Level AI’s Sentiment Analysis: Generative AI for Understanding Human Emotions and Customer Satisfaction
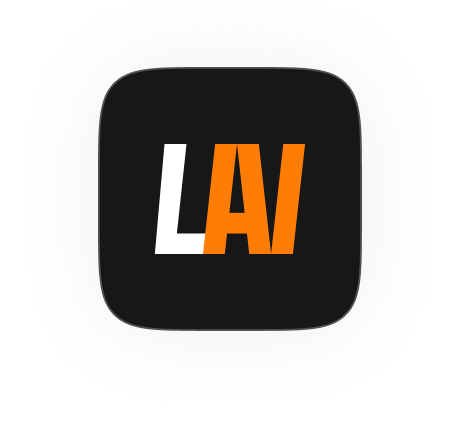
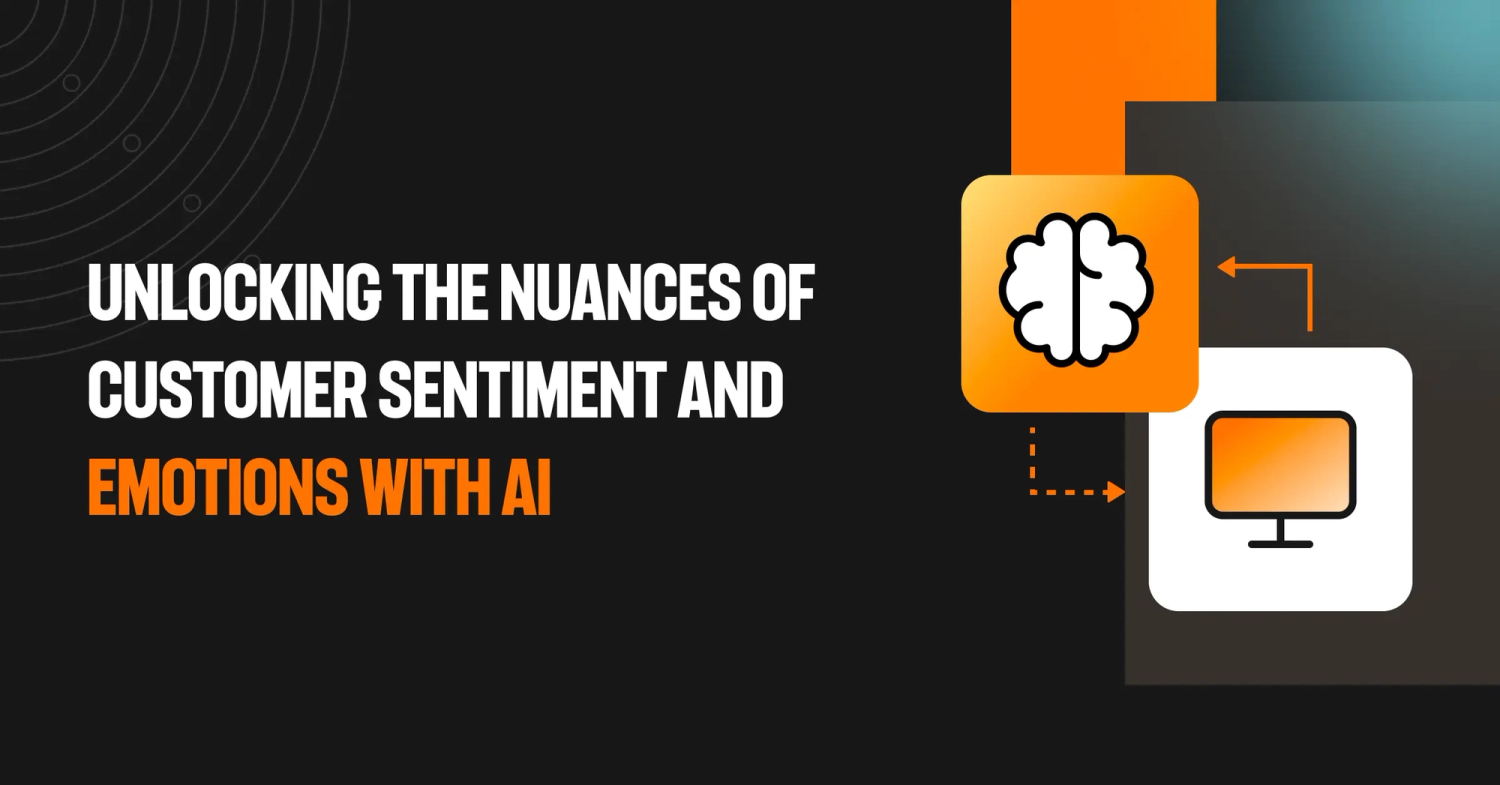
In today's ever-evolving landscape of customer experience and service, businesses are on a relentless quest for innovative solutions to unlock a deeper understanding of customer experience. While traditional CSAT and keyword-based sentiment analysis techniques have long served as the cornerstone for gauging customer sentiment, their limitations are becoming increasingly evident, leaving businesses wanting a more nuanced and insightful approach.
One of the primary limitations of traditional methods is their tendency to categorize customer feedback into simply "positive" or "negative," failing to capture the rich tapestry of emotions and motivations that drive individual responses. This binary approach to customer sentiment analysis lacks a scale that can differentiate the intensity of different emotions, can lead to misinterpretations, and hinder effective action, leaving businesses unable to quickly address the full spectrum of customer issues.
Furthermore, legacy sentiment analysis methods often rely on rudimentary keyword matching and rule-based algorithms, resulting in inaccurate or misleading scores. For example, a frustrated customer may say, “Oh, wonderful! I just love spending half my day on hold with your customer service team,” and be falsely interpreted as very positive as it contains many positive keywords. This reliance on simplistic techniques can mask critical insights and prevent businesses from identifying the true drivers of customer satisfaction or dissatisfaction. As a result, businesses struggle to effectively tailor their strategies to address the underlying factors influencing customer sentiment.
Feedback from CSAT scores often results in an inverted bell curve. This means businesses mostly hear from either very satisfied or very dissatisfied customers, with little to no feedback from the vast middle ground, resulting in biased and very misleading data.
Finally, traditional methods often provide only a high-level overview of customer sentiment, lacking the ability to delve deeper into the "why" behind the feedback. This absence of root cause analysis leaves businesses unable to address the underlying issues that drive customer satisfaction or dissatisfaction. Consequently, businesses struggle to proactively address issues and prevent them from escalating, ultimately impacting customer loyalty and brand reputation.
Generative AI-based sentiment score
These limitations paint a clear picture of the need for a more advanced and nuanced approach to sentiment analysis. Level AI's Sentiment Score AI is built from the ground up to really understand, explain, and quantify customer emotions.
Unlike traditional keyword and phrase-detecting sentiment analysis tools, the AI is like a good conversationalist. It waits for the customer to finish speaking and takes it all into context for analyzing emotions and sentiments. For example, when a customer says: “I’m a long-time customer and have always recommended your products to my friends. Every time I call though, the customer service is a letdown,” our model will accurately classify as “Disappointment” as it understands the initial sentence is the context for why the customer is particularly disappointed in the service. When working with textual interactions like chat, email, or sms, the AI also considers punctuation like exclamation marks to accurately detect emotions.
It also goes beyond just detecting positive and negative sentiments to identify and tag a wide variety of emotions and the degrees (very positive, mildly negative, etc.) associated with them across multiple channels. This all culminates in a single sentiment score from 0 to 10 that can accurately represent the customer’s true experience of the interaction. It also works as a much better representation of customer satisfaction, as the illustration below shows a normal distribution of customer sentiment that is not polarized to extremes like traditional CSAT. With most customer data, we have found a median sentiment score of 6 to be prevalent.
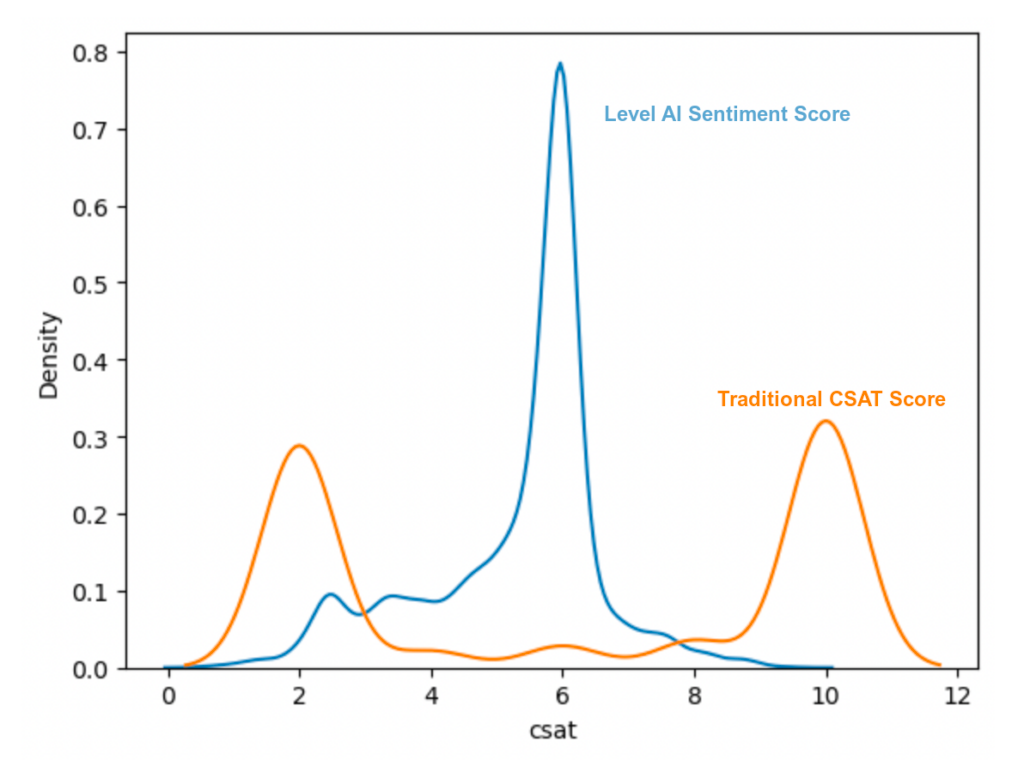
The AI model takes a novel non-linear approach, assigning varying weights to sentiments throughout a conversation. This means we don't just focus on the initial impression because customers rarely contact support because they are happy about something. Instead, we recognize that the way an agent handles an issue later in the interaction can have a lasting impact on the customer's overall experience. That's why we give higher weight to sentiments expressed later in the conversation, highlighting the ability of agents to turn a negative situation into a positive one.
The illustration below shows how customer sentiment changes while interacting with an agent. The customers are different, but they have expressed the same sentiments; the duration of the conversation is also similar, i.e., about 5 minutes. We chart the ebb and flow of emotions during an interaction, providing higher weightage to sentiments occurring later vs those occurring at the beginning.
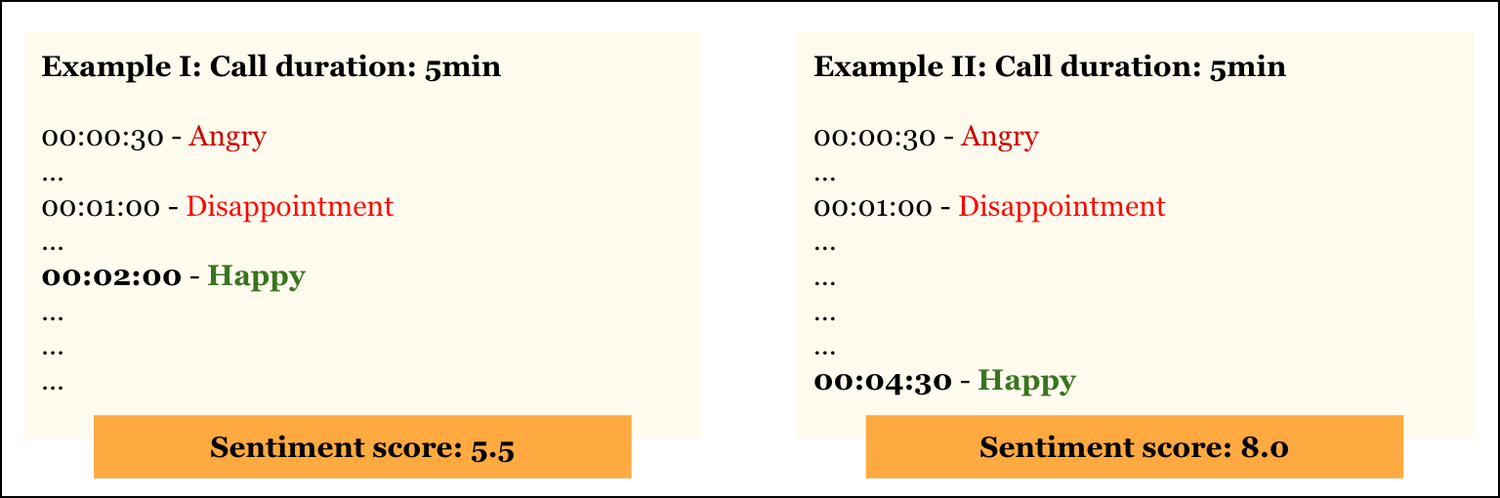
Level AI’s sentiment AI, along with all of our other AI models, is also continually evaluated with hundreds of test samples from our customer data every month. A team of human annotators assign sentiments to parts of the conversations and benchmark the results against the AI. We have found that the AI model’s accuracy, when compared to a consensus of human annotators, is similar to that of an average native language speaker. Users can also report incorrect sentiments by clicking the thumbs-down icon next to it, as shown below. This allows the AI model to train and improve further.
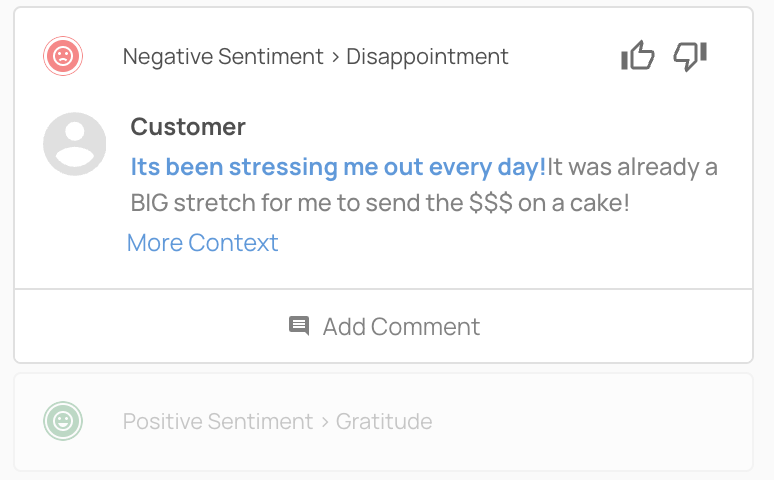
Sentiment Tags and Customization
In addition to a sentiment score, the AI tags moments in a conversation where an emotion is detected, as shown in the image below.

Though the model can detect a wide variety of emotions, we have found that 8 emotions (Anger, Annoyance, Disappointment, Disapproval, Worry, Admiration, Gratitude, and Happiness) tend to impact customer satisfaction the most. These emotions are all assigned certain weights by default that indicate the degree of positive or negative impact on CSAT.
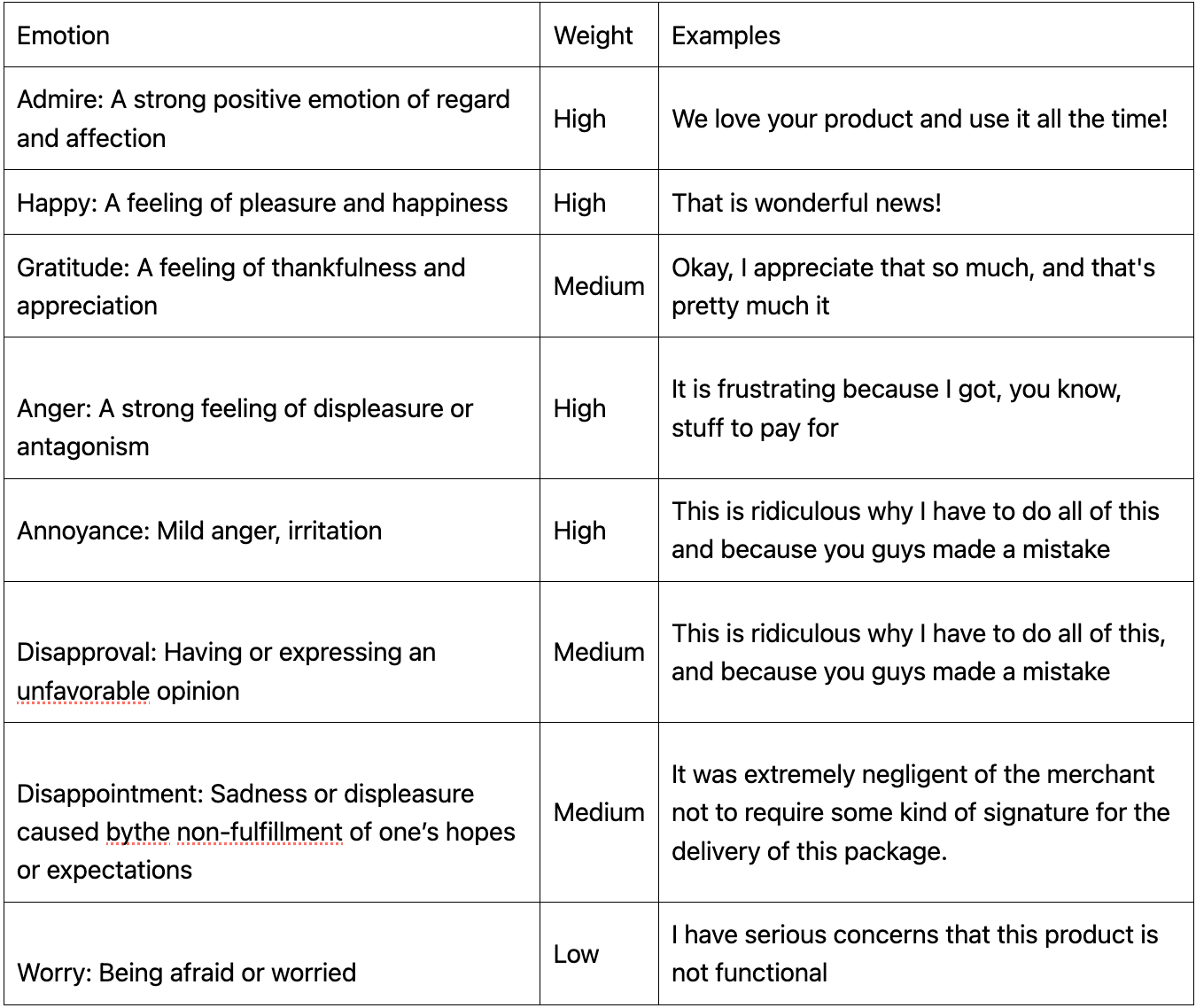
There are also nuances that are taken into consideration; for example, the AI knows that a customer saying “Thank you” at the end of the call is not necessarily indicative of positive customer satisfaction. The industry also shapes the nature of customer calls; a customer calling a hospital worried is understandable. To make sure sentiment analysis works for you, Level AI provides settings to turn on/off certain emotions and assign weights to them that reflect your business environment.
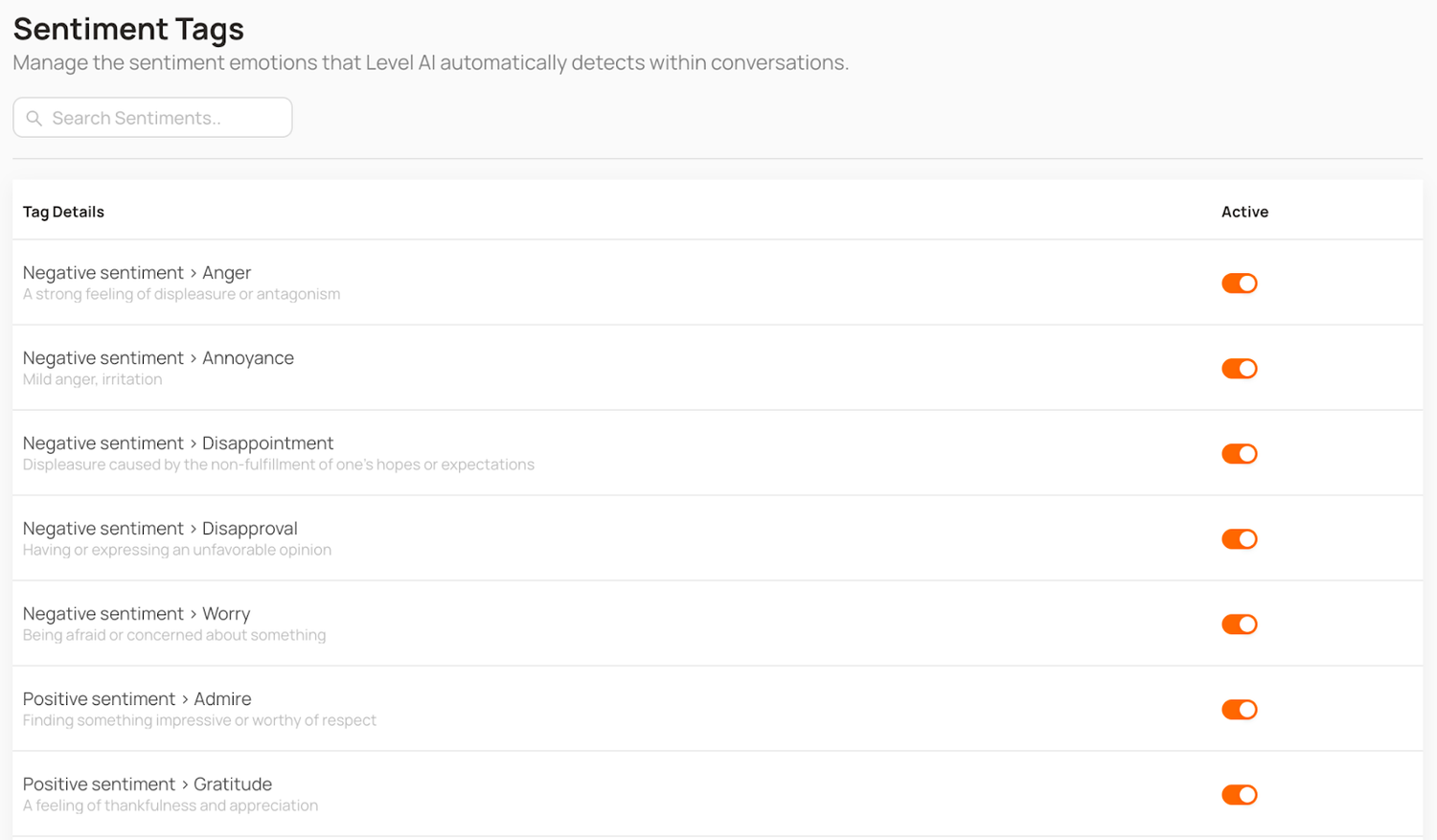
Going further, sentiment tags can be used to create custom reports with sentiment-related dimensions sliced with any business metrics. Users can also filter calls by one or more detected emotions, and they can dig into individual calls to understand the impact of customer emotions and agent responses.
This nuanced approach unlocks a treasure trove of insights. Not only can we pinpoint the exact moments where customer sentiment shifts, but this allows us to identify the specific behaviors that might have triggered these shifts. Did a friendly greeting at the start of the interaction establish a positive tone? Did an empathetic response to a complaint turn frustration into appreciation? By understanding these triggers, we can provide targeted coaching and training to help agents consistently deliver outstanding customer experiences.
Keep reading
View all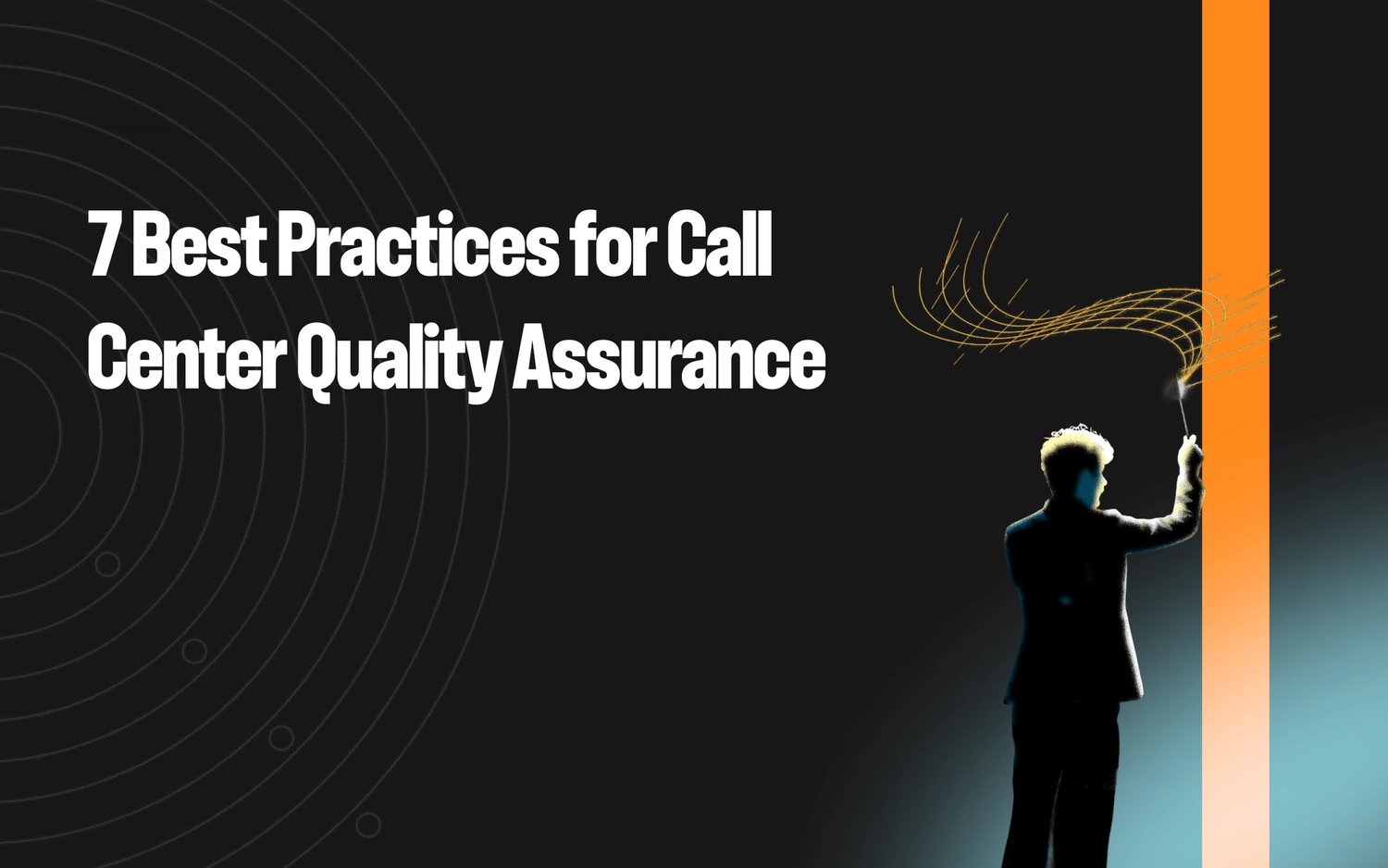
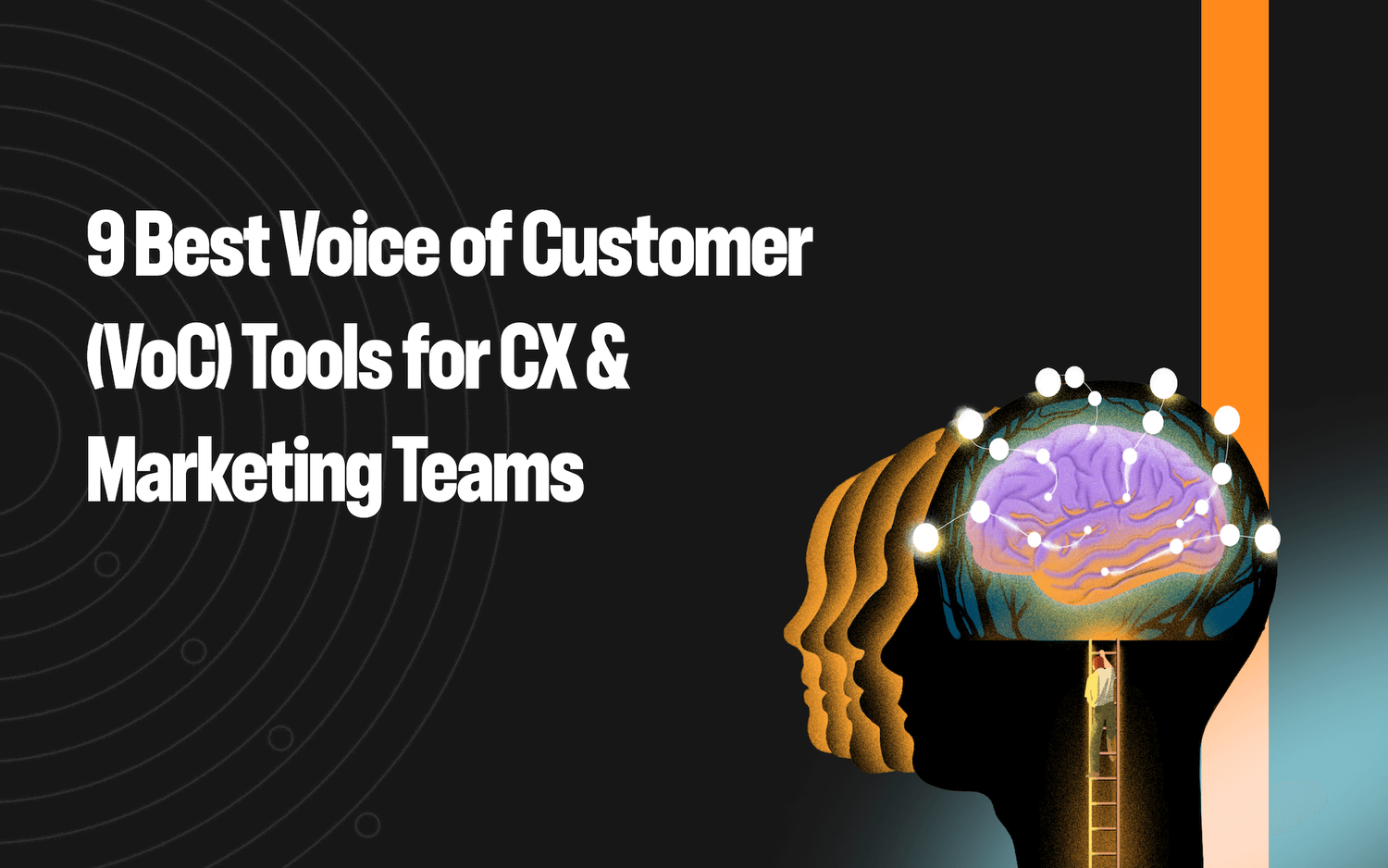
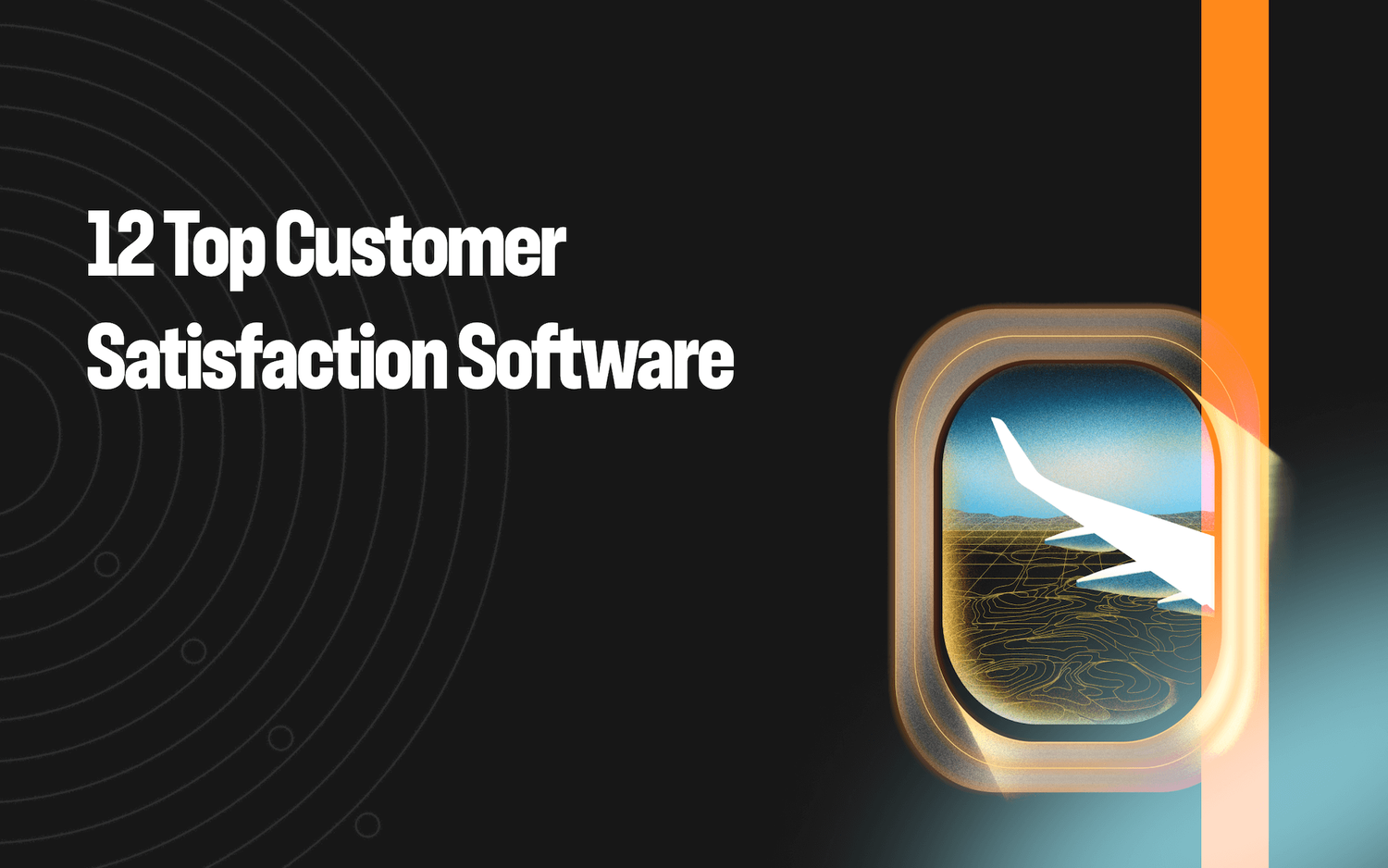