8 Best Practices for Monitoring Call Center Performance in 2025

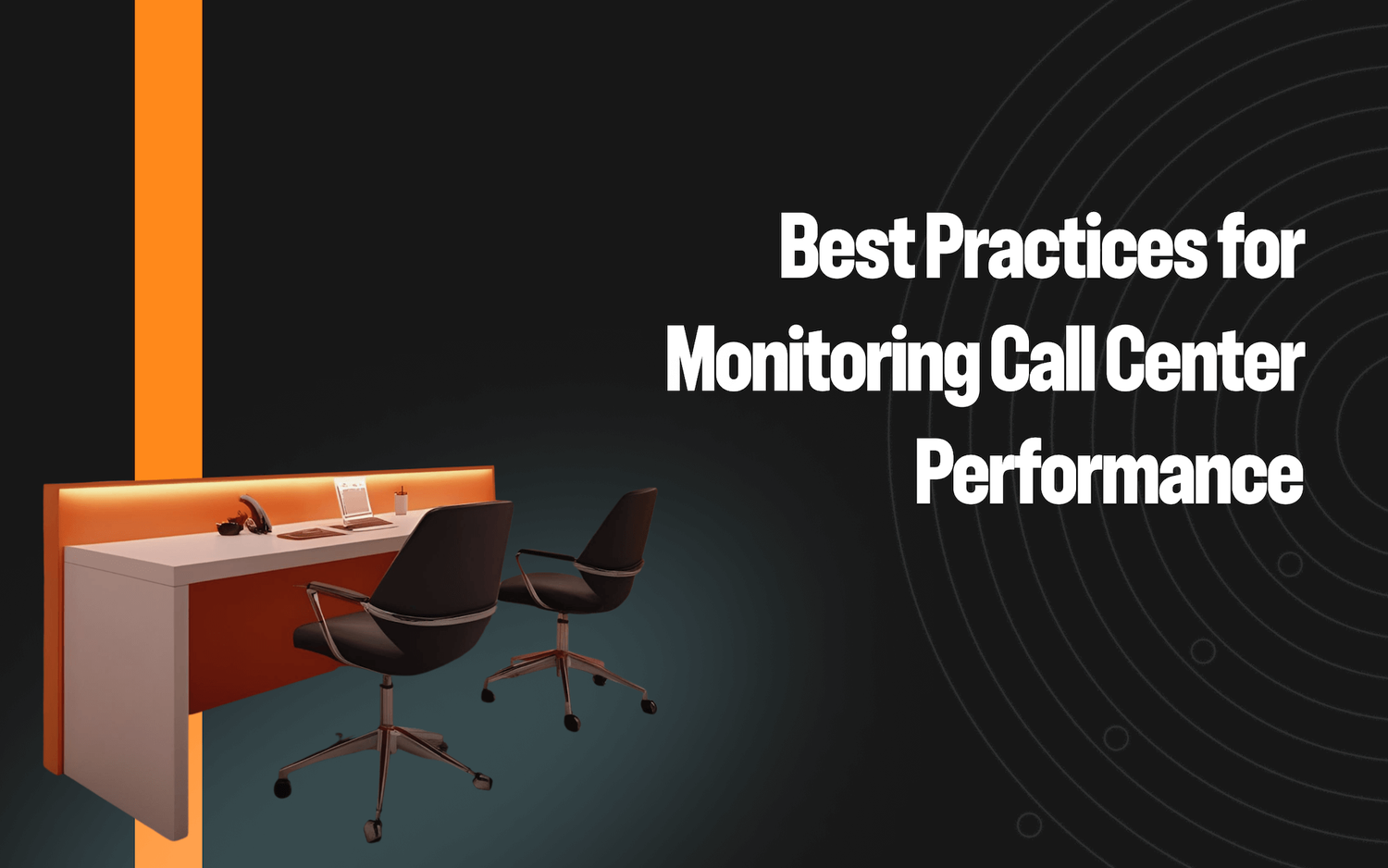
Most modern call centers track performance using key metrics focused on efficiency, like average handle time (AHT), first call resolution (FCR), call volume, and abandoned call rate.
Many take it a step further by including voice of the customer (VoC) metrics such as customer satisfaction (CSAT), net promoter score (NPS), and post-call surveys. These give teams a clearer view of how customers feel about their experience instead of just how quickly issues are resolved.
However, the most advanced contact centers now use AI-powered tools to analyze every single customer-agent interaction, including parsing conversations in real time. This unlocks a level of understanding on what customers are saying, feeling, and why that was previously difficult or impossible to obtain through legacy tools.
They can detect emotions, tone shifts, and reasons for the call — spotting frustration, satisfaction, or signs that a customer might leave. This allows agents and supervisors to respond faster and more effectively.
In this article, we break down 8 proven best practices used by high-performing call centers to monitor and improve performance, along with real-world examples from our call center monitoring platform.
Table of Contents
- Focus on customer-centric metrics
- Track performance at every level of your call center
- Combine quantitative metrics with qualitative feedback
- Analyze customer sentiment to uncover service gaps
- Unify reporting across silos
- Implement real-time call monitoring to instantly surface issues
- Continuously gather customer feedback
- Use AI to pinpoint coaching opportunities
1. Focus on Customer-Centric KPIs
Every call center performance monitoring program should start with tracking key metrics, which are usually gathered from post-interaction surveys:
Customer Satisfaction Score (CSAT) | Measures customers’ satisfaction with a product, service, or call center experience. CSAT uses a 1–5 scale (very dissatisfied to very satisfied) and gives teams a quick snapshot of each interaction with the option to collect follow-up feedback. |
---|---|
Customer Effort Score (CES) | Measures how hard it was for customers to resolve their issue on a call. Just like CSAT, it’s measured on a scale from 1 to 5, from “Very hard” to “Very easy.” |
Net Promoter Score (NPS) | Measures (on a scale from 1 to 10) how willing customers are to recommend a company to others, and also helps to understand customers’ overall experience and satisfaction with a company. |
First Call Resolution (FCR) Rate | Also known as first contact resolution rate, this tracks how many calls have been resolved during the first call without customers having to follow up or wait for a call-back. |
Average Call Abandonment Rate | The percentage of customers who hang up before reaching an agent. It’s a key call center performance metric that strongly affects customer satisfaction and NPS — especially when wait times are long. |
Total Resolution Time | Shows how long, on average, it takes agents to solve a customer issue. |
Average Wait Time | Also known as average hold time, this tracks the average amount of time customers spend on hold throughout the conversation. |
First Response Time (FRT) | Measures how long it takes for customers to be connected to an agent. |
Active Waiting Calls | Tracks the number of calls on hold while agents speak with other customers. |
Average Handle Time (AHT) | Measures the length of time it takes to resolve a customer query, including customer wait time and agent after-call work. |
Agent Utilization Rate | Tracks agent productivity: how much time agents spend handling calls out of their total work time. |
After-Call Work Time (ACW) | Measures how long it takes agents to complete call-related tasks before moving on to the next call. |
Even though tracking agent and call center efficiency metrics is important, you’ll want to prioritize customer satisfaction metrics (e.g., CSAT, CET, NPS). That way, you can avoid situations where customer experience suffers from over-optimizing for efficiency.
For example, agents closing tickets after simply responding to a query instead of resolving it would improve a “resolution rate”-related stat, but at the expense of customer experience.
2. Track Performance at Every Level of Your Call Center
In our experience, the most effective call centers don’t just track metrics across the entire call center; they monitor these metrics at every level: organizational, team, and individual.
Some examples of monitoring at the organizational level include:
- Tracking progress on stated goals. For example, if one of your goals is to improve customer satisfaction overall, then tracking CSAT scores will show if your call center performance is actually making progress against that goal. This avoids a situation where an organization declares a goal but doesn’t have an objective metric to track if progress is actually being made.
- Monitoring call center performance against business objectives. If your organization focuses on increasing customer loyalty, you’ll need to track NPS and customer feedback closely during their interactions with call center agents to see what can be done to lift NPS and reduce churn.
- Analyze customer call trends to find improvement opportunities across teams. Call center teams are often the first to notice how product updates, supply chain changes, and policy changes affect customers. They can help other teams understand the impact of those changes and improve customer satisfaction overall. For example, call center teams can add updated product information to a knowledge base or expand FAQ sections to specific website pages.
At the team level, you can uncover opportunities to improve your agents’ performance:
- Monitor common issues that agents struggle with to develop group training programs.
- Identify high-performing agents and analyze their performance.Then you can use that data to update your call center quality assurance best practices and implement them across the whole team.
- Finally, compare how different agents perform against each other to uncover systemic issues that affect everyone’s performance. For example, you may realize that your onboarding training contains out-of-date materials and needs to be updated.
On an individual level, it’s worth evaluating each agent by using both standardized rubrics and performance benchmarks to get a full picture of their effectiveness. This helps identify areas where agents need to improve and track their progress (we’ll cover best practices for evaluating agents below).
3. Combine Quantitative Metrics with Qualitative Feedback
A risk that teams face is focusing exclusively on improving metrics without understanding their impact on customer experience.
For example, trying to decrease AHT by encouraging agents to resolve calls faster can lead to agents rushing through calls, which may lower customer satisfaction and, ultimately, retention.
This is why it’s important to balance different sources of performance data:
- Metrics and KPIs as benchmarks and early warning indicators.
- Customer feedback to understand what can be done to improve customer experience and recognize what’s already working well.
- Ongoing call reviews to uncover quality issues and training opportunities for agents.
While tracking and monitoring metrics is relatively straightforward, qualitative data can feel daunting to handle, especially if you don’t have tools that automate its analysis.
The most common approach is to develop rubrics that help QA teams evaluate agent performance quickly and consistently by scoring their ability to follow company policies, such as:
- Greeting and addressing customers according to the company script
- Closing the conversation for unresolved issues by outlining next steps
- Documenting customer interactions according to company protocols
Rubrics can also measure agents’ ability to use soft skills like active listening or showing empathy.
To gather qualitative data from conversations (like adherence to rubrics), QA teams must usually listen to randomly selected call recordings to get a representative sample of how agents are doing. It’s a time-consuming activity that usually means getting through only 1–2% of the total call volume.
Although the samples can be considered representative, this doesn't fully capture how all agents perform across the entire call center.
The most advanced contact centers use AI-powered solutions like Level AI to achieve full coverage of customer interactions — automatically scoring every call and chat for rubric adherence, soft skills, and service quality. This gives teams a complete view of agent performance without the need for manual review.
For example, Level AI’s InstaScore uses AI speech analytics to automate quality monitoring across all phone calls and consistently auto-scores agent performance against your defined rubrics (we talk more about this capability below).
4. Analyze Customer Sentiment to Uncover Service Gaps
Without sentiment data, businesses can only see what was said, not how it was said.
Relying solely on written customer feedback or CSAT scores doesn’t capture the more subtle emotional signals in a customer’s tone of voice, such as frustration, urgency, or disappointment.
These emotional cues are often missed in text but play a key role in understanding the true customer experience.
Advanced call centers use call center voice analytics to capture customer sentiment, which helps QA teams quickly spot calls where customers express frustration or dissatisfaction. It also reveals patterns, like specific words or phrases that tend to appear in negative interactions.
(Read our latest article on AI use cases in the contact center.)
Another key reason to track sentiment throughout a call is that drops in customer satisfaction often appear as subtle tone shifts before complaints start to rise. For example, a customer’s voice may gradually sound more frustrated as the call goes on.
By monitoring these changes in real time, teams can take action earlier and help prevent escalations.
Level AI tracks changes in customer sentiment in real time and recognizes the highest number of emotions of any product in its category (i.e., anger, annoyance, disapproval, disappointment, worry, happiness, admiration, and gratitude).
This data is then used in our live call dashboards and real-time alerts for managers (we’ll talk about those features later below) and to generate iCSAT (inferred CSAT), which is a composite score that gives a holistic view of customer satisfaction.
iCSAT is calculated from three separate scores to give you a more accurate and nuanced understanding of customer satisfaction:
- Resolution score is based on whether a customer's issue was completely or partially resolved.
- Sentiment Score evaluates a customer’s overall sentiment throughout the call.
- Customer effort score assesses the amount of effort customers put into resolving their issue, such as entering account details multiple times or going through a complex self-service flow before being able to talk to an agent.
Overall, iCSAT is measured on a scale from 1 to 5, where 1 is very dissatisfied and 5 is very satisfied:
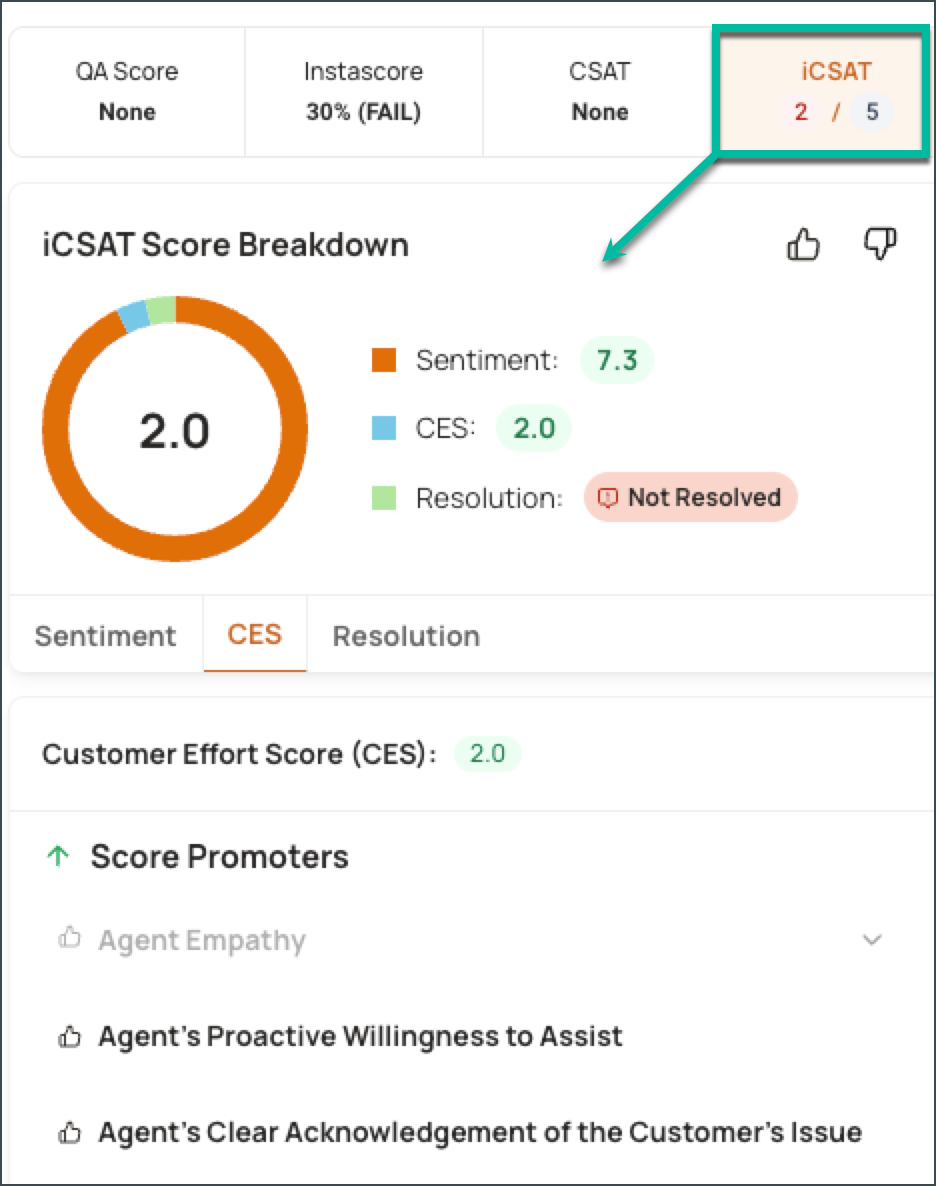
Call center managers and QA teams can use iCSAT to uncover unmet customer needs, pinpoint the root causes of frustration, and identify common call situations that impact satisfaction — without manually searching through and reviewing call recordings.
For example, if customers express high negative sentiments after spending a long time on hold, you can search for solutions to decrease AHT. If they get frustrated with script-driven responses, you can update the scripts to address that. And, if customers become angry during agent escalations, you may update their training to address the issue (more on that below).
5. Unify Reporting Across Silos
Dealing with fragmented data is one of the biggest challenges in call center reporting. Information might be scattered across spreadsheets, locked inside CRMs or help desk tools, or managed by third-party teams.
This fragmentation creates an incomplete view of the customer experience, making it harder to identify root causes, track sentiment, or spot service gaps. It can also lead to conflicting metrics when different systems report KPIs using inconsistent definitions or timeframes.
To get an accurate picture of how your call center is really performing, reporting should be based on unified data sources. It’s easier to uncover trends, connect the dots, and confidently make decisions when data is centralized.
Many contact centers are now solving fragmented data using technology to consolidate information from multiple systems into a single source of truth. With integrated platforms, teams no longer need to juggle dashboards or manually pull reports. They get a clear, consistent view of performance in real time.
Level AI’s Query Builder allows you to create reports from both its own data (e.g., iCSAT scores, agent performance data, and NPS results), as well as data from external sources:
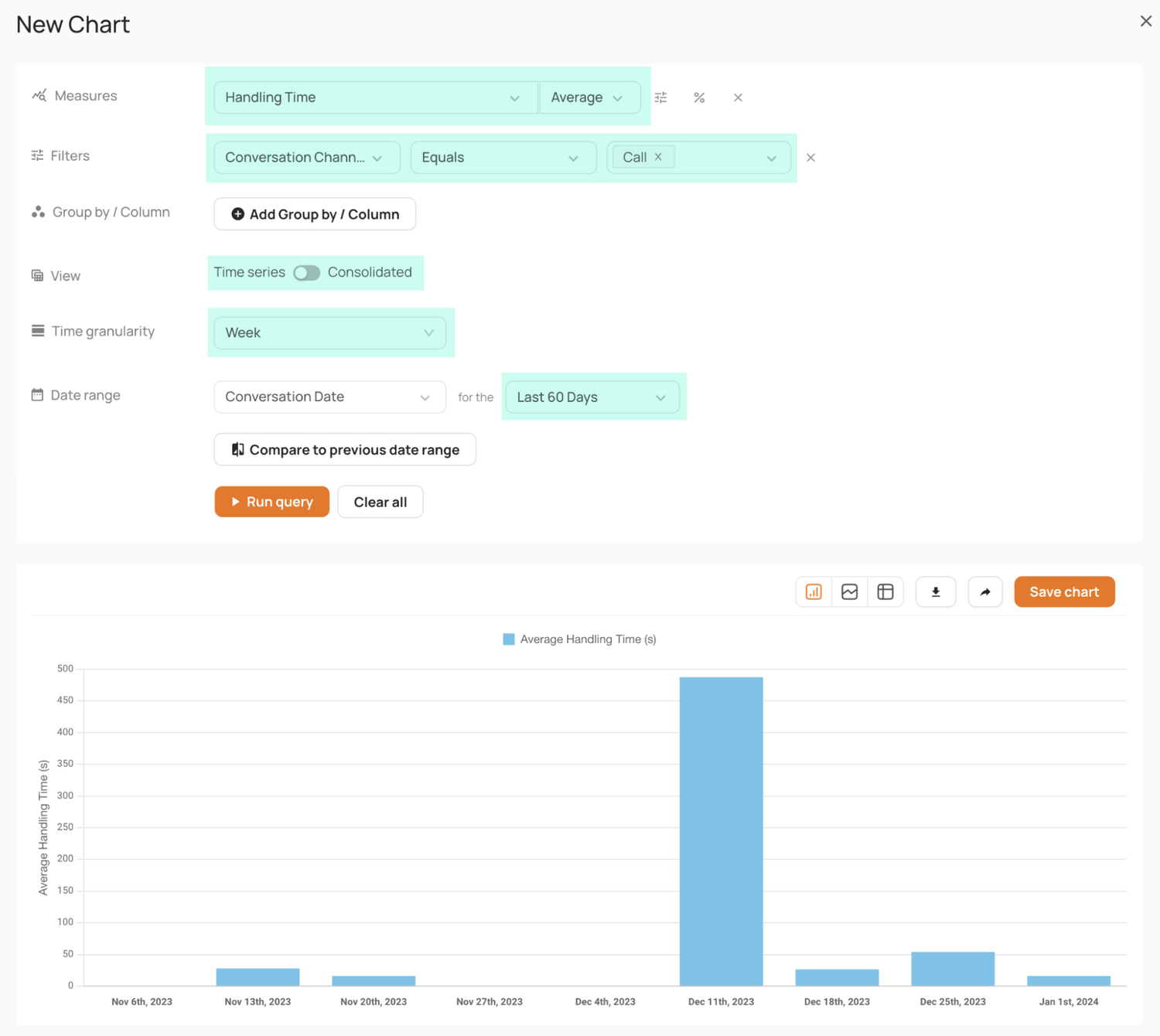
You can combine data in custom dashboards to answer questions like:
- Did our new customer scripts lead to higher CSAT scores?
- Which strategies are generally effective for reversing negative customer sentiment during calls?
- Are there any patterns in customer interactions that consistently happen before customers churn or cancel their accounts?
- Are there any specific types of issues (for example, installing software or using a particular product model) that consistently result in repeat calls?
You can share each call center analytics dashboard with other teams across your organization.
Level AI also provides unified reporting through a growing list of integrations with major CRM, LMS, workforce management and communications applications and systems.
6. Implement Real-Time Call Monitoring to Quickly Identify Issues
Traditionally, call center managers rely on operational metrics to identify calls where they may need to intervene, such as call duration higher than AHT, excessively long hold times, or high frequencies of holds on a call.
However, these metrics aren’t always a reliable indicator of escalation, because they don’t take into account customer sentiment or customer intent.
Because of that, managers may end up intervening in calls where their help isn’t needed and miss the calls where agents could have used extra support.
For example, sometimes calls run longer because customers have a lot of questions or need extra help when going through a specific set of instructions. Or, customers get frustrated and hang up instead of staying on the call to let agents resolve their issue in a more satisfactory way.
Tech-enabled call centers use AI for customer sentiment analysis in real time to measure how customers are feeling, track how their sentiment changes throughout a call, and analyze their intent to gauge more accurately whether or not it's worthwhile to intervene.
Level AI’s Real-Time Manager Assist provides an overview of all conversations in progress along with key performance indicators like call Sentiment Score, InstaScore (agent performance), and actionable insights to let you know at a glance how the call is going:

You can dig deeper into call stats by clicking on the relevant KPI:
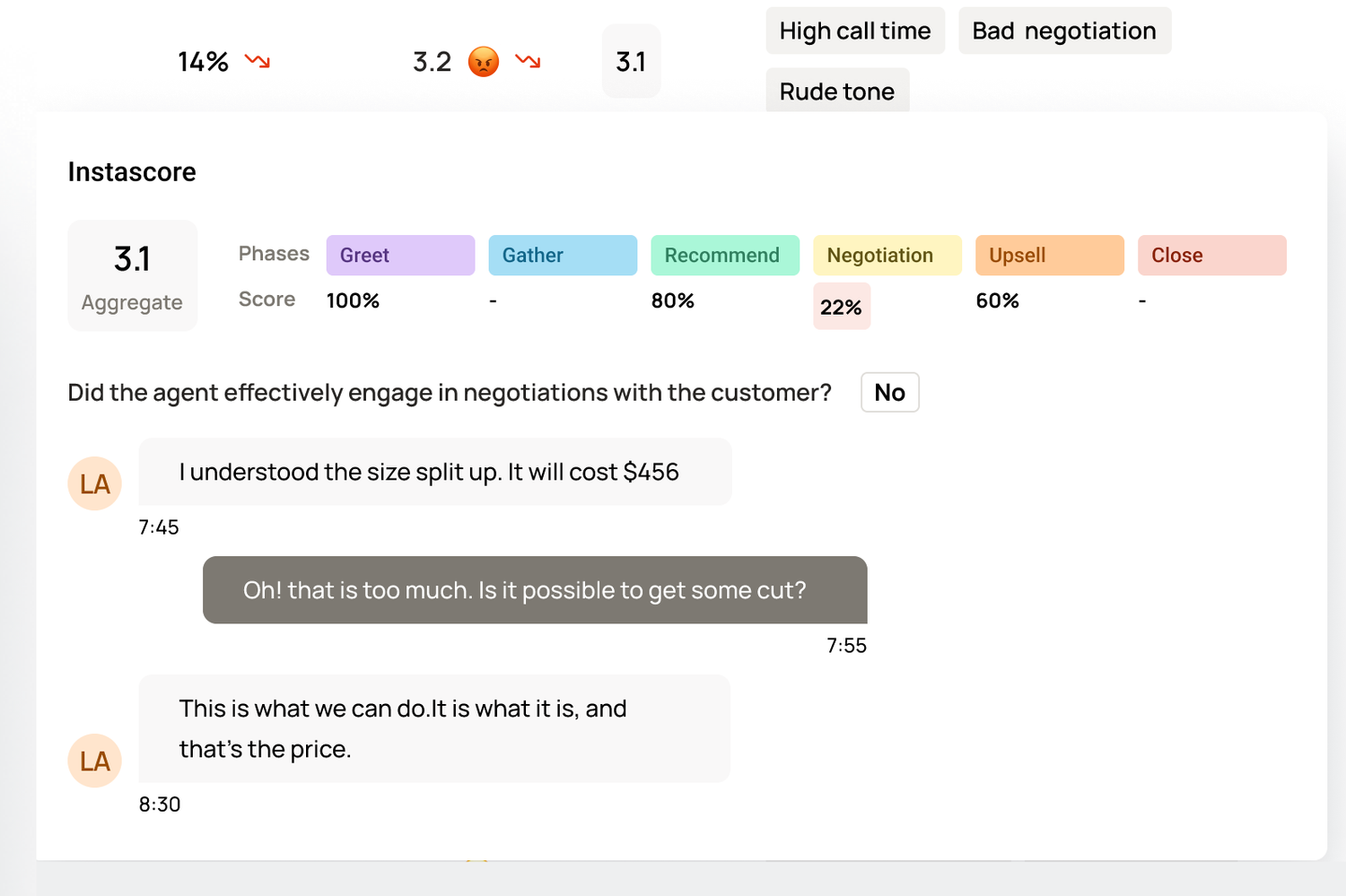
After reviewing those details, you can choose call whispering or call barging to help agents resolve issues quickly and effectively.
You can also set up the system to send alerts when certain thresholds are met (like if a customer is about to churn) through Slack or Microsoft Teams.

7. Continuously Gather Customer Feedback
Many call centers collect feedback through post-call surveys, but these tend to have low response rates. They also attract responses from customers who had either very positive or very negative experiences, excluding the silent majority of moderately satisfied or neutral customers.
(Read our latest article on top SurveyMonkey alternatives.)
This incomplete feedback limits the organization’s ability to assess overall sentiment accurately, identify emerging issues, and make informed decisions to improve service quality and customer experience at scale.
In contrast, modern call centers use AI tools for customer service powered by natural language understanding and semantic intelligence to analyze every single call, chat, or email. These tools look not just at the words being said, but also how they’re being expressed.
This allows them to figure out both the intent behind a customer’s message (what they want) and the emotion behind it (how they feel). By doing this across all interactions, these systems can spot patterns that might go unnoticed with traditional methods, like a sudden rise in complaints about a certain product, or common behaviors shared by top-performing agents.
This, in turn, helps call centers improve training, fix recurring problems faster, and create a better experience for both their teams and their customers.
Level AI's Voice of the Customer (VoC) Insights analyzes conversations to detect patterns, automatically displaying key metrics like CSAT and NPS, along with emerging trends and hidden anomalies that might otherwise go unnoticed.
These trends and stats are presented in intuitive dashboards that can be shared across your organization:
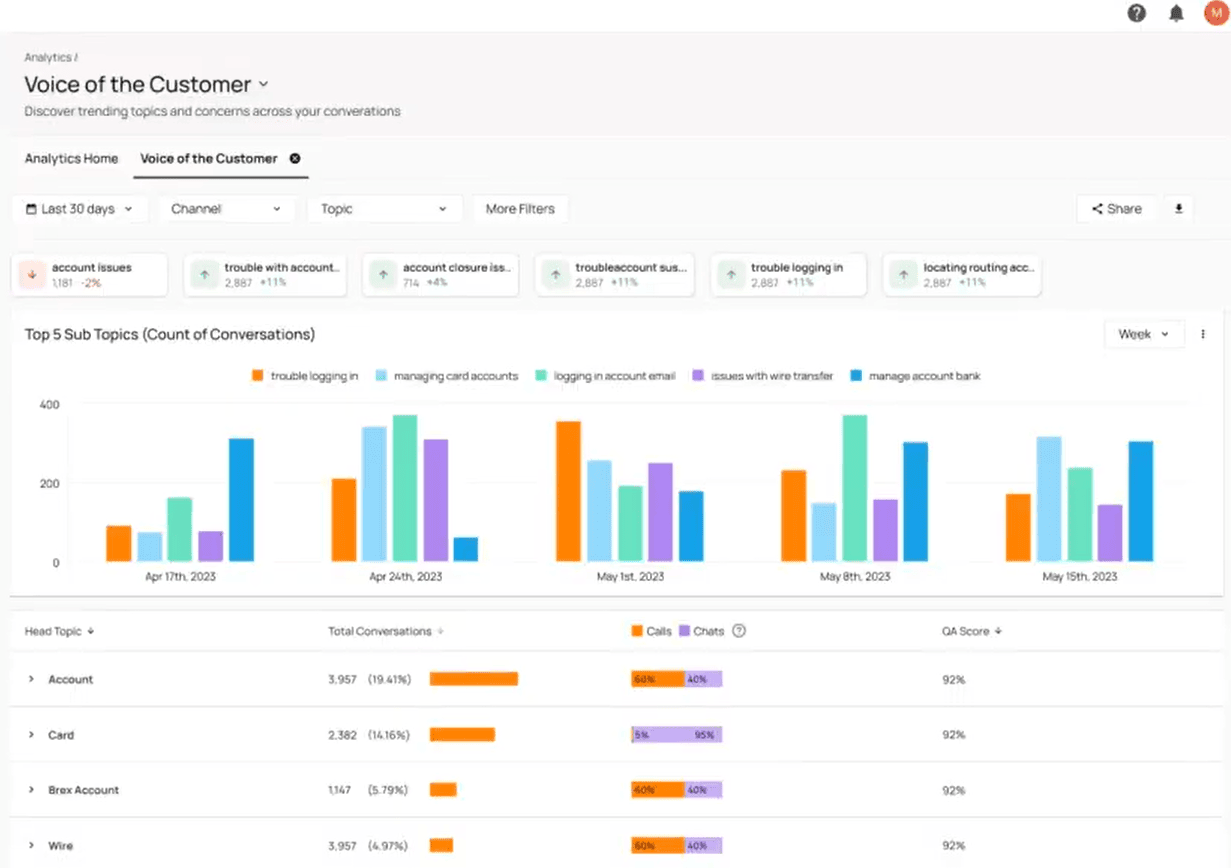
Using Level AI’s VoC Insights, a leading financial institution saved over $3 million after identifying their customers’ desire for more autonomy, which led them to design a self-service solution.
8. Use AI to Pinpoint Coaching Opportunities
Accurately and consistently identifying where agents struggle requires tracking agent performance at scale across all customer calls in your organization.
Doing so will reliably pinpoint specific coaching opportunities, recurring knowledge gaps, and process breakdowns that may be holding back both individual agent success and overall contact center performance.
Level AI’s InstaScore analyzes all interactions to measure every agent’s performance against your organization’s rubrics, such as how they responded to customer concerns, if they followed issue resolution policies, or whether they were courteous.
This score is expressed as a percentage and appears next to every conversation in our QA dashboards, providing an instant snapshot of how well each agent is meeting quality standards. InstaScore also makes it easy to identify who needs support and where to focus coaching efforts.
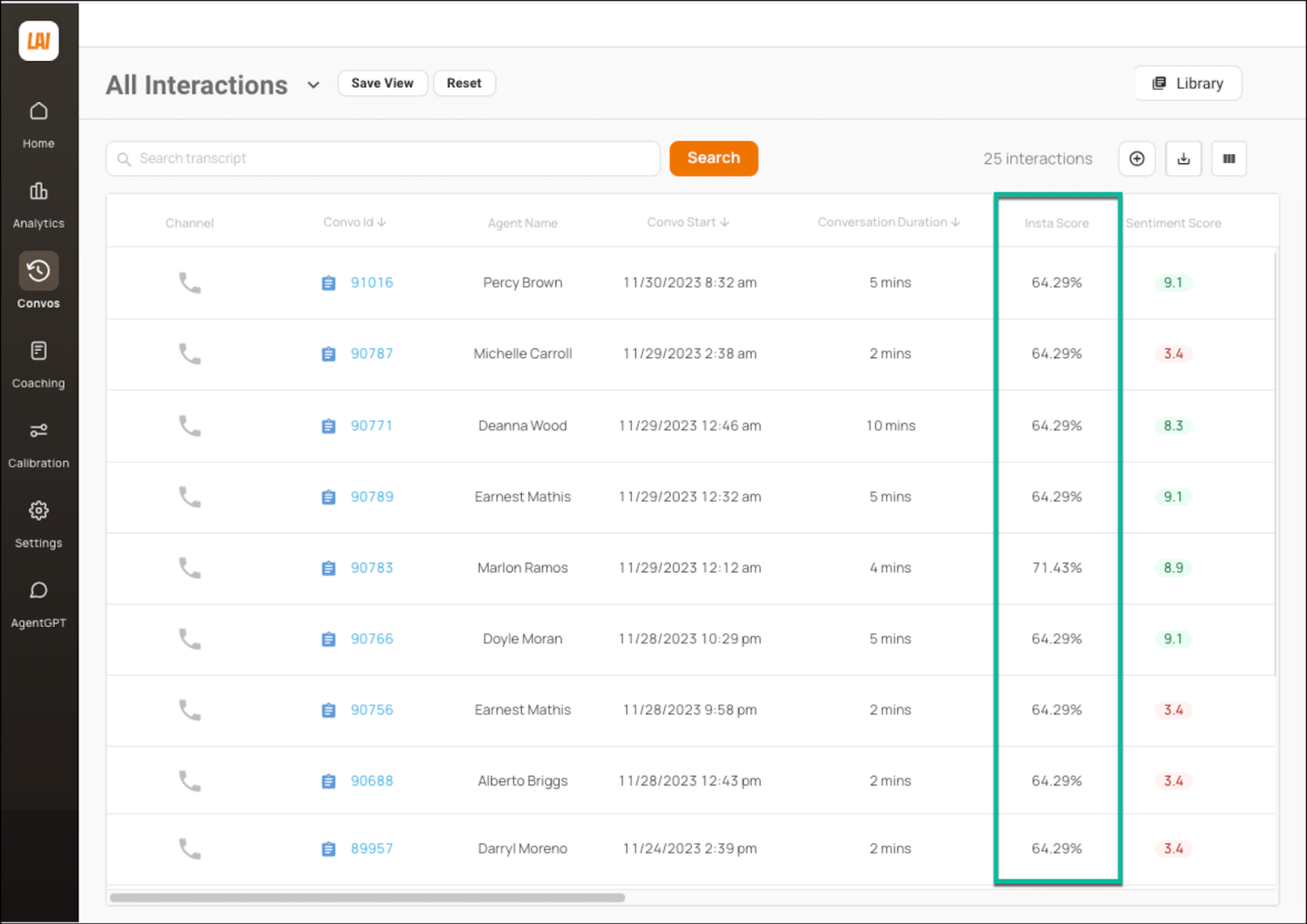
One of Level AI’s customers, a multinational design and marketing firm, increased their overall QA score from 60% to 88% after deploying their rubric and targeted action plans based on their agents’ performance.
As a result of targeted agent training and improved QA, the company saw an increase in CSAT scores and a reduction in unwarranted refunds, saving over $30 million.
Establish Your Advanced Call Center Monitoring Program
Depending on your call center size, simply monitoring KPIs, manually checking agents’ calls for compliance, and providing training based on those reviews may be enough to improve your call center performance.
But if you need a more comprehensive approach that helps streamline call center operations, track agent performance in real time, and provide in-depth speech analytics, schedule a demo with Level AI.
Keep reading
View all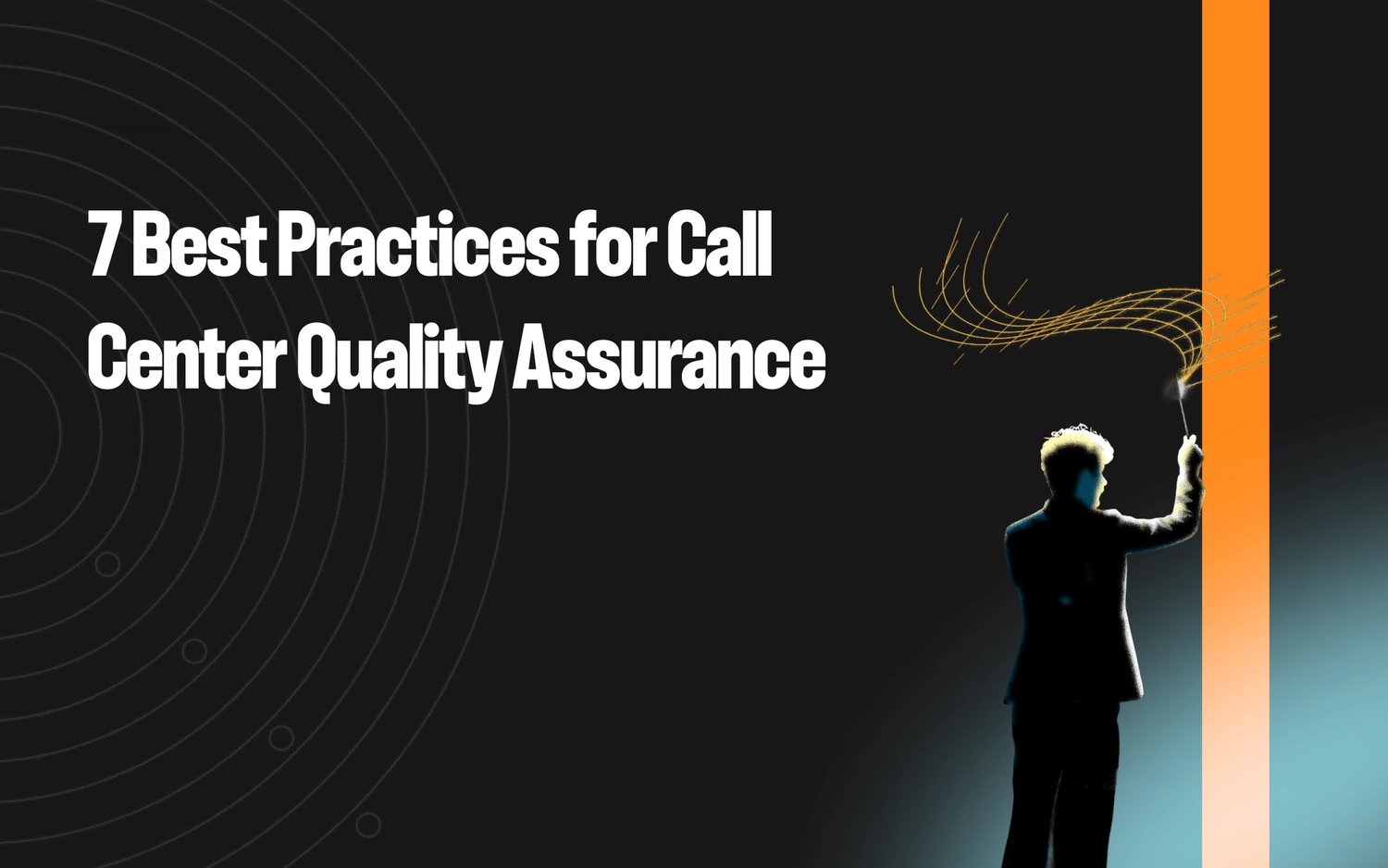
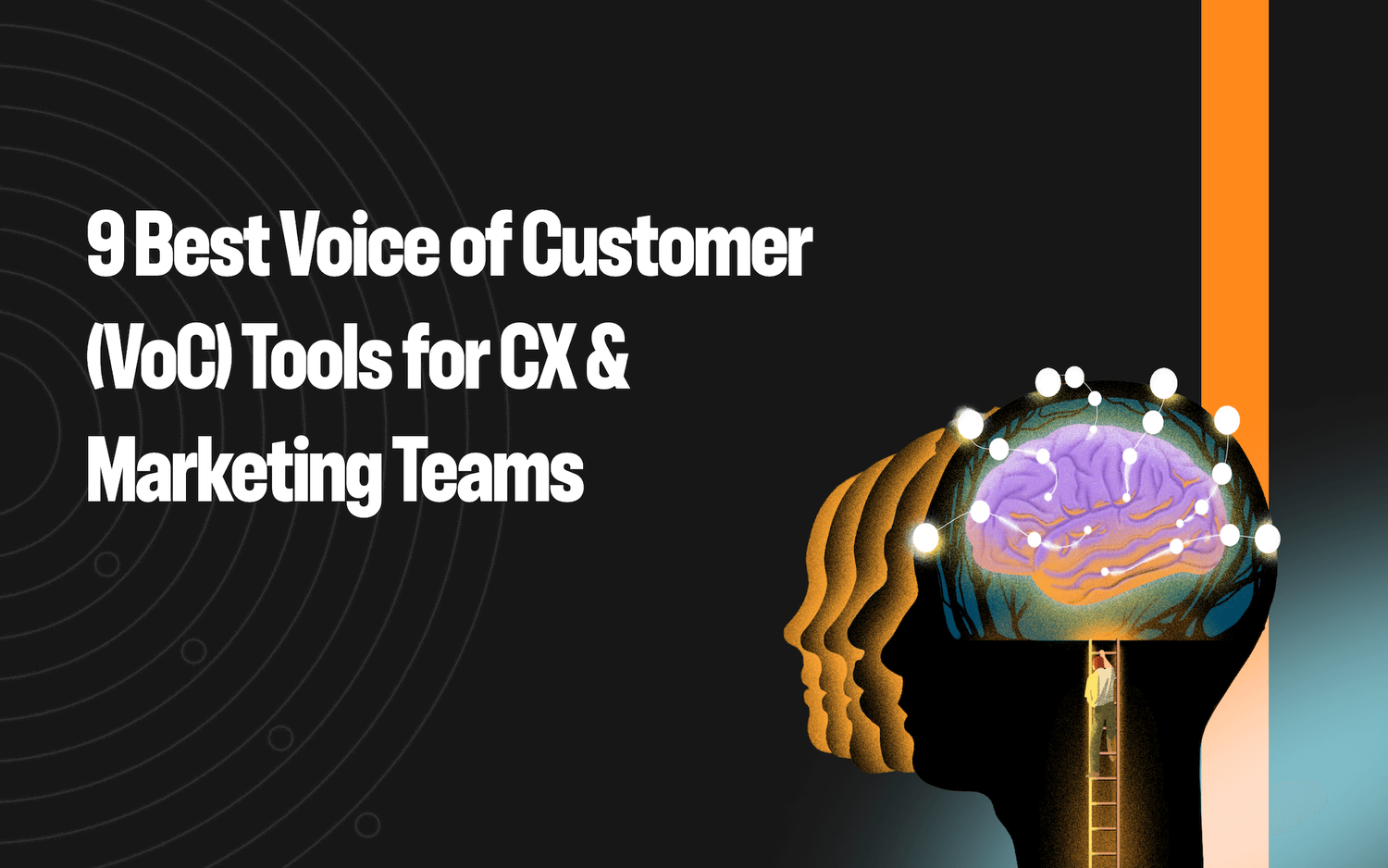
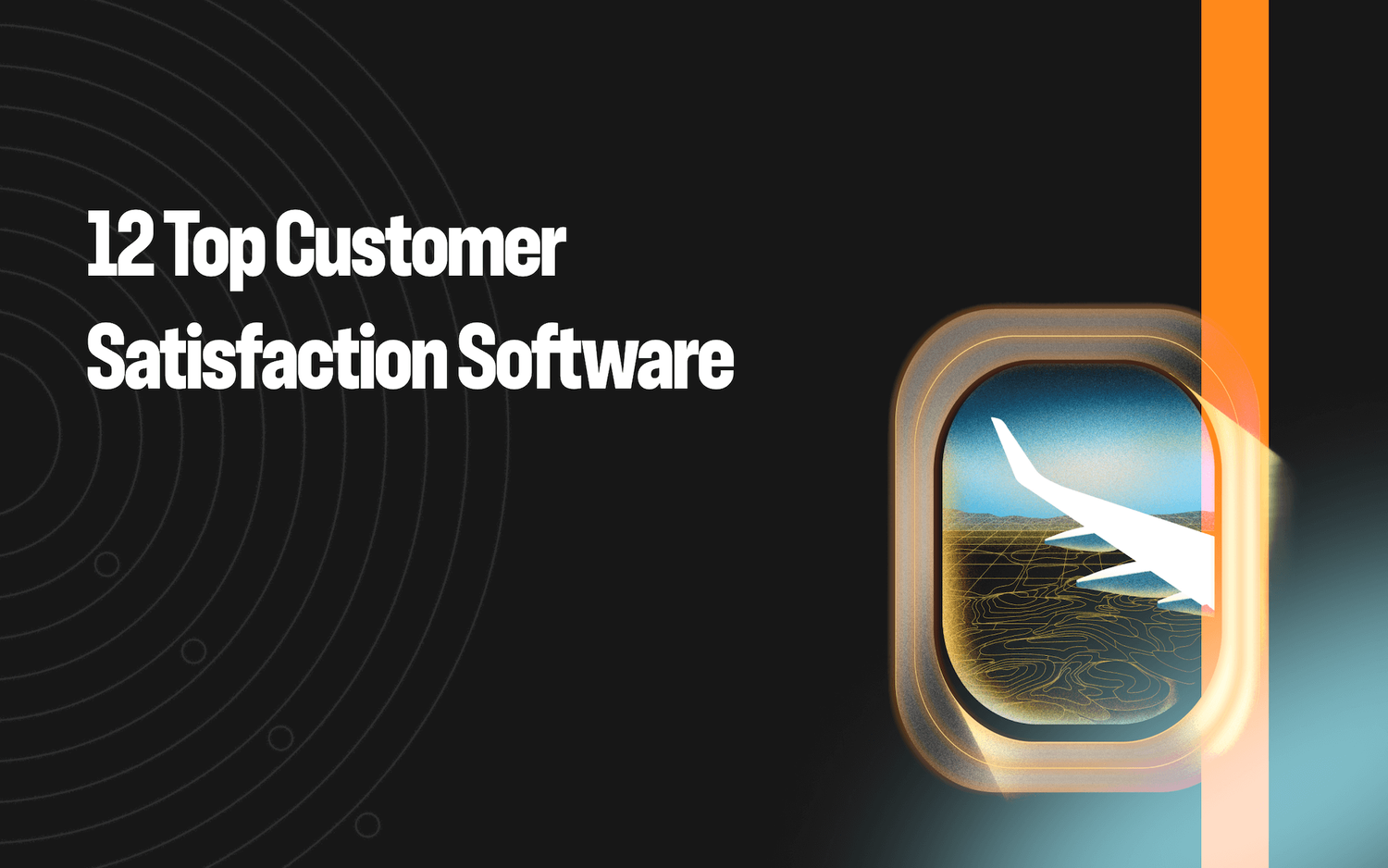