Improving the Customer Experience with Artificial Intelligence and Machine Learning
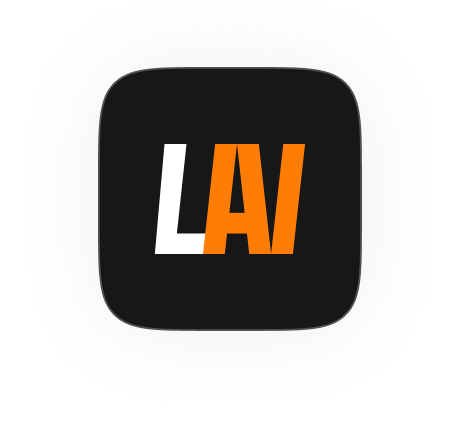

As customer service expectations rise and customer experience (CX) becomes a key competitive differentiator, businesses are increasingly looking to smart technologies such as artificial intelligence (AI) and machine learning in customer service (ML) to provide improved customer service capabilities.
With the advancement of machine learning in customer service, companies now have the opportunity to take their CX operations to the next level by automating and streamlining processes, improving customer self-service capabilities, and enabling virtual agents to provide 24/7 support services that can assist customers quickly and effectively.
Machine learning in customer service is driving more actionable insights from the wealth of contact center data. With modern technological capabilities, ML tracks each individual customer interaction across all channels– whether via texts, emails, or live calls with a contact center agent.
To learn more about the capabilities and applications of machines that are able to understand human language, watch our recent webinar with Forrester.
What Is Machine Learning?
Oxford Language defines machine learning as “the use and development of computer systems that are able to learn and adapt without following explicit instructions, by using algorithms and statistical models to analyze and draw inferences from patterns in data.” In other words, you might say that machine learning is programming a computer to program itself.
What Is Machine Learning in Customer Service?
To apply machine learning in customer service, the business opportunity is in what we “teach” the computer to do. AI can do many useful tasks to improve customer service, such as:
- Answering basic customer inquiries via a chatbot or auto attendants
- Finding relevant information during a live call to resolve customer problems faster
- Collecting performance data to enhance QA and improve training
To learn how to handle a task, AI models need significant amounts of call data specifying which words and phrases to look for and how to respond to them. For example, when deciding which department to send a customer support call to, AI will need to know what data is relevant in order to route the call. There is a large volume of data that pours into contact centers from:
- Chatbots,
- Phone calls,
- Email requests,
- Social media
This data then is able to be used to train AI models to streamline and improve the customer experience.
Knowledge Base
In addition to training data, AI models need a way to point customers and agents to valuable information. The “thinking” part of the AI takes cues from agents or customers. It then uses it to search its “memory,” containing all the information that the contact center allows it to, enabling it to give direction. To implement machine learning in customer service tools successfully, you need a strong, well-organized knowledge base to rely on.
A knowledge base is a complex collection of information that spans every part of your business. It’s where an AI goes to find answers to the questions that it receives. The better the knowledge base, the more relevant the answer will be. This should be everything that you can think of that might be useful to helping an agent or customer. Then, over time, you may also add information as new situations, solutions, and requirements appear. The AI model itself can help this process, learning to pull correct answers, and even alerting the humans that run it when it needs updating.
In the contact center, this same technology can be used in conjunction with semantic AI models to solve problems faster. When a customer makes a query, the AI can interpret the meaning, search its entire knowledge base, and return suggestions to an agent. This can lead to rapid results with high accuracy, and can be effective in solving a client’s problem without needing to transfer them to another department, and can avoid the need for long hold times. In this case, the AI has access to every department’s data, which means it can point the agent to a pertinent solution. For these reasons and more, to make best use of AI and machine learning in customer service in your contact center, a well-maintained and comprehensive knowledge base is a necessity, not a luxury.
NLP Vs. NLU
As for the rise of Natural Language Understanding (NLU) in customer service, it is important to first address the difference between NLU and NLP (natural language processing). NLU differs from NLP in that it focuses on finding the human intent behind a language prompt. This differentiator is often referred to as semantic intelligence. Instead of looking for keywords to match, NLU models can process the meaning of the words, opening the door for more varied use cases. NLU is not only capable of making customer service interactions more enhanced by interpreting what customers want, but also assisting live agents to help them have more productive conversations.
Ways ML and AI Can Enhance Customer Service
AI tools for customer service can assist both the customers themselves and your contact center team. Some machine learning in customer service technologies are designed to interface directly with customers, while others use AI to support your agents and enhance your contact center’s capabilities.
Here are 4 AI technologies that you can implement in your contact center to enhance the customer experience.
Chatbots. One application of machine learning in customer service is building conversational AI models to respond to customers via chatbot.
Recently, it’s become an expectation to see a chatbot on an online store waiting to answer simple questions like “I need help with billing.” This prevents human agents from having to answer basic questions that involve pointing the customer to the right page. This frees up agents to focus on problems that are too complicated for AI to handle.
Auto attendants. Chances are, most customers have talked to a machine on the phone a few times. Or a lot of times.
Machine learning in customer service has driven the creation of automated answering that can understand basic questions. As with chatbots, it doesn’t seem like much, but automating simple problems makes a big deal once a business starts to scale. AI improves the customer experience by giving immediate answers rather than making them wait in a queue to ask something simple.
Big data analytics. Big data analytics in customer service are useful in early identification of issues that are frustrating to customers, and also provide valuable intelligence to companies about which services are most requested actions at the CX level. In addition, AI enhances the customer experience by aggregating data and consumer insights about common purchases, and based on these, the model is able to give recommendations. AI can do many similar tasks with large data sets and also keeps the information well-organized, categorized, and able to be sorted and searched for insights and trends.
Agent co-pilots. NLU-powered AI really shines when paired up with humans as. By searching for customer intent, an AI assistant can populate useful information to an agent’s screen automatically. This allows the agent to focus on the customer experience without getting distracted, and empowers the agent to have the right information at the exact time it’s needed.
Without an AI co-pilot, an agent has to manually find the related files or items while they are trying to guide them through the conversation. As a result, customers can get frustrated with the wait. An AI model with semantic intelligence helps keep the conversation moving forward, improving the customer journey.
Contact Center QA. AI can be implemented alongside transcription software to grade every piece of communication that passes between agents and customers.
Most human QA teams are only able to grade 2% of an agent’s calls, for example, which is an extremely small sample. With the right AI technology for contact centers, you can get the full picture of how an agent is performing and also more opportunities to grade, since the auditor can select to grade any of one of the 100% of conversations captured. This allows QA teams to focus on the most glaring opportunities for improvement, rather than getting distracted or losing time manually searching for missed customer services behaviors or tasks.
How to Start Using Machine Learning for a Better Customer Experience Today
Hiring a team of data scientists and spending years on research and development isn’t the only way to see the benefits of high-quality AI and ML tools. Contact center platform tools such as Level AI’s can offer customizable, advanced NLU models that can be implemented and integrated into client systems quickly.
If you’re interested in learning more about how artificial intelligence and machine learning in customer service can be used to improve the customer experience (CX) at your business, watch our webinar with Forrester.
If you’re interested in getting started, contact us for more information.
Keep reading
View all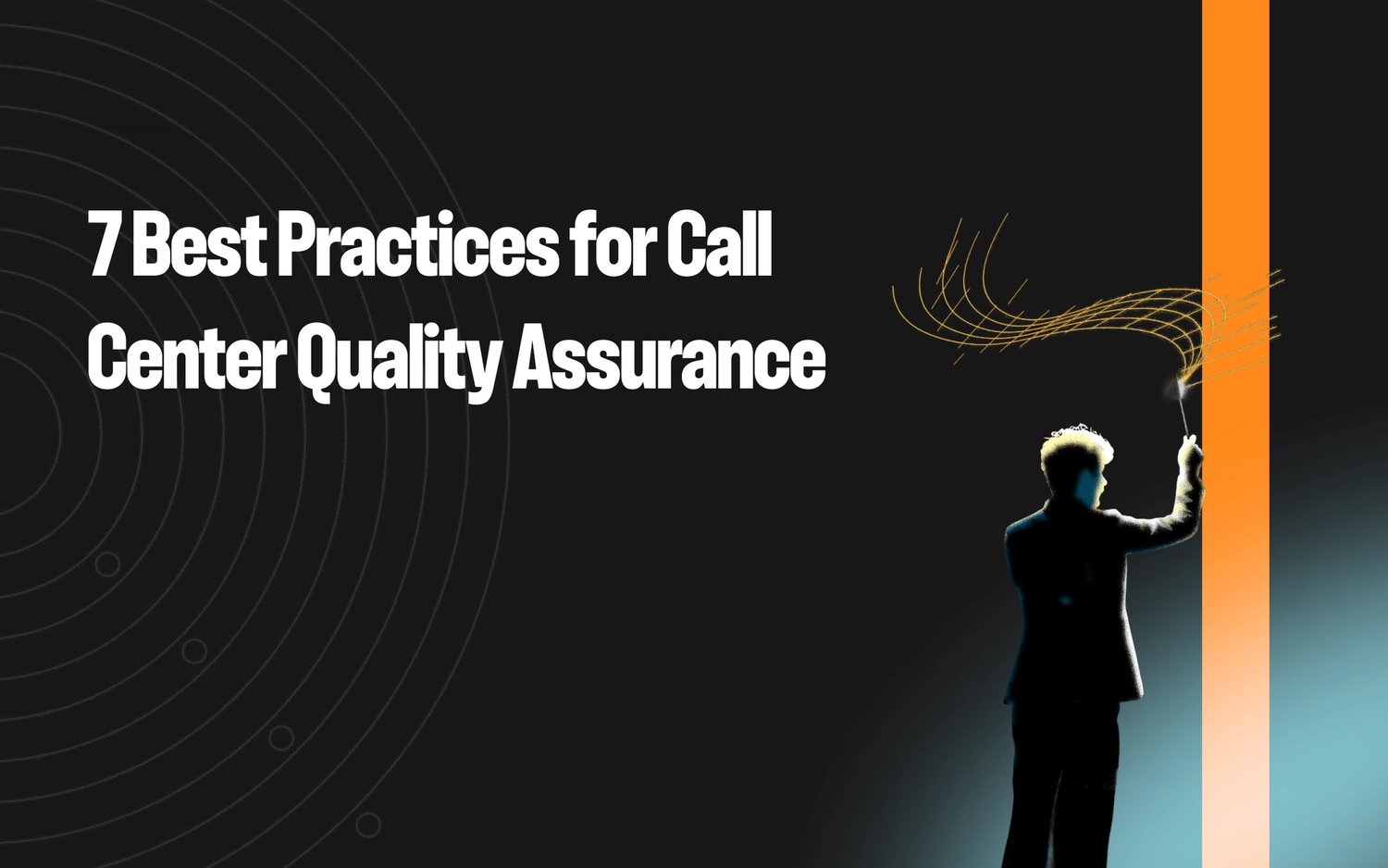
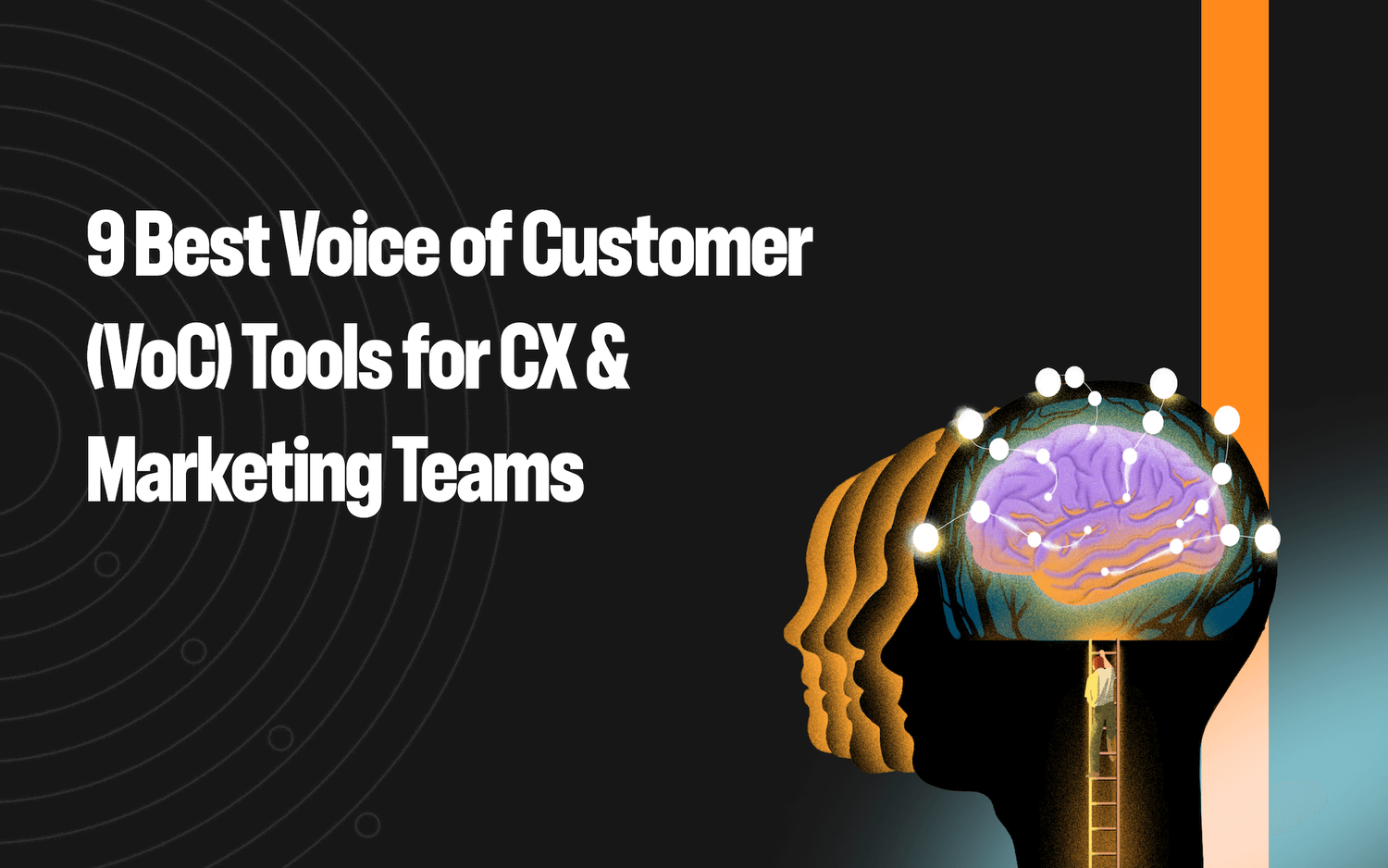
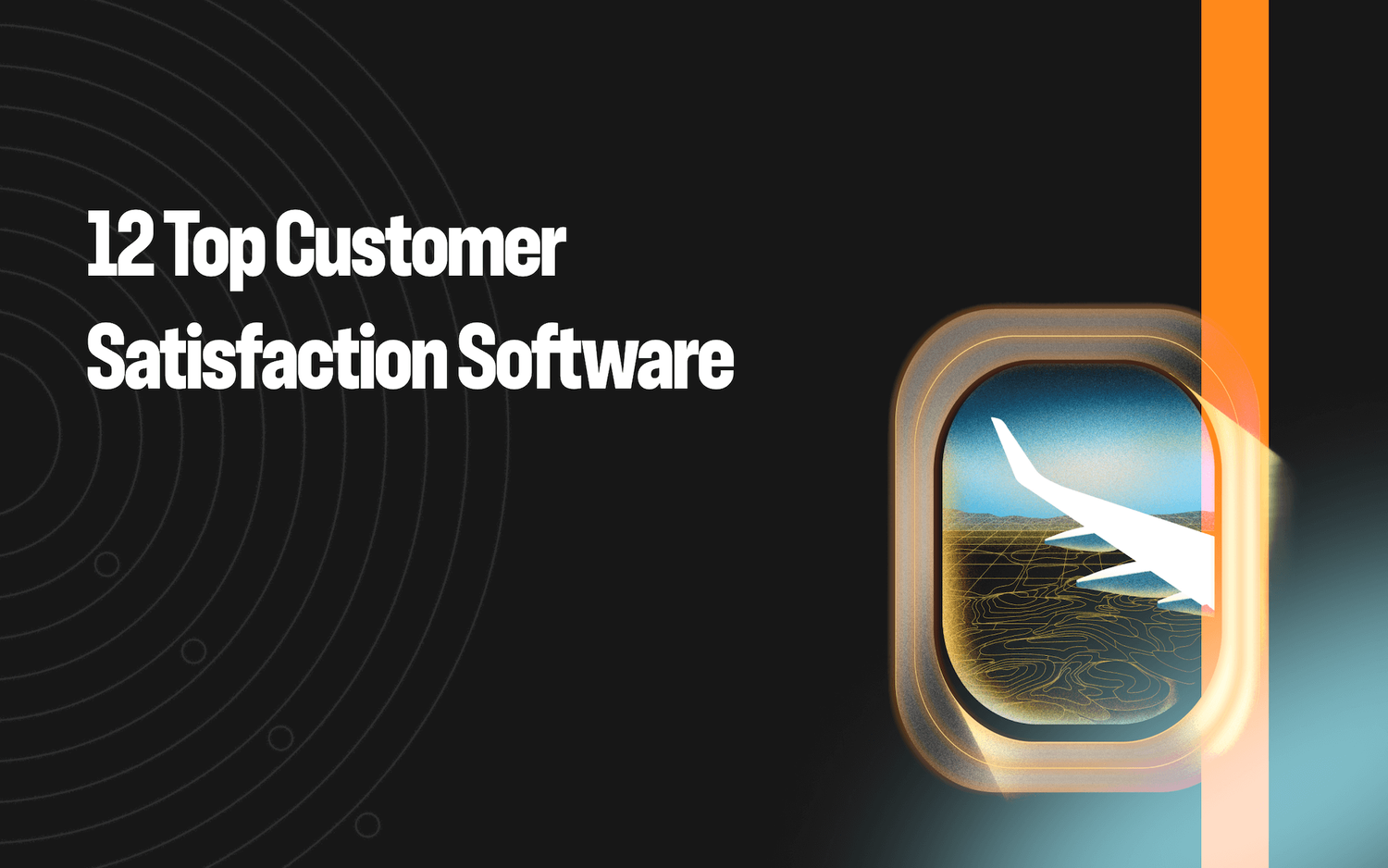