What Is Natural Language Understanding (NLU)?
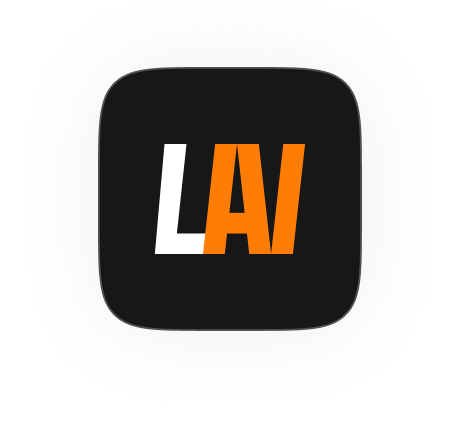
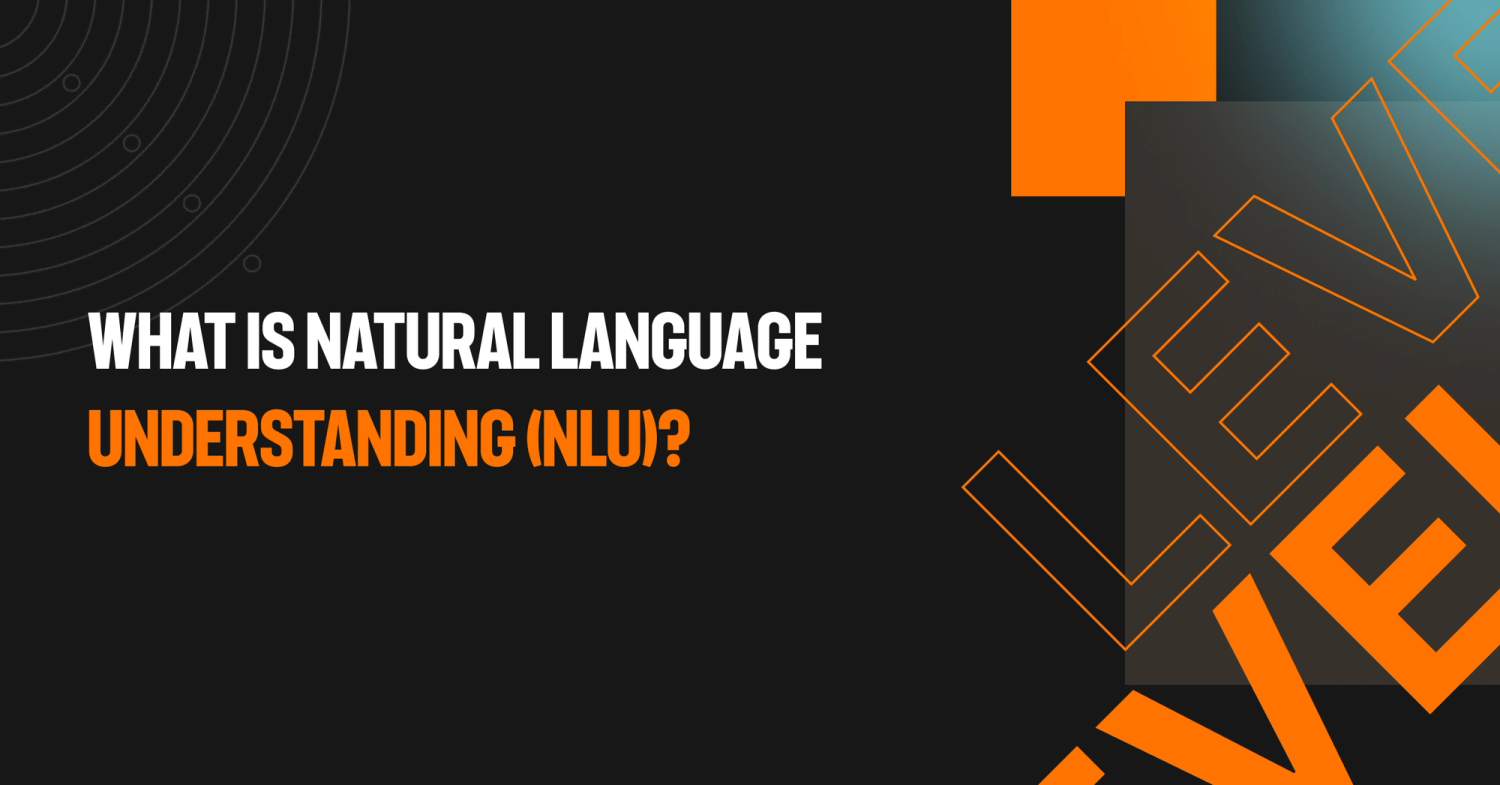
Natural language understanding (NLU) is an artificial intelligence-powered technology that allows machines to understand human language. The technology sorts through mispronunciations, lousy grammar, misspelled words, and sentences to determine a person’s actual intent. To do this, NLU has to analyze words, syntax, and the context and intent behind the words.
Natural Language Understanding (NLU) connects with human communication’s deeper meanings and purposes, such as feelings, objectives, or motivation. It employs AI technology and algorithms, supported by massive data stores, to interpret human language.
NLP Vs. NLU Vs. NLG: What’s The Difference?
Although natural language understanding (NLU), natural language processing (NLP), and natural language generation (NLG) are similar topics, they are each distinct. NLU and NLG are components of NLP. Let’s take a moment to go over them individually and explain how they differ.
Natural language processing (NLP) is a field of computer science, artificial intelligence, and linguistics concerned with the interactions between machines and human (natural) languages. As its name suggests, natural language processing deals with the process of getting computers to understand human language and respond in a way that is natural for humans.
Natural language understanding (NLU) is a branch of natural language processing that deals with extracting meaning from text and speech. To do this, NLU uses semantic and syntactic analysis to determine the intended purpose of a sentence. Semantics alludes to a sentence’s intended meaning, while syntax refers to its grammatical structure.
Additionally, NLU establishes a data structure specifying relationships between phrases and words. While humans can do this naturally in conversation, machines need these analyses to understand what humans mean in different texts. While NLP analyzes and comprehends the text in a document, NLU makes it possible to communicate with a computer using natural language.
Natural language generation (NLG) is a process within natural language processing that deals with creating text from data. This can be done through summarization, description, or narration.
The difference between natural language understanding and natural language generation is that the former deals with a computer’s ability to read comprehension, while the latter pertains to a machine’s writing capability.
NLG refers to creating human speech responses from data inputs. Text-to-speech services can convert this text into a speech format. To generate text, NLG algorithms first analyze input data to determine what information is important and then create a sentence that conveys this information clearly. Additionally, the NLG system must decide on the output text’s style, tone, and level of detail.
Typical computer-generated content will lack the aspects of human-generated content that make it engaging and exciting, like emotion, fluidity, and personality. However, NLG technology makes it possible for computers to produce humanlike text that emulates human writers. This process starts by identifying a document’s main topic and then leverages NLP to figure out how the document should be written in the user’s native language.
The computer produces text based on the outcome of this decision. For example, a computer can use NLG to automatically generate news articles based on data about an event. It could also produce sales letters about specific products based on their attributes.
How Does Natural Language Understanding Work?
The natural language understanding process has three main steps:
- Semantic analysis
- Syntactic analysis
- Discourse analysis
Semantic analysis is the first step in NLU. In this step, the system extracts meaning from a text by looking at the words used and how they are used. This process looks at the context of words to determine their meaning. For example, the term “bank” can have different meanings depending on the context in which it is used. If someone says they are going to the “bank,” they could be going to a financial institution or to the edge of a river.
Syntactic analysis is the second step in NLU. In this step, the system looks at the grammatical structure of a sentence to determine its meaning. This process focuses on the order of words and how they are combined to form phrases and clauses. For example, the sentence “The boy went to school” has a different meaning than “Went the boy to school?”
Discourse analysis is the third and final step in NLU. In this step, the system looks at the relationships between sentences to determine the meaning of a text. This process focuses on how different sentences relate to each other and how they contribute to the overall meaning of a text. For example, the discourse analysis of a conversation would focus on identifying the main topic of discussion and how each sentence contributes to that topic.
NLU systems use these three steps to analyze a text and extract its meaning. Additionally, NLU systems can use machine learning algorithms to learn from past experience and improve their understanding of natural language.
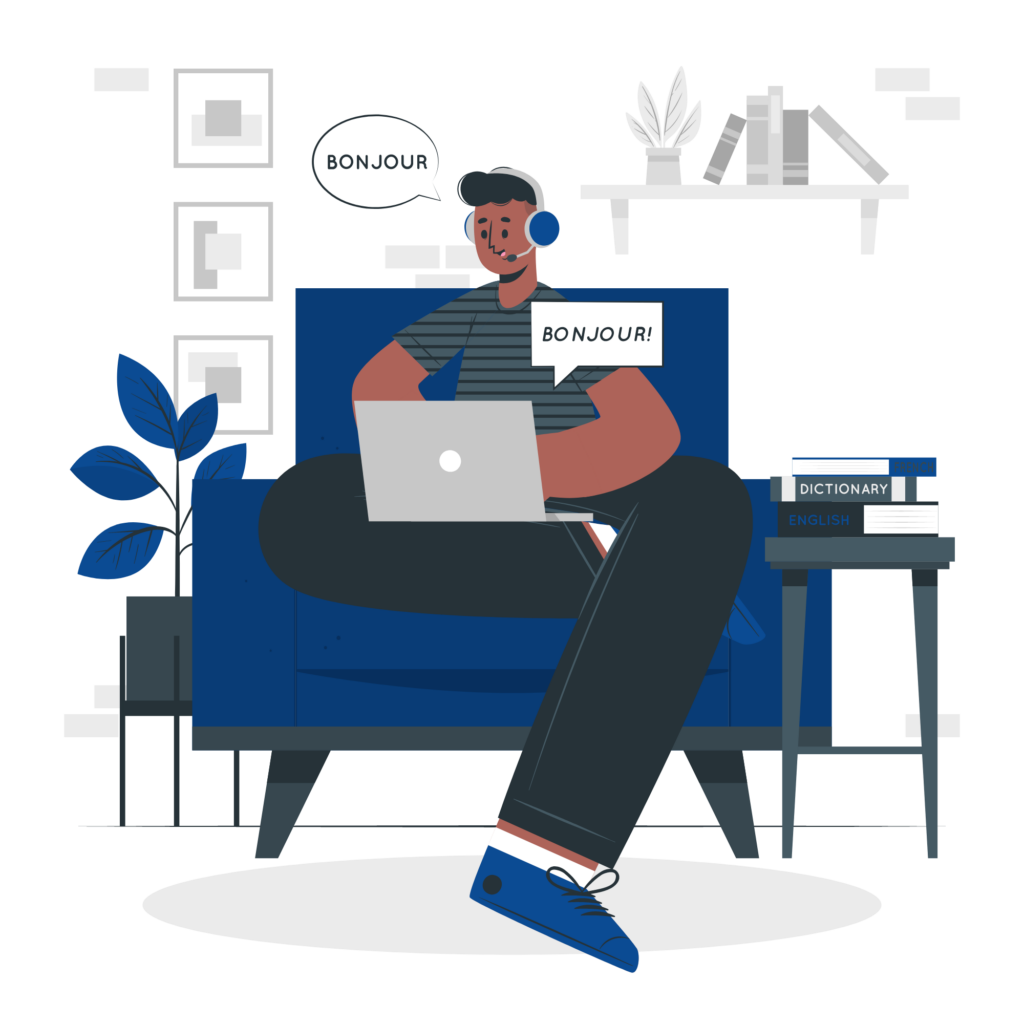
Why Is Natural Language Understanding Important?
The following are some of the reasons why NLU has gained traction, especially in business circles:
1. Human language is complex for computers to understand
Due to the fluidity, complexity, and subtleties of human language, it’s often difficult for two people to listen or read the same piece of text and walk away with entirely aligned interpretations.
If humans find it challenging to develop perfectly aligned interpretations of human language because of these congenital linguistic challenges, machines will similarly have trouble dealing with such unstructured data. With NLU, even the smallest language details humans understand can be applied to technology.
2. There’s a growing need to be able to analyze huge quantities of text contextually
NLU is improving the ways machines interpret human language. This branch of AI lets analysts train computers to make sense of vast bodies of unstructured text by grouping them together instead of reading each one. That makes it possible to do things like content analysis, machine translation, topic modeling, and question answering on a scale that would be impossible for humans.
With today’s mountains of unstructured data generated daily, it is essential to utilize NLU-enabled technology. The technology can help you effectively communicate with consumers and save the energy, time, and money that would be expensed otherwise.
3. Customers want to feel like they’re being listened to as individuals.
Consumers overwhelmingly want businesses to care more about them. Over 60% say they would purchase more from companies they felt cared about them. Part of this caring is–in addition to providing great customer service and meeting expectations–personalizing the experience for each individual.
In fact, according to Accenture, 91% of consumers say that relevant offers and recommendations are key factors in their decision to shop with a certain company. It isn’t easy to meet customers individually when there are so many. NLU software doesn’t have the same limitations humans have when processing large amounts of data. It can easily capture, process, and react to these unstructured, customer-generated data sets.
4. Many companies and consumers are already using it
By 2025, the NLP market is expected to surpass $43 billion–a 14-fold increase from 2017. Businesses worldwide are already relying on NLU technology to make sense of human input and gather insights toward improved decision-making.
With the advent of voice-controlled technologies like Google Home, consumers are now accustomed to getting unique replies to their individual queries; for example, one-fifth of all Google searches are voice-based. You’re falling behind if you’re not using NLU tools in your business’s customer experience initiatives.
The Impact of NLU on Customer Experience
Natural language understanding can positively impact customer experience by making it easier for customers to interact with computer applications. For example, NLU can be used to create chatbots that can simulate human conversation. These chatbots can answer customer questions, provide customer support, or make recommendations.
Also, NLU can generate targeted content for customers based on their preferences and interests. This targeted content can be used to improve customer engagement and loyalty.
Use Cases for Natural Language Understanding
There are many potential use cases for natural language understanding. Below are some examples:
Conversational chatbots
Chatbots are necessary for customers who want to avoid long wait times on the phone. However, if all they do is give simple answers, they’re not very helpful. With NLU (Natural Language Understanding), chatbots can become more conversational and evolve from basic commands and keyword recognition.
Chatbots offer 24-7 support and are excellent problem-solvers, often providing instant solutions to customer inquiries. These low-friction channels allow customers to quickly interact with your organization with little hassle. As a result, chatbots tend to produce higher customer satisfaction ratings.
NLU gives chatbots the power to:
- Easily answer FAQs
- Quickly facilitate shipping processes
- Provide personalized input and direction
NLU enables chatbots to cover what would otherwise be a human shortcoming. For example, it is difficult for call center employees to remain consistently positive with customers at all hours of the day or night. However, a chatbot can maintain positivity and safeguard your brand’s reputation.
Secondly, NLU instills some emotional intelligence in chatbots. It helps them understand sentiment and form responses accordingly. For example, the chatbot could say, “I’m sorry to hear you’re struggling with our service. I would be happy to help you resolve the issue.” This creates a conversation that feels very human but doesn’t have the common limitations humans do.
Sentiment analysis
Using NLU-based sentiment analysis, organizations can use otherwise unstructured customer data to understand what people are saying about their brand. This information is gathered by extracting emotions from text, providing insight at a micro level (like gauging the tone behind a social media post) and a macro level (like understanding overarching customer trends.)
This gives you a better understanding of user intent beyond what you would understand with the typical one-to-five-star rating. As a result, customer service teams and marketing departments can be more strategic in addressing issues and executing campaigns. The outcome is an immediate boost for your business.
Automated ticketing support
Manual ticketing is a tedious, inefficient process that often leads to delays, frustration, and miscommunication. However, this issue can be greatly improved by utilizing NLU. This technology allows your system to understand the text within each ticket, effectively filtering and routing tasks to the appropriate expert or department.
NLU does more than keywords. It understands the actual request and facilitates a speedy response from the right person or team (e.g., help desk, legal, sales). This provides customers and employees with timely, accurate information they can rely on so that you can focus efforts where it matters most.
NLU provides support by understanding customer requests and quickly routing them to the appropriate team member. This saves time for both customers and employees. Because NLU grasps the interpretation and implications of various customer requests, it’s a precious tool for departments such as customer service or IT. It has the potential to not only shorten support cycles but make them more accurate by being able to recommend solutions or identify pressing priorities for department teams.
Automated document review
Manual document review is time-consuming and error-prone, especially when you have to review multiple documents; you’re working with long texts full of industry-specific language, which lengthens the review process and makes it more susceptible to human mistakes.
Natural language understanding can help speed up the document review process while ensuring accuracy. With NLU, you can extract essential information from any document quickly and easily, giving you the data you need to make fast business decisions.
Use Of NLU And NLP In Contact Centers
A growing number of modern enterprises are embracing semantic intelligence—highly accurate, AI-powered NLU models that look at the intent of written and spoken words—to transform customer experience for their contact centers. In addition to making chatbots more conversational, AI and NLU are being used to help support reps do their jobs better.
With an agent AI assistant, customer interactions are improved because agents have quick access to a docket of all past tickets and notes. This data-driven approach provides the information they need quickly, so they can quickly resolve issues – instead of searching multiple channels for answers.
On average, an agent spends only a quarter of their time during a call interacting with the customer. That leaves three-quarters of the conversation for research–which is often manual and tedious. But when you use an integrated system that ‘listens,’ it can share what it learns automatically- making your job much easier. In other words, when a customer asks a question, it will be the automated system that provides the answer, and all the agent has to do is choose which one is best.
Conclusion
The purpose of NLU is to understand human conversation so that talking to a machine becomes just as easy as talking to another person. NLU will take unstructured data and make it more digestible. NLU will play a key role in extracting business intelligence from raw data. In the future, communication technology will be largely shaped by NLU technologies; NLU will help many legacy companies shift from data-driven platforms to intelligence-driven entities.
Keep reading
View all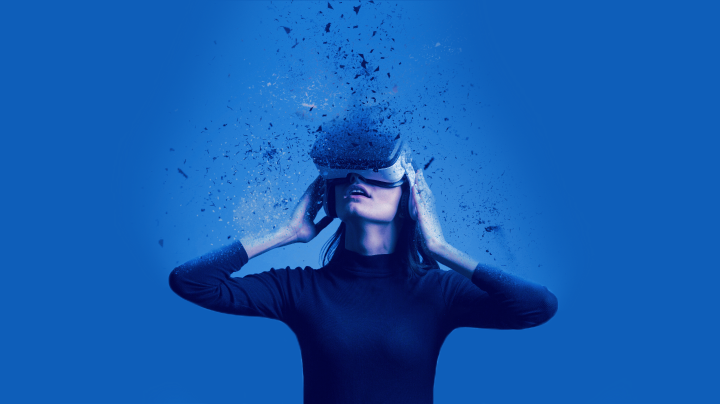
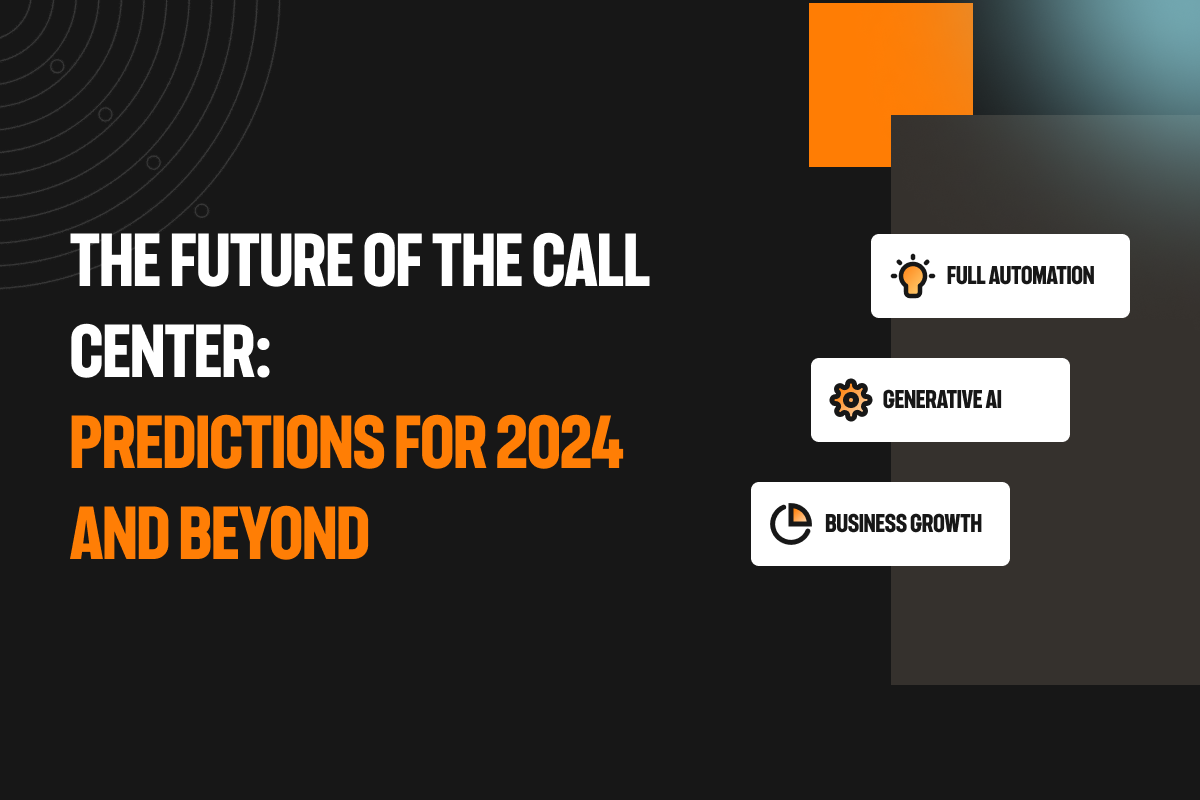
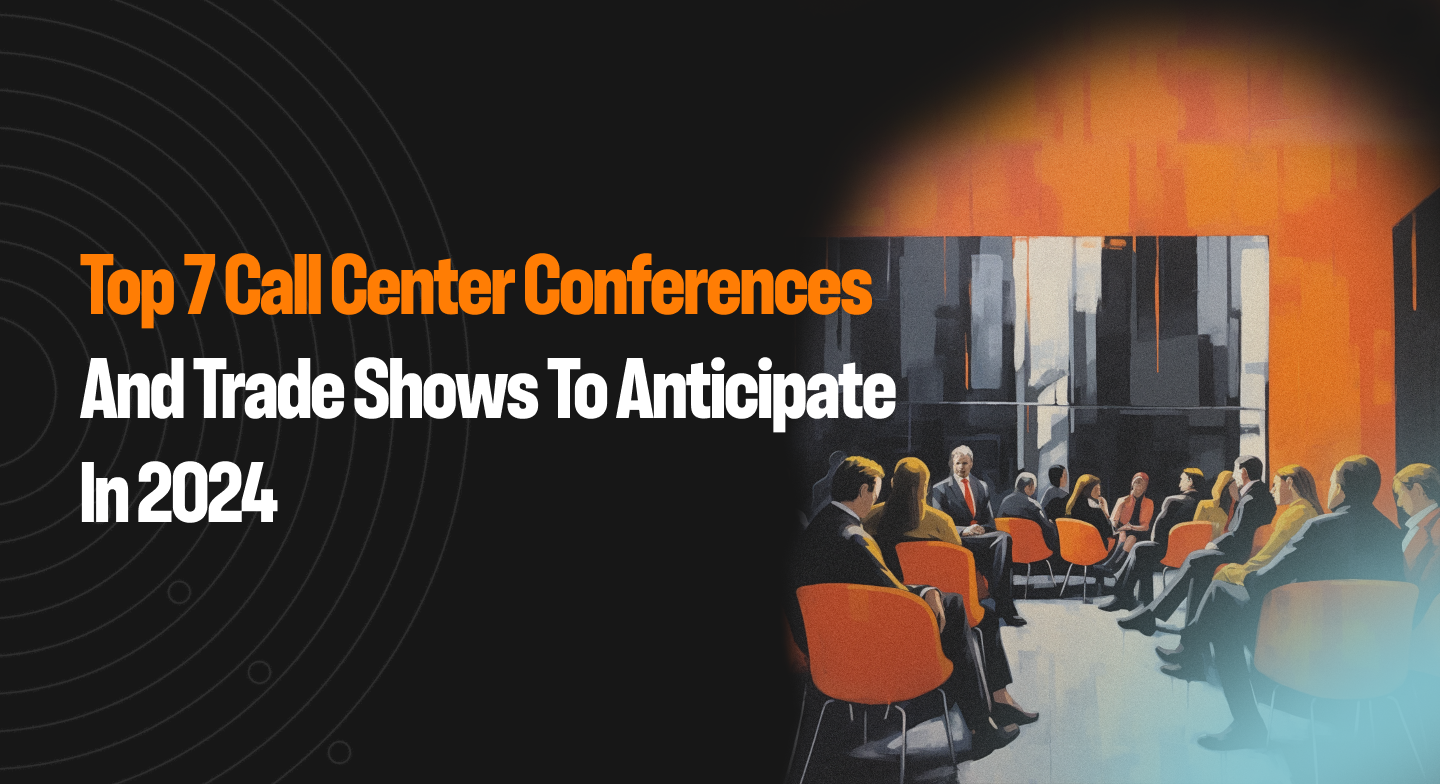