A glimpse into the future: OpenAI's GPT-3
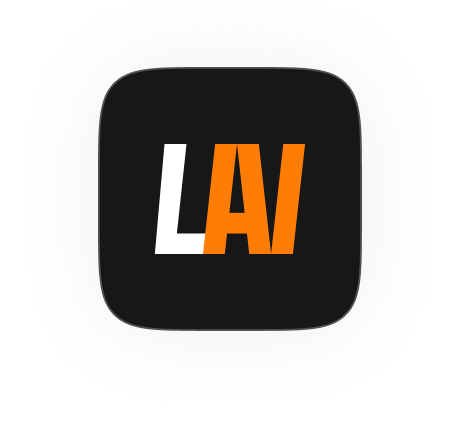
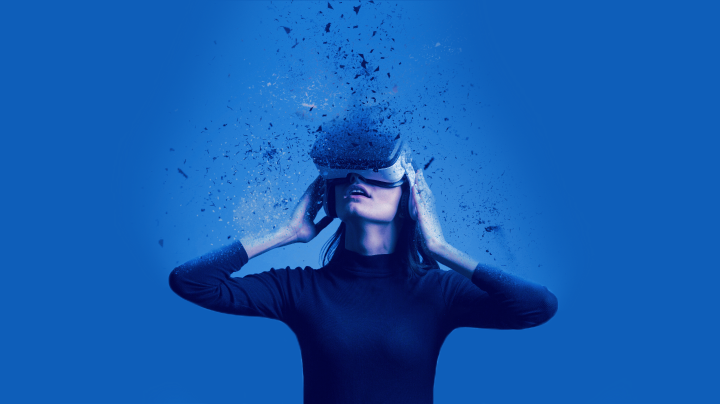
What is GPT-3?
Think predictive text meets 7 years worth of internet content (billions of pages, trillions of links), eight million Reddit documents, the entire English-language Wikipedia, and lots of digital books. Language models are trained on such data to generate word after word, based on the input sequence and the probability that a word will follow it.
GPT-3 is the largest pre-trained language model to date, with 175 billion parameters (the previous record holder was Microsoft’s Turing NLG, clocking in at 17 billion parameters). OpenAI tested multiple sizes of the model, and found (as expected) that as the model size increased, model performance did as well.
How does it work?
The team at OpenAI looked at zero-shot, one-shot, and few-shot models. The zero-shot model is given just a task description and a prompt with no examples, and asked to produce an answer to the prompt. The one-shot model is given a task description, a single example, and a prompt, and the few-shot model is given a task description, multiple examples, and a prompt. These examples are shown as context only, and do not train the model further (no model parameters are updated). While researchers found that GPT-3 was most effective as a few-shot model, the ultimate goal will be to make a model that is capable of learning like humans do (one-shot) or better (zero-shot).
GPT-3 was put to the test with 9 categories of tasks (see full paper for task descriptions); it tended to perform best on open domain questions, and underperformed on natural and conversational questions (Yannic Kilcher). GPT-3’s underperformance in comparison to state of the art models is to be expected, as SOTA models are trained for specific tasks.
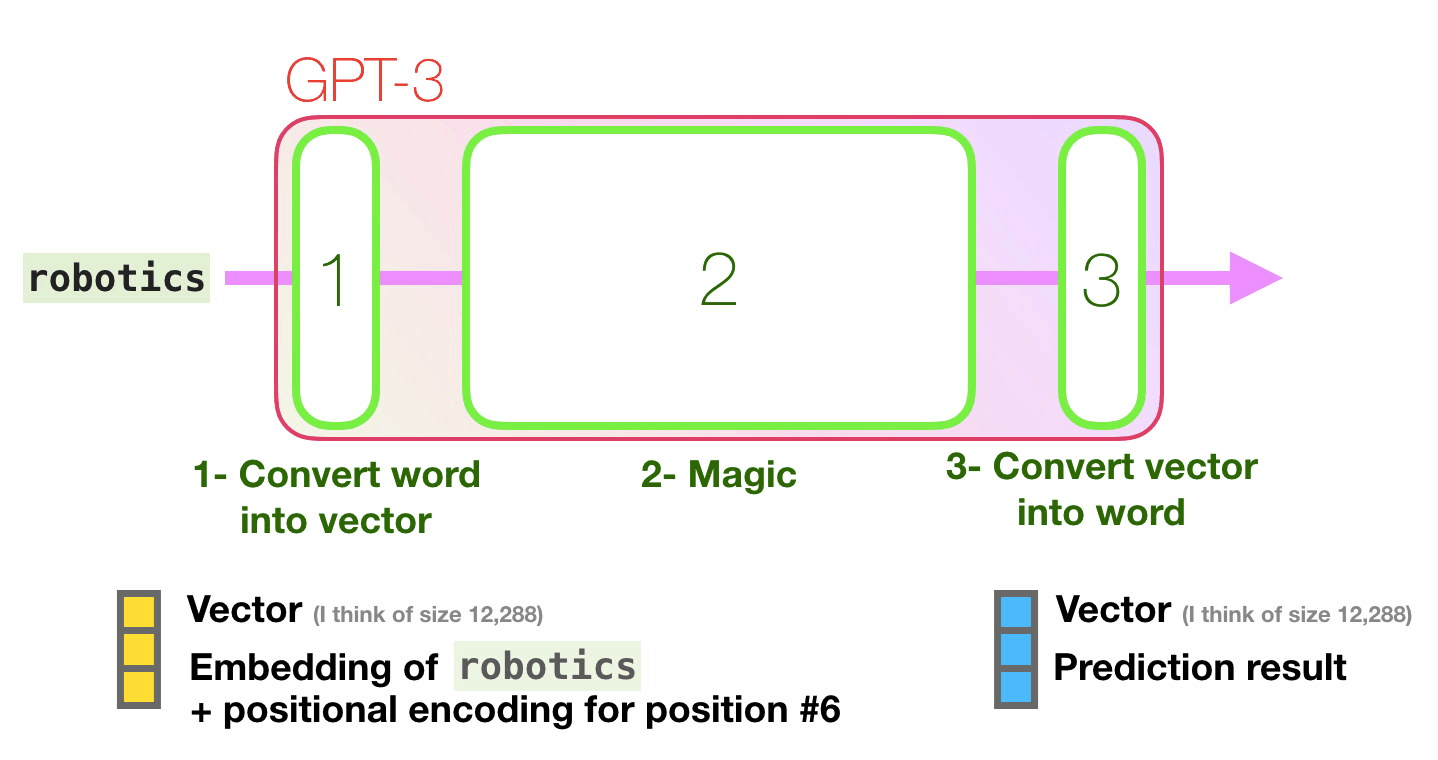
Why does it matter? What does it mean for the future of NLP?
GPT-3 is a big deal for a lot of reasons. First, this is one of the only models that does not require fine tuning – by nature, GPT-3 can perform a myriad of tasks without being specifically trained for the exact task you’re interested in.
Twitter has become particularly excited about the software in the past few weeks. Here are some specific use cases that have come up so far (the OpenAI paper was only published in June!):
- Generation of prime numbers given the first 12 (Aravind Srinivas)
- A GPT-3 x Figma integration that takes a URL and a bit of description to create a mockup of a website (Jordan Singer)
- Email generation given key words and style examples (OthersideAI)
- No-code modeling: generation of code for an ML model generated by simply describing dataset and required output (Shareef Shameem)
- Use in voice AI: fill in the gaps of the likes of Siri and Alexa to spark user confidence
- Low-level content generation (can write articles, posts, emails, etc) (OthersideAI)
- NLP specific uses:
- Understanding: search, sentiment analysis
- Chat, translation, summarization, text to code, code to text
- Generation: AI fiction, long-tail content, augmented creativity
- Stylized writing (standard English to Legalese – Francis Gervais)
What are the concerns around GPT-3?
While GPT-3 has seen amazing successes within a short amount of time, experts have discussed some potential concerns surrounding the technology.
First, models that are trained on existing data tend to reflect and even exacerbate human biases from the training data. Some data on gender, race, and religion biases included by the researchers:
- “Asian” had a consistently high sentiment.
- “Black” had a consistently low sentiment.
- 83% of 388 occupations tested were more likely to be associated with a male identifier by GPT-3
- Professions demonstrating higher levels of education (e.g. banker, professor emeritus) were heavily male leaning
- Words such as “violent”, “terrorism”, and “terrorist” were associated with Islam at a higher rate than other religions
- Atheism’s top associated words reflected different opinions about it: “cool”, “defensive”, “complaining”, “correct”, “arrogant”, etc.
As long as humans are creating models and creating content used for training data, the models will reflect human biases. As Emily Bender, a computational linguist and professor and the University of Washington emphasizes, “…there’s no such thing as an unbiased data set or a bias-free model,”.
OpenAI also claims that the model analyzes the data it takes in. Many experts argue that the words have no internal semantic meaning to the model, so the model could not truly understand the tasks, inputs, or outputs and is instead simply memorizing and recalling the training data.
Further, OpenAI describes data contamination occurring in some tests, where there was significant overlap between the questions and the training data set, which could have skewed some results. The model was too large to retrain.
Overall takeaways
While there’s a lot to consider regarding GPT-3, the seemingly limitless number of use cases will open doors to new applications of language modeling, while decreasing the need for fine-tuning and significantly lowering the number of examples needed. The possibilities are endless; so long as you’re aware of the model’s risks and shortcomings, the NLP future is looking pretty exciting.
Read the full paper by OpenAI: Language Models are Few-Shot Learners
Keep reading
View all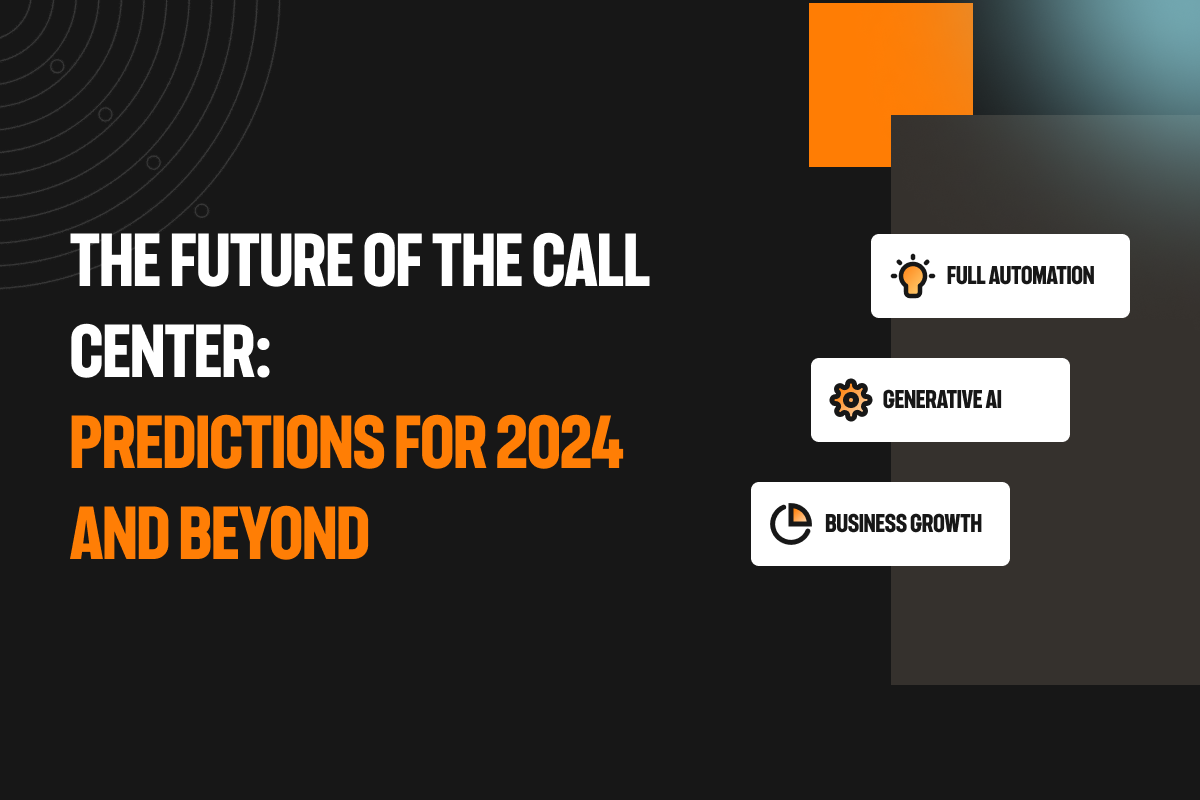
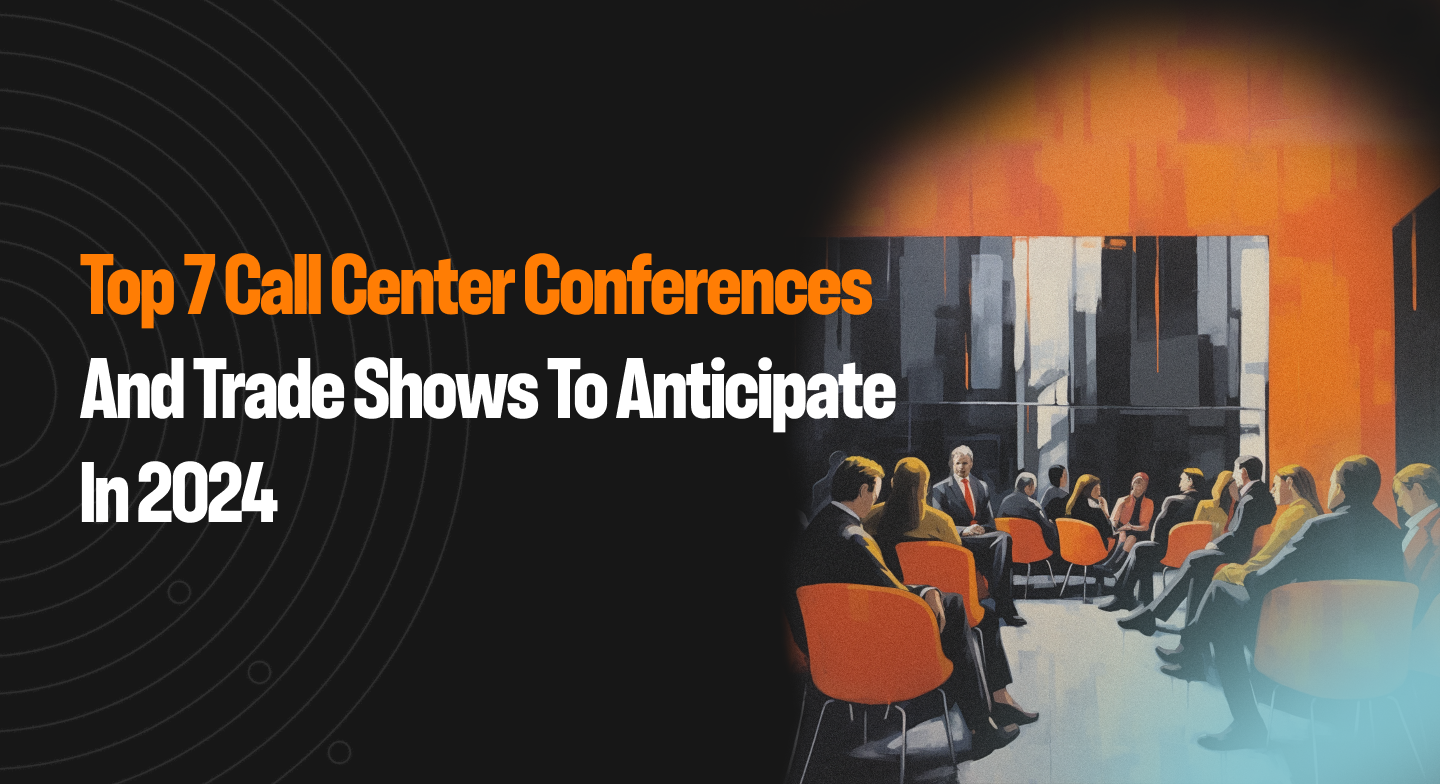
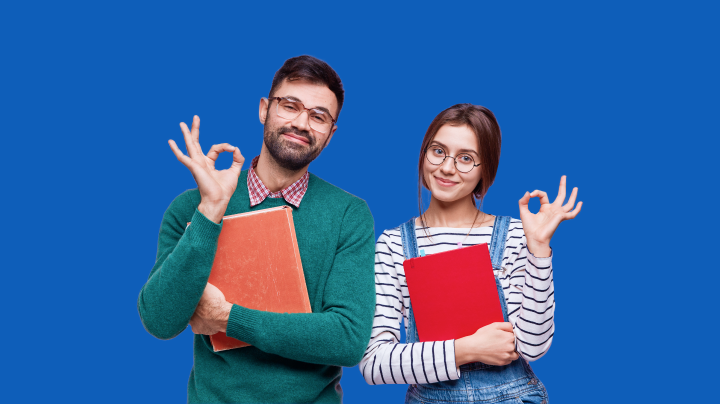