drill down into specific areas of interest:
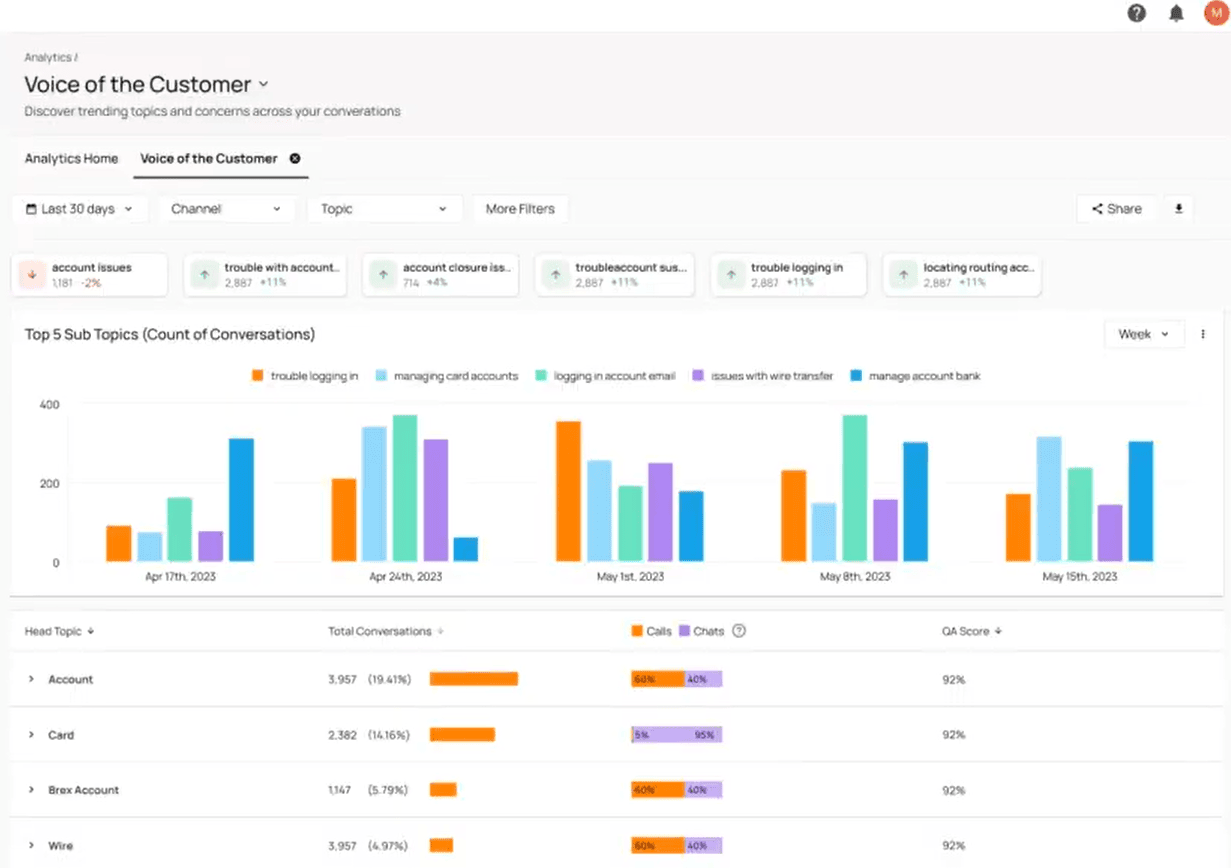
For instance, if customers frequently complain about the same account issues when logging in from a mobile device, our software reveals such a pattern in order for product teams to decide whether a change can be made that would improve the customer experience.
Read more about Medallia vs Qualtrics for enhancing customer experience.
Our VoC Insights go even further, providing a fuller picture of the customer experience with inferred customer satisfaction (iCSAT). While sentiment scoring provides valuable emotional context, this AI-powered scoring system combines Sentiment Score with two key components:
- Customer Effort Score: Measures how much work customers put into getting their issues resolved, including factors like repetition, hold times, and number of transfers.
- Resolution Score: Evaluates whether the customer's problem was successfully addressed by analyzing conversation outcomes and final interaction states.
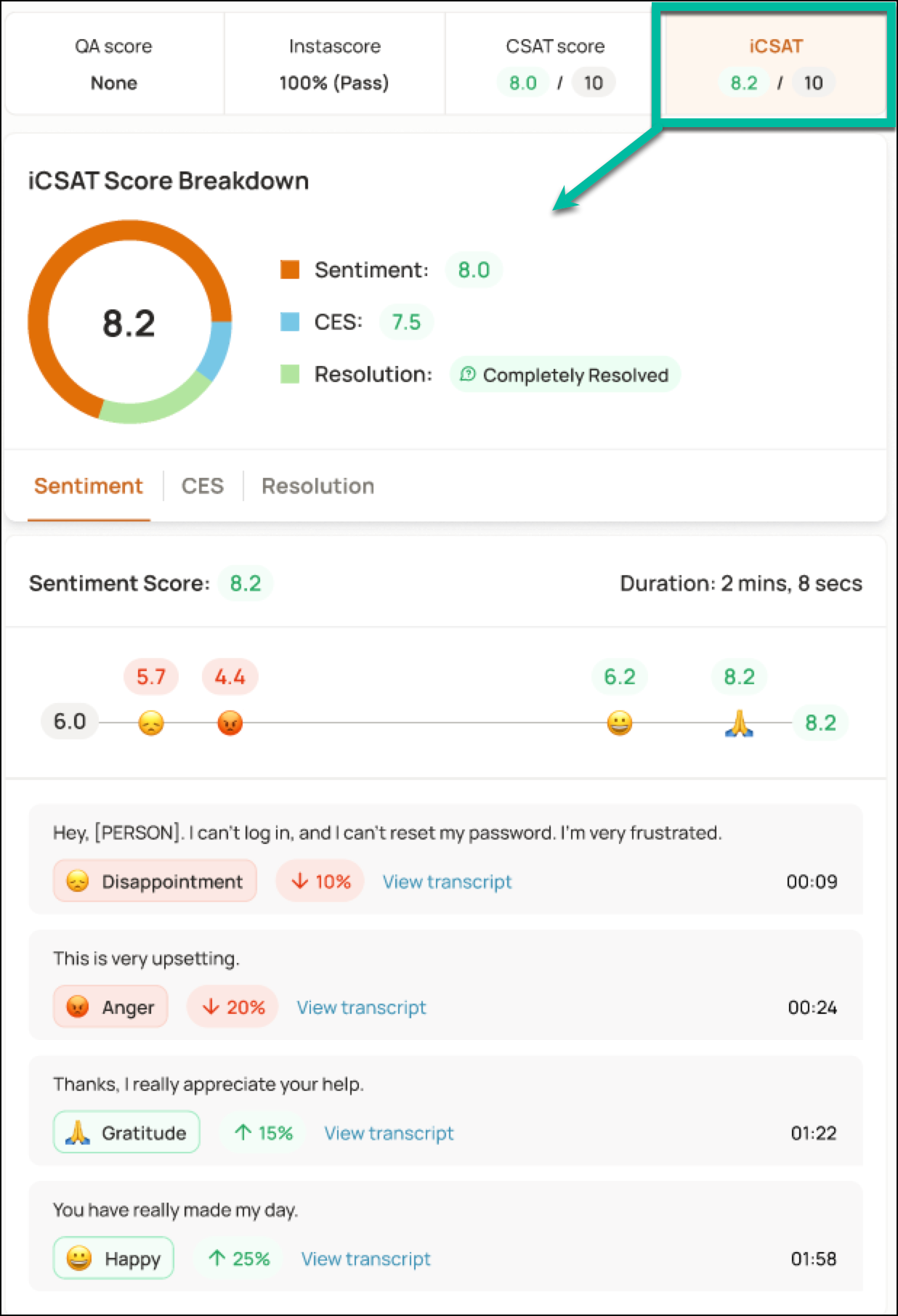
These three components are calculated together to create a score better reflecting customer satisfaction. Rather than relying on traditional CSAT surveys, iCSAT can help businesses automate the identification of satisfaction trends and opportunities that might otherwise go unnoticed.
Level AI also offers advanced reporting and analytics. Our Query Builder allows users to create customized reports that combine customer data from multiple sources, integrating internal platform data with external sources like CRM systems, knowledge bases, ticketing systems, survey tools, etc.
You can adjust your reporting by selecting specific metrics (e.g., number of flagged conversations), date ranges, and other customer filters:
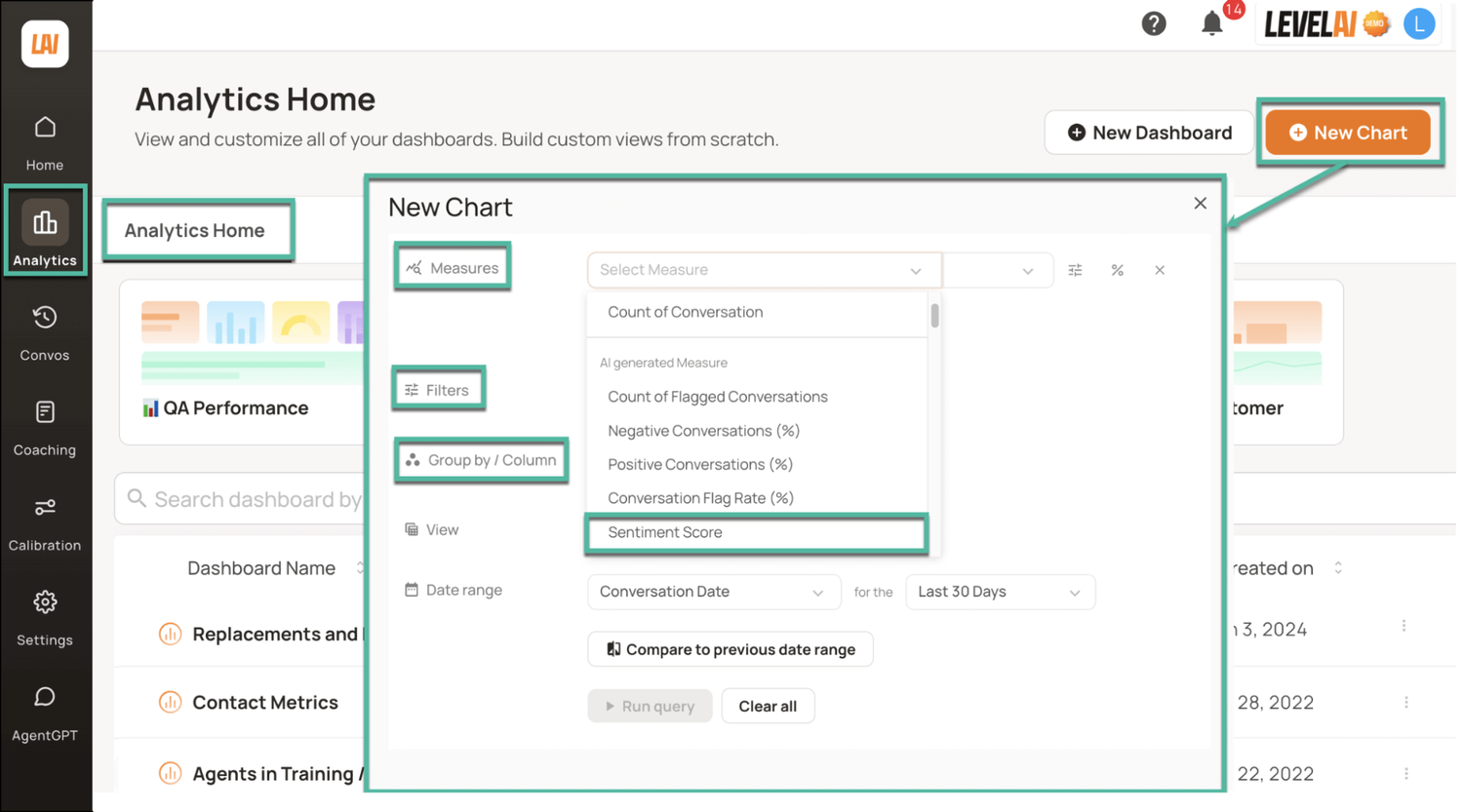
Consolidating all of your customer interaction data into a central call center analytics dashboard helps you uncover answers to critical questions, such as:
- Which topics regularly drive low customer satisfaction?
- What topics cause agents to transfer or escalate calls?
- How does customer sentiment predict the likelihood of positive feedback?
- Which agent responses most influence satisfaction metrics?
Answering such questions allows you to identify customer pain points and predict customer needs in order to improve customer experience.
Read more about Verint competitors and the best CX platforms.
Improve Customer Satisfaction with Advanced Sentiment Analysis
Level AI reveals how your customers truly feel with near-human accuracy, giving companies the tools they need to quickly analyze and act on customer feedback.
Ready to implement customer sentiment analysis and use cases in your organization? Book a demo with Level AI to learn about our platform’s secure and customizable Generative AI capabilities, and how we can help improve your customer satisfaction and business performance.
Keep reading
View all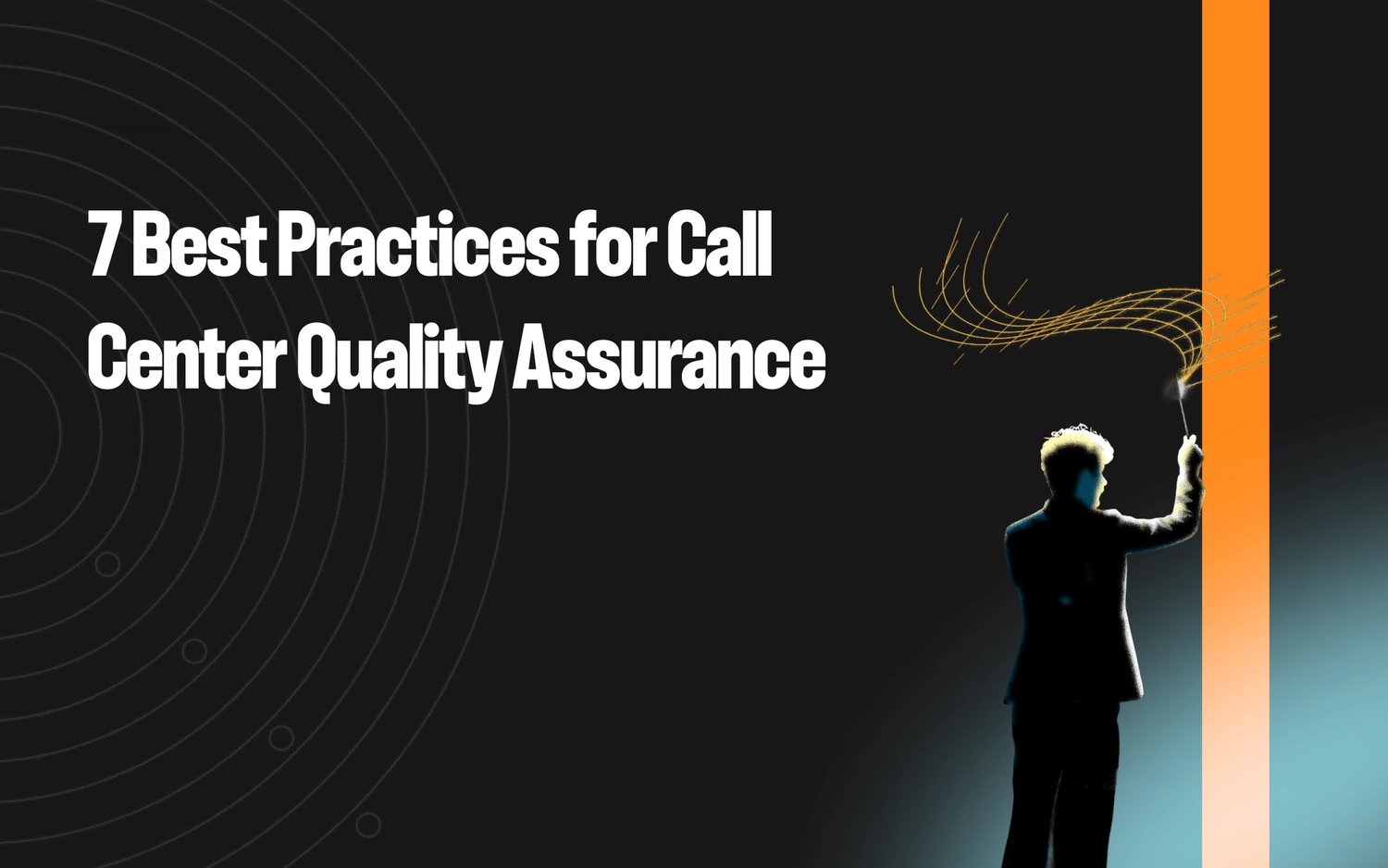
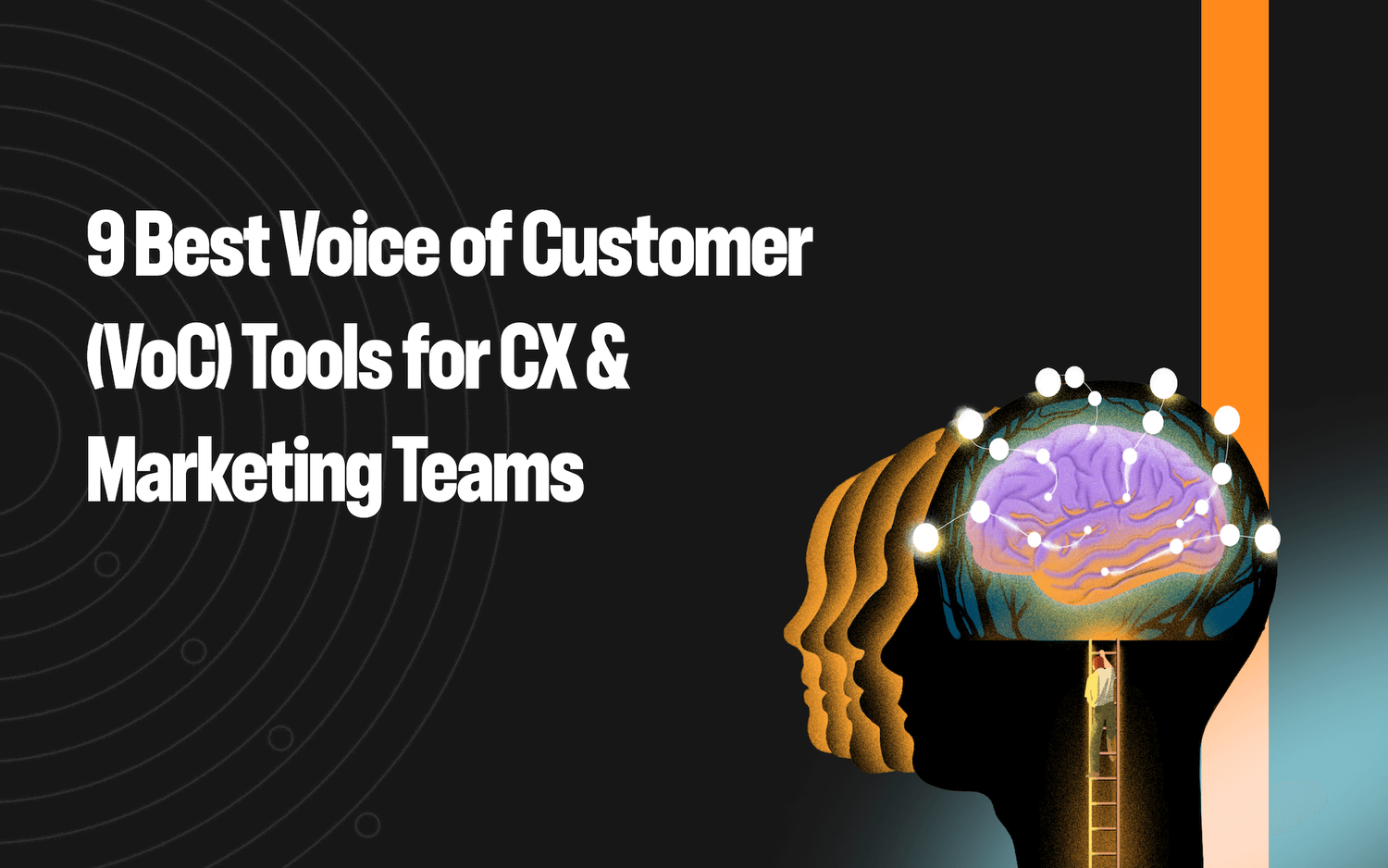
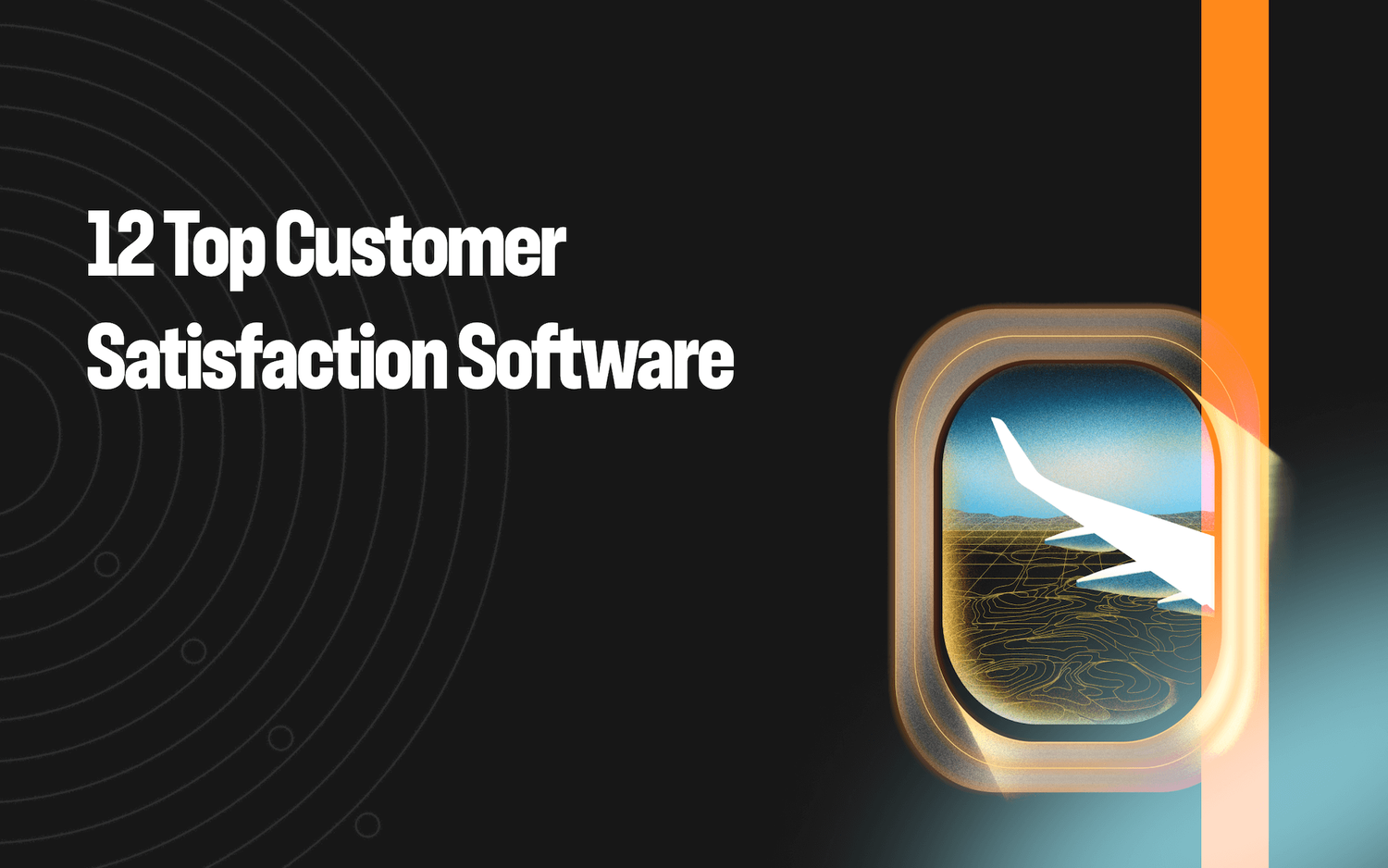