COVID-19 Emergency Unemployment Helpline: First Look
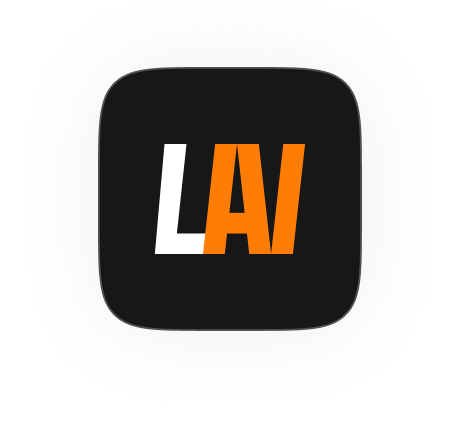
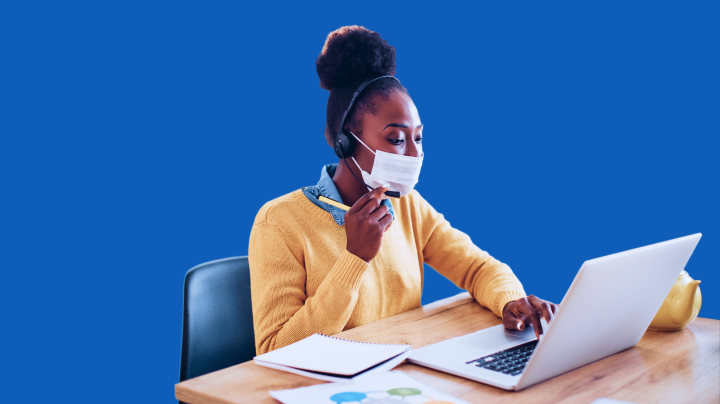
Challenge
When government unemployment offices were hit by unprecedented waves of claims following COVID-19 lockdown measures, it became nearly impossible for those in need to get through to an agent. Seeing so many vulnerable people struggle to speak to a human and receive information, Level AI decided to set up an independent unemployment helpline to provide information to people in New York, the hardest-hit state in the US.
With limited time, the Level AI team needed to onboard and train a number of new agents for the helpline. These agents had to know the ins and outs of the NY unemployment claims process and the ever-changing methods of filing for unemployment insurance – specifically being trained on information about eligibility, payout timelines, and other regulatory details.
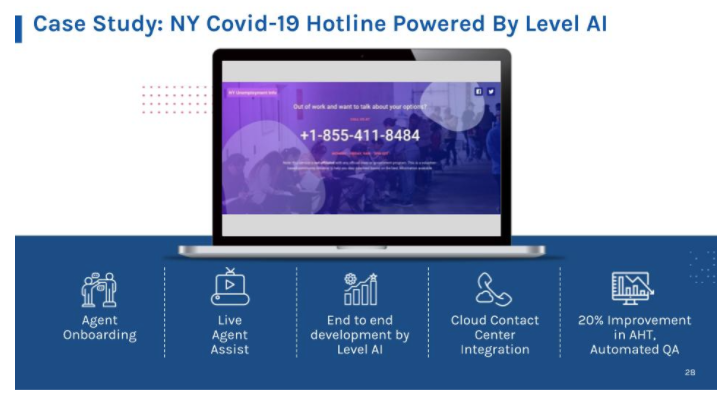
Solution
The helpline team implemented Level’s own Agent Assist AI to provide step-by-step guidance and relevant documentation to agents live while they assisted callers. The system also automated reviews to identify gaps in agent knowledge and identify risky situations where agents went outside of guidelines. This required connecting the chosen communication platform (Twilio Flex) to Agent Assist’s AI services and adding a component to the agent interface for tips and resources to be presented while speaking to callers.
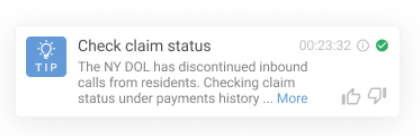
Engineering Implementation
Level AI used the audio streaming service from Twilio to get real-time input for agent assist AI. Multiple agents could be assisted in parallel with Level AI technology, essentially creating multiple instances of the AI agent.
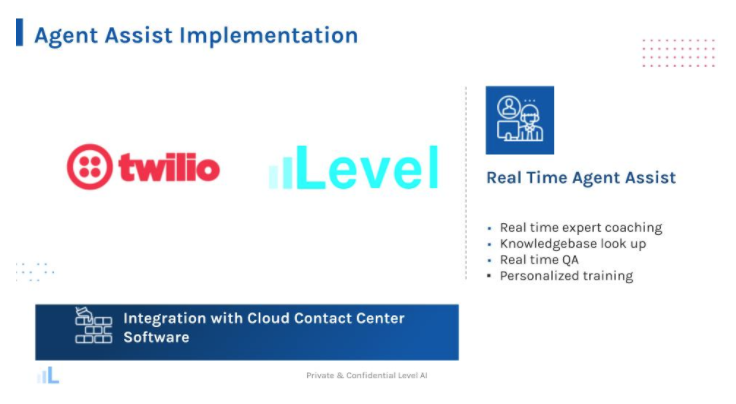
Results
Over a period of seven weeks (April 6 – May 2, 2020), agents staffing the Unemployment Info Helpline provided advice to hundreds of New Yorkers seeking help.
During the first week of operation, each call took an average of 14:12 (minutes:seconds), 10:44 of this time being spent in conversation with the caller. In the second week, we saw a dramatic decrease in average handling time (AHT) and average talk time (ATT), by 85% and 108% respectively. In the following weeks we continued to see improvements in both AHT and ATT. Weighted by the number of handled conversations, AHT and ATT decreased by an average of 14.7% and 18.7% week over week after Week One.
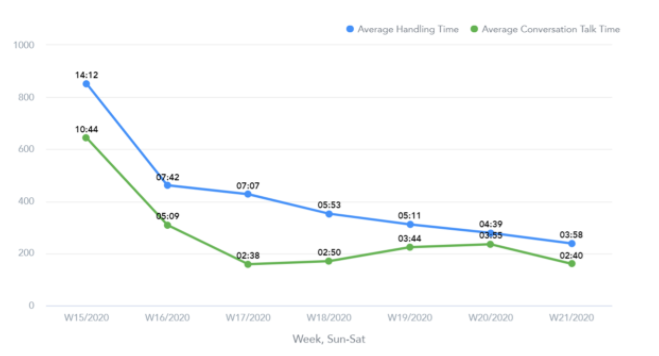
Simple repetition and practice for agents likely played a role in improving efficiency. At the same time Level’s agent assist system generated 2-5 knowledge assists, QA hints and warnings for each conversation. The agent assist was a definite factor in reducing the training time and AHT of the agents.
Automated QA allowed the team to identify areas where agents needed to improve. When these needed changes were identified by Agent Assist, the following weeks saw noticeable improvements in both handling time and talk time. When few or no needed changes were identified, efficiency suffered by as much as 31.4% in the following weeks.
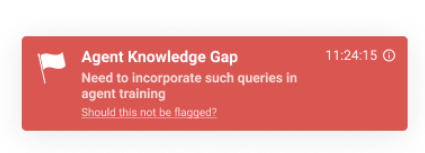

While on calls, the team heard overwhelming gratitude from people in need. When a post-call NPS survey was implemented in the second half of the project, the callers’ positive sentiment was matched by an average NPS score of 8.69 / 10.
What’s Next?
Contact center agents have a heroic job in most circumstances. Helping others solve problems is both rewarding and taxing when trying to balance providing a high degree of empathy and helping as many people as quickly as possible. Adding intelligent automation to help agents find answers faster can be a massive boon, but only when balanced correctly to provide just the right amount of information. It’s also much easier to find trouble spots when a machine does it automatically instead of requiring someone to manually go through each recording or transcript.
Keep reading
View all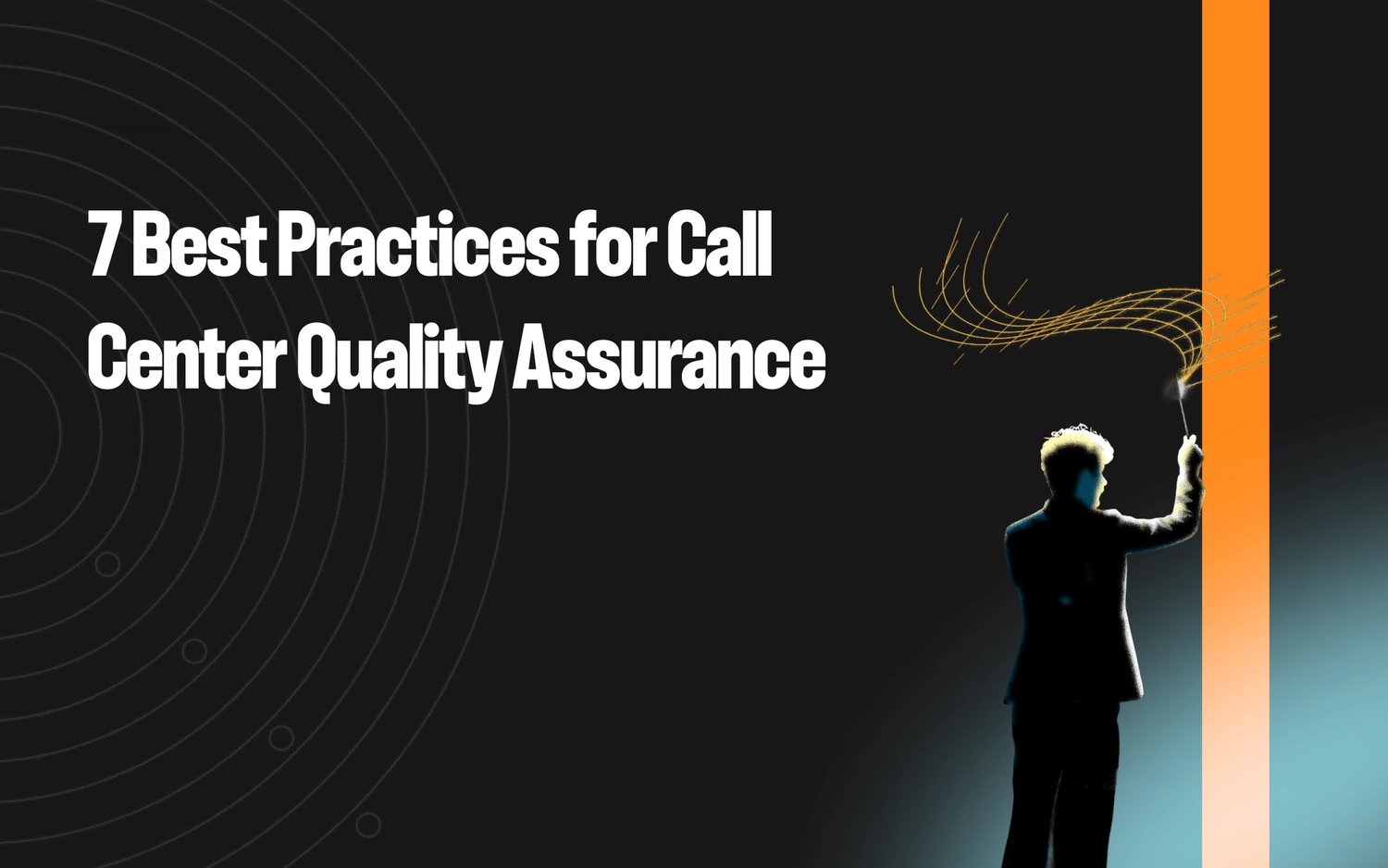
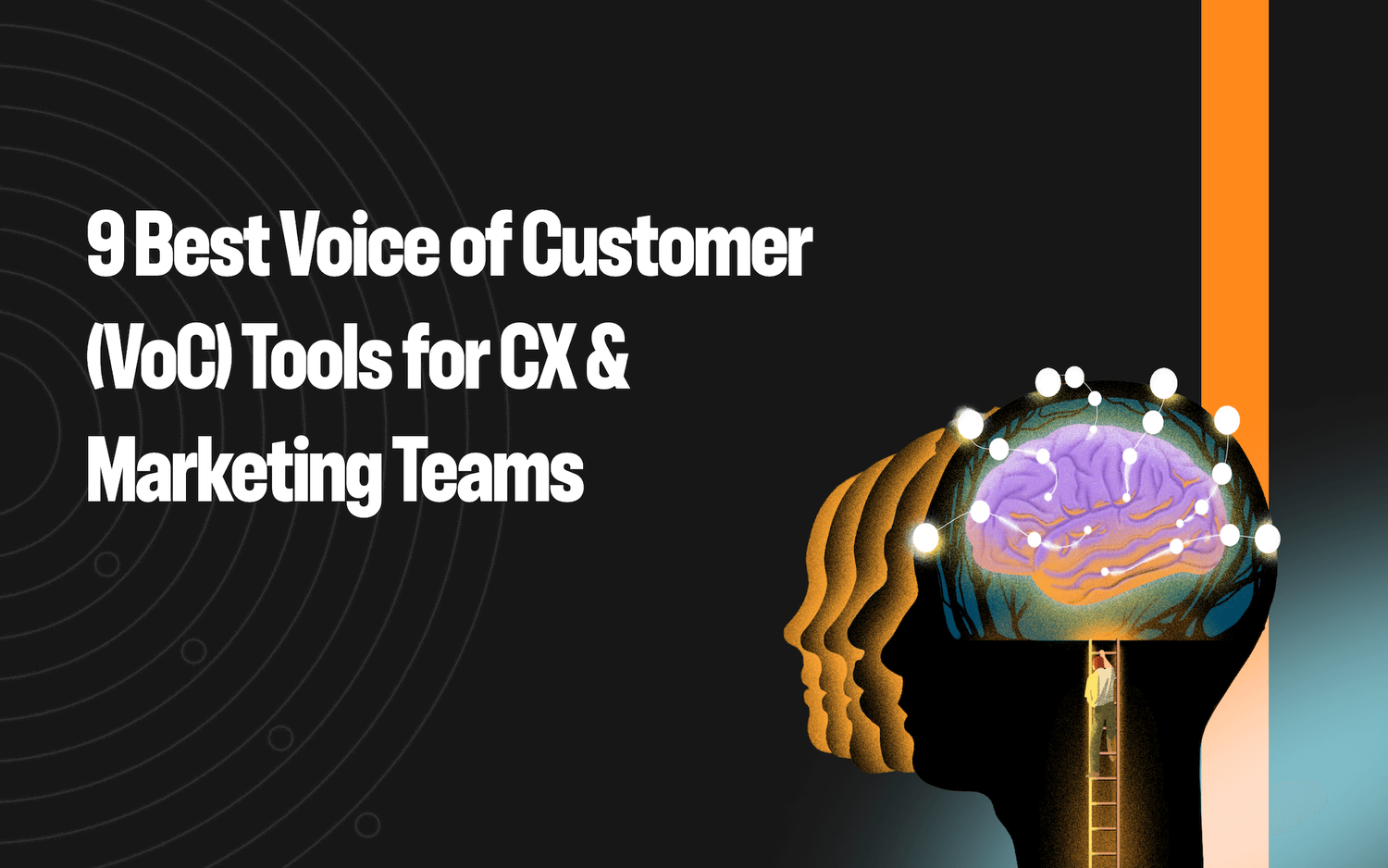
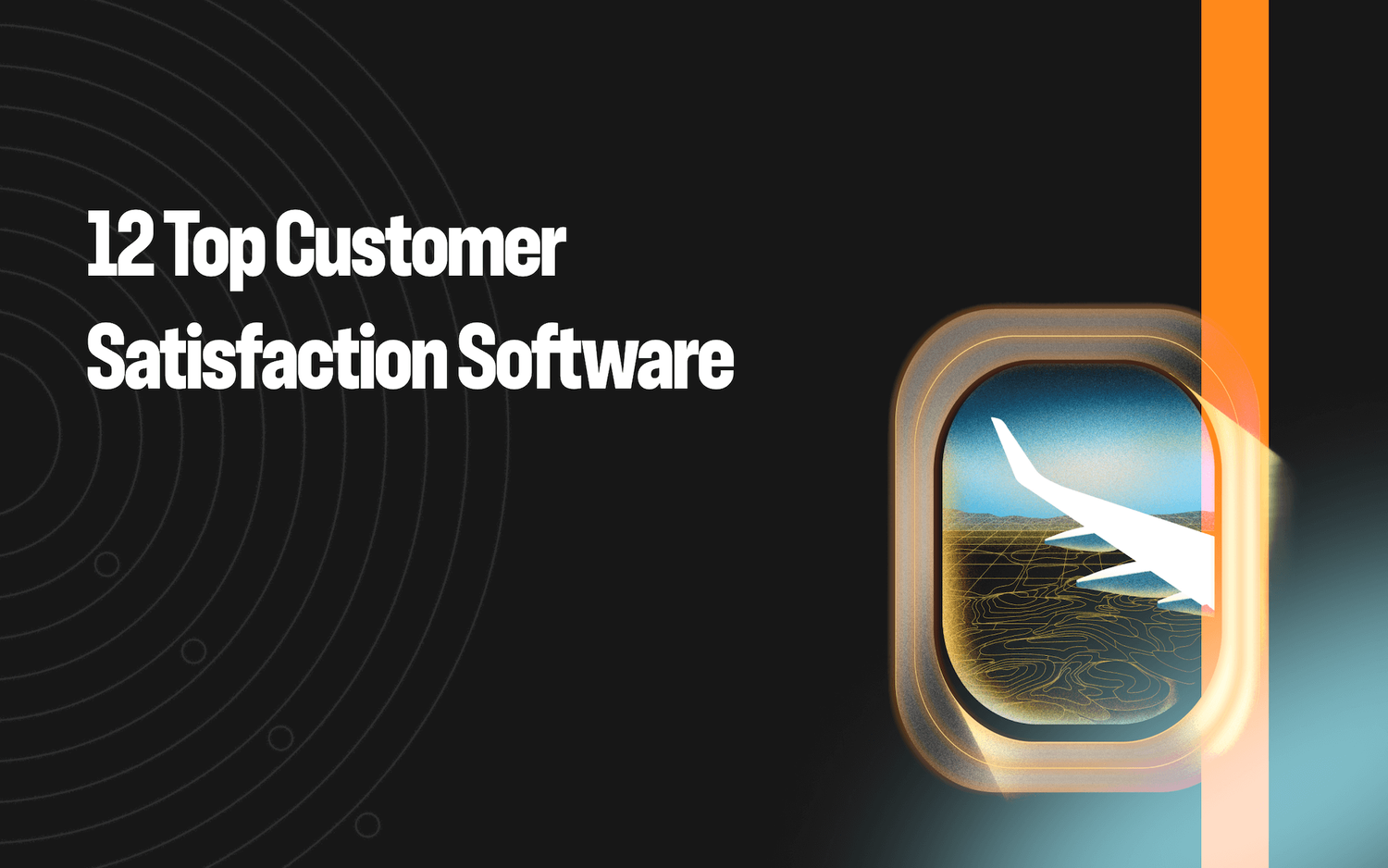