A Guide to Customer Interaction Analytics

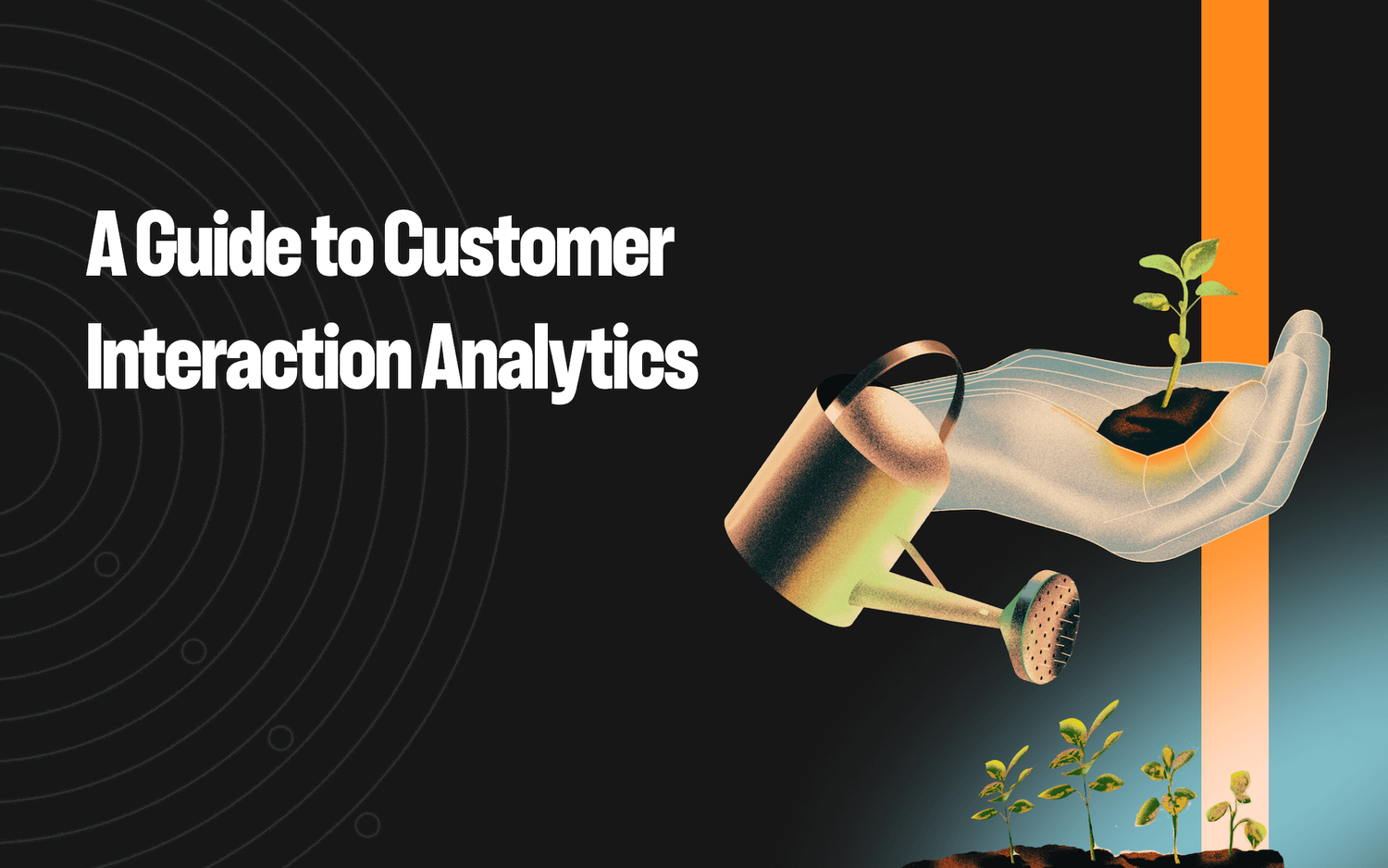
Customer interaction analytics collects and analyzes customer conversations across various channels — phone calls, emails, or text chats — to provide insights into what customers are saying and feeling.
Traditionally, data was gathered by manually sampling recorded conversations, a labor-intensive process limited to just 1–2% of interactions. Agent reviews, based on small, subjective samples, were often influenced by recent or memorable calls, leading to biases and gaps in understanding.
This limited view resulted in missed opportunities to proactively address customer needs and build stronger connections.
Fortunately, modern customer interaction analytics overcomes these challenges by leveraging natural language understanding (NLU) and AI to capture the full range of customer sentiment and intent.
In this guide, we explain how customer interaction analytics work and what to look for in a comprehensive solution, using examples from Level AI, our contact center QA software that analyzes 100% of customer interactions.
6 Ways Contact Centers Use Customer Interaction Analytics to Increase Customer Satisfaction and Operational Efficiency
Modern customer interaction analytics equips contact centers with deep insights into every customer interaction, providing new possibilities for improving service quality, proactively addressing customer needs, and making operations more efficient.
1. Improve the Customer Experience
Customer interaction analytics help identify recurring issues — like product problems or service bottlenecks — that lead to dissatisfaction.
This highlights root causes of problems, allowing a contact center to understand where to reduce the friction customers experience, resulting in a smoother, more positive journey.
For instance, by aggregating data from every conversation across channels, customer interaction analytics reveals common service obstacles, from long wait times to difficulties with system navigation.
These insights might suggest adjusting staffing levels or refining processes. When customers spend less time waiting and experience smoother interactions, they’re more likely to feel valued and heard.
2. Anticipate Customer Preferences by Identifying Trends
Customer interaction analytics allows customer service organizations to go beyond problem identification by recognizing common themes in customer preferences and proactively offering solutions and suggestions that resonate.
By analyzing the full range of interactions, it uncovers the broader interests and needs that may otherwise go unnoticed, equipping agents with insights to deliver personalized, value-driven recommendations.
For example, if interaction analytics reveal increased interest in fitness-related products, agents can mention relevant items during conversations to increase customer engagement and add value.
This proactive, insight-driven approach helps contact centers deepen customer relationships and build brand loyalty by delivering value that goes beyond simply responding to queries.
3. Improve Agent Performance
Through advanced speech analytics and a range of performance metrics, managers can identify the qualities that distinguish top-performing agents.
This allows them to pinpoint specific behaviors — such as empathy, attentive listening, or deep product knowledge — that contribute to positive outcomes.
Such insights can help shape targeted training programs, establishing a standard of excellence across the team.
For instance, analytics may reveal that high-performing agents frequently demonstrate empathy by paraphrasing customer issues, building a rapport that reassures customers their concerns are understood and valued.
4. Proactive Issue Resolution
By continuously monitoring interactions, customer interaction analytics helps support organizations address issues before they escalate into major problems.
It leverages historical data to identify patterns in customer complaints, allowing contact centers to anticipate and prevent recurring issues.
The system also flags when an issue requires escalation, enabling call centers to engage specialized support teams early.
For example, if customer analytics detect increased complaints after a software update, QA staff can notify the technical support team to publish troubleshooting guidance or proactively reach out to affected customers, reducing frustration and incoming customer support requests.
5. Improve Operational Efficiency
Customer interaction analytics identifies repetitive tasks and workflow bottlenecks, helping centers pinpoint opportunities for automation or reorganization to optimize agent productivity.
For instance, detecting a high volume of calls for routine tasks like address changes or password resets highlights the potential for automated solutions.
This insight enables quality assurance and operations teams to prioritize self-service options or chatbots, allowing customers to handle straightforward requests independently.
6. Targeted Training
Customer service organizations can develop targeted training programs by analyzing interactions across dimensions like empathy, clarity, and efficiency.
With its ability to track and evaluate each conversation, interaction analytics helps supervisors identify specific skill gaps for focused training, ensuring agents receive the support they need.
Furthermore, it provides data on agent performance, enabling contact centers to assess the effectiveness of each initiative. Metrics such as resolution time, customer satisfaction, and clarity scores reveal how well training programs translate to improved customer interactions.
What to Look for in a Customer Interaction Analytics Solution
Software That Recognizes What Customers Are Saying
To understand customer needs and pain points at scale (and translate these insights into actionable steps), you need software that truly comprehends what both customers and agents are saying in a conversation.
Legacy customer interaction analytics software often relies on basic techniques like keyword matching to interpret speech, but this approach is, at best, a crude indicator of meaning.
For instance, phrases like “I want a refund” are easy to interpret, but others, like “I’m hoping there’s something you can do to make this right,” can be trickier. System administrators must often input tens or even hundreds of keywords to capture a single intent, trying to account for all possible expressions of a single meaning.
However, this manual effort doesn’t always guarantee success, especially given the richness and complexity of human language. Algorithms can fail to grasp the context in which the words are expressed.
As a result, conventional approaches often fall short of fully understanding language complexity and context, missing subtle cues like tone, intent, and sentiment shifts, which are important for accurately capturing customer intentions.
This is why we recommend using an AI-enabled system for interaction analytics. Such systems typically interpret the subtle meanings of words and their context.
Artificial intelligence systems allow you to accurately understand what customers are saying across all communication channels, highlighting gaps in customer expectations about your products and services.
Additionally, these systems are highly adaptable, continuously learning and improving without the need to meticulously define rules or frequently make manual adjustments. Once set up, they require minimal intervention, usually only needing new training phrases when expanding to include additional capabilities.
Our customer service QA platform, Level AI, gathers interaction data from all customer channels in your contact center, using NLU and semantic intelligence to comprehend customer intent, sentiment, and conversational context at scale.
Below, we explain how Level AI detects intent expressed by customers and agents.
Intent and Scenarios in Level AI
Level AI’s Scenario Engine sifts through mountains of raw interaction data to classify different detected intents as scenarios. Potential scenarios could be, for instance, Refund Request, Feature Inquiry, or Pricing & Billing Question.
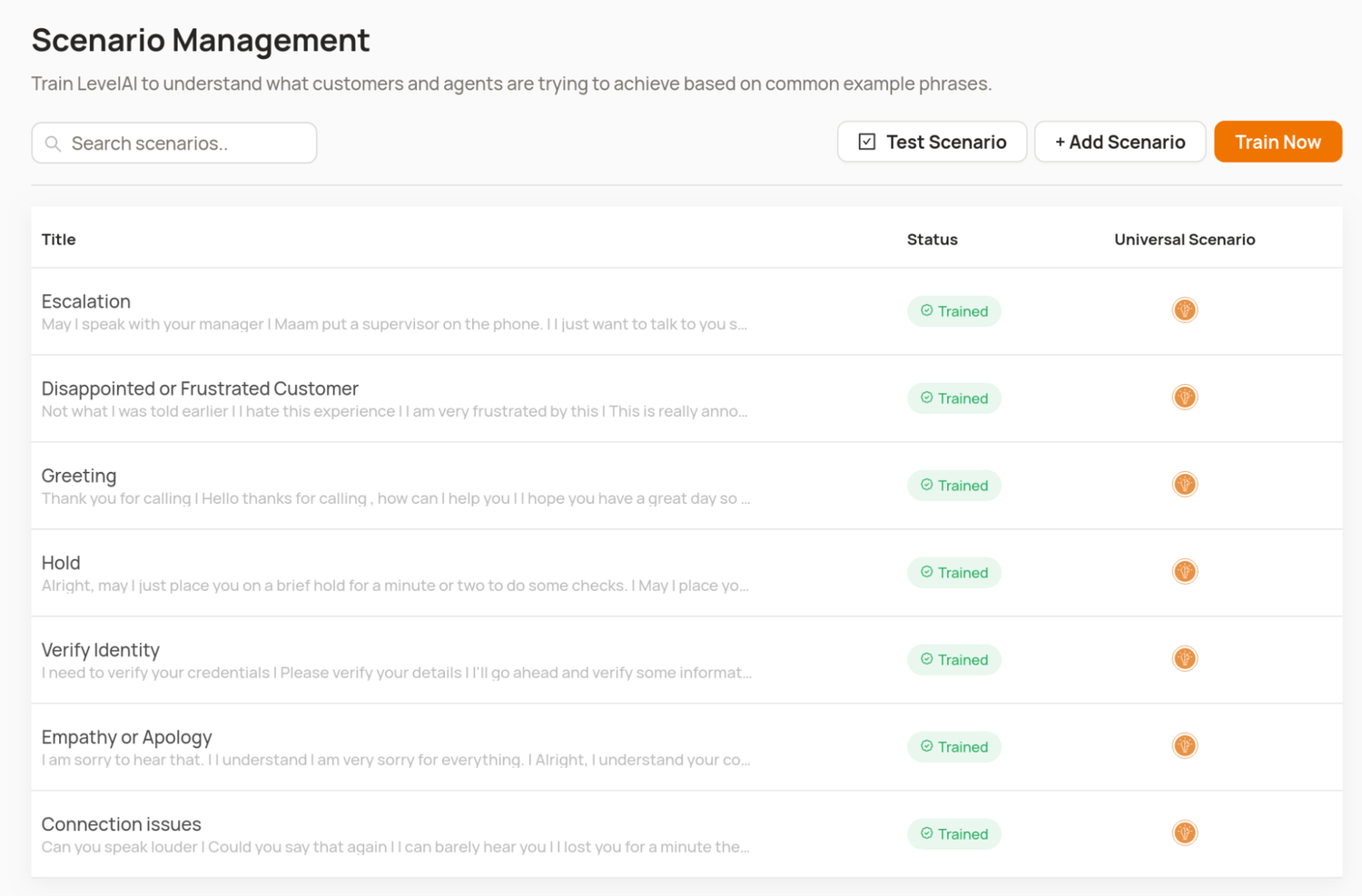
For example, if a customer mentions dissatisfaction with a recent purchase, the Scenario Engine might classify this as a Refund Request, while inquiries about how a product works would fall under a Feature Inquiry.
The system comes with many predefined scenarios but you can also create your own by providing a set of example phrases to train the AI:
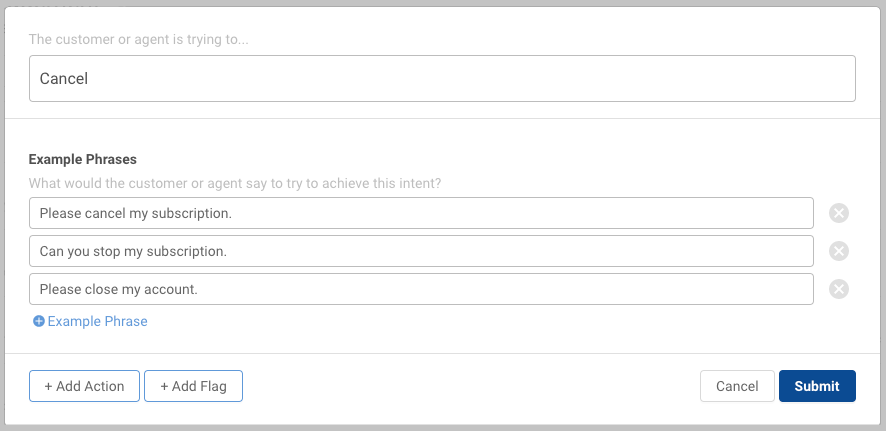
The ability to recognize scenarios is the basis for most other Level AI features that we describe in detail below.
Conversation Tags
Level AI denotes scenarios expressed throughout a recorded conversation via conversation tags. A conversation tag indicates a particular scenario and provides visibility on the underlying meanings behind what customers and agents are saying.
Conversation tags are helpful in showing you where — in a recorded conversation — particular intents appeared. They’re also searchable and filterable, allowing you to create lists of conversations containing a particular intent, such as where an agent mentioned they would follow up on an issue:
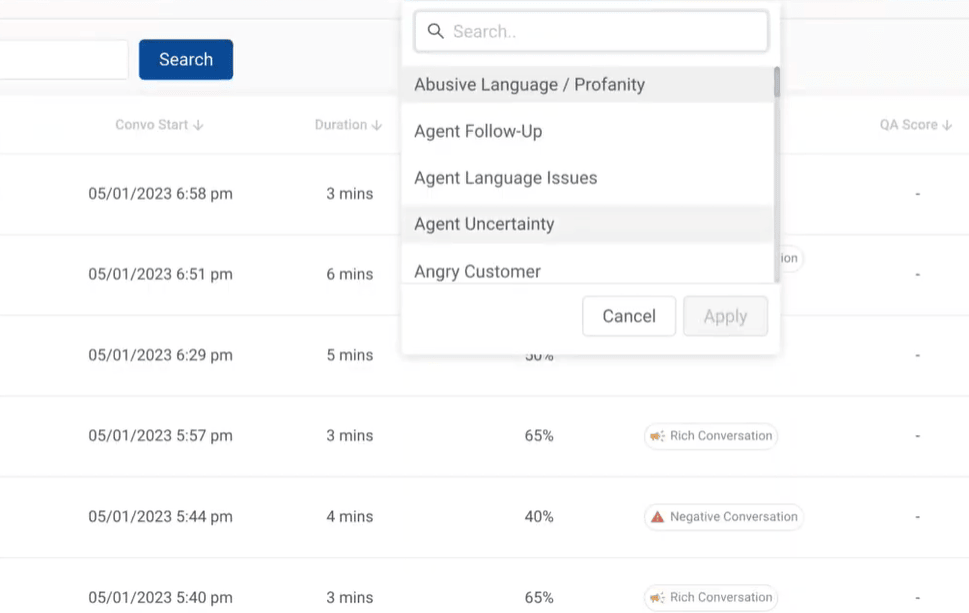
This allows you to detect patterns and common issues in interactions and decide whether to follow up with targeted training or coaching for recurring issues.
Conversation tags can be integrated into Level AI’s reporting dashboards, allowing you to analyze various dimensions against them (our reporting feature is described further below). This helps uncover trends, such as identifying which intents correlate with higher customer satisfaction or determining which customer intents agents handle most effectively.
The Emotions Behind Every Interaction are Captured
Legacy sentiment analysis classifies customer emotions as either positive or negative.
While helpful, this approach doesn’t capture the full spectrum of a person’s feelings or satisfaction, missing much of the human emotional range.
Being able to recognize all possible emotions allows businesses to respond more appropriately and anticipate customer needs more accurately.
Level AI’s Sentiment Analysis
Level AI detects the widest range of emotions across multiple channels, more than any other software in the industry, including:
- Anger
- Disapproval
- Disappointment
- Worry
- Happiness
- Admiration
- Gratitude
The platform tracks expressions of these emotions consistently across calls, chats, and emails to give an integrated view of the customer experience.
With this integrated view, Level AI can identify the emotions driving each interaction, distilling these into sentiment tags highlighting key emotional moments.
Sentiment Tags
A sentiment tag identifies a specific emotion in a conversation, and, like a conversation tag, makes it easy to pinpoint critical moments in customer interactions, whether they involve expressions of frustration, satisfaction, or excitement.
Sentiment tags are also reportable, and Level AI’s reporting feature allows you to cross-analyze emotions with factors like call topics, agent performance, and interaction outcomes.
Sentiment Score
In addition to detecting emotions, we provide an overall Sentiment Score (ranging from 0 to 10), based on a weighted average of all emotions expressed by a customer during the course of a conversation.
This score reflects both the state (negative, neutral, positive) as well as the intensity of a customer’s sentiment throughout the interaction.
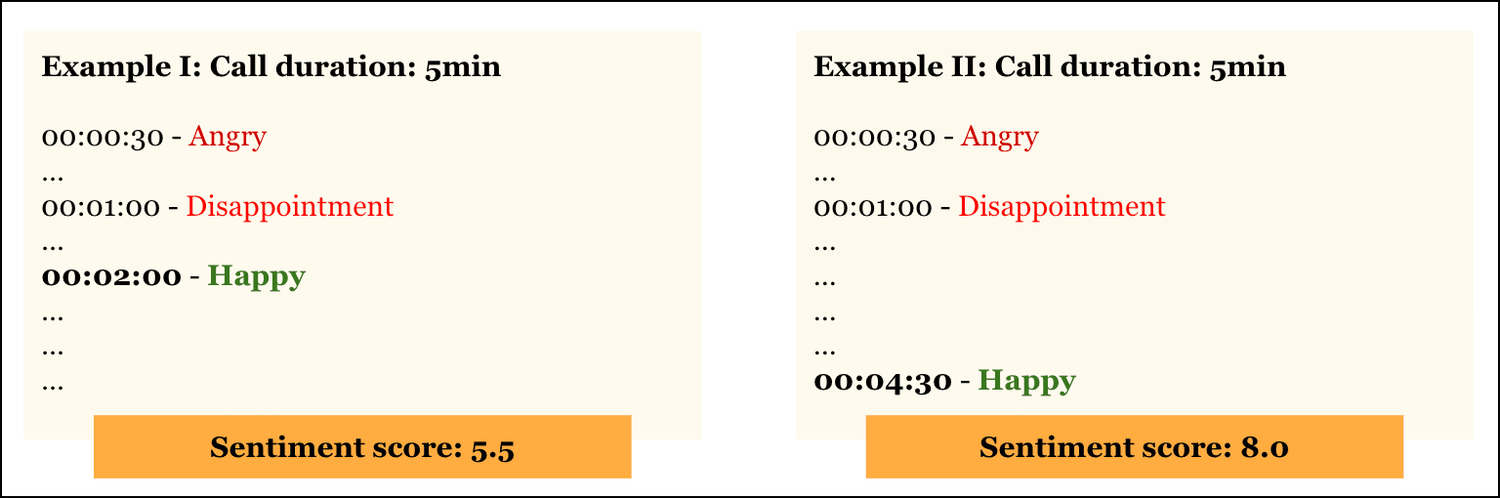
We assign greater weight to sentiments expressed at the end of a call, as these more accurately reflect a customer’s lasting impression and overall satisfaction with the interaction.
This is due to biases like the Recency Effect, where people tend to remember the final part of an experience more vividly, making those moments particularly relevant for gauging customer satisfaction and guiding future improvements in customer service.
Every Agent Interaction Is Tracked and Scored
We also recommend a solution that, thanks to an ability to grasp natural language, can detect how conversations between agents and customers unfold and rate agent performance accordingly.
Such analytics tools can auto-score the performance of all your agents across your contact center’s interactions, evaluating them based on specific parameters and highlighting areas for improvement, while also providing broader insights into team-wide performance trends.
InstaScore
Level AI lets you InstaScore agent performance against predefined rubrics, such as professional courtesy or how well the agent handled the call without unnecessary delays.
InstaScore is expressed as a percentage, reflecting how well each agent's performance aligns with your organization’s specific standards, providing a quick, quantitative measure of their effectiveness.
This at-a-glance score lets you instantly assess whether an interaction meets quality management benchmarks or if further review and coaching might be beneficial.
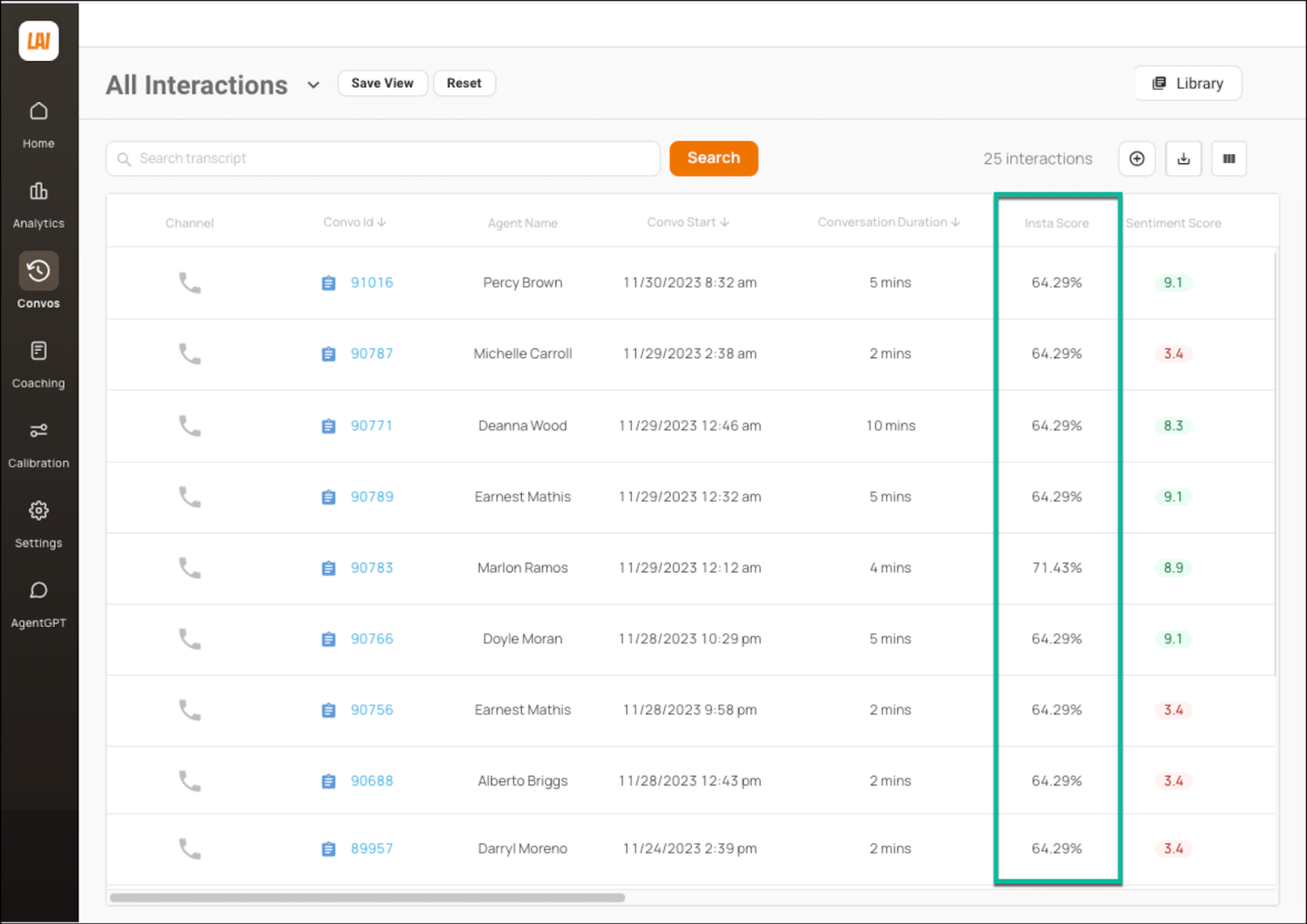
You can use InstaScore as a metric to determine whether it’s worthwhile to dig deeper into a particular conversation. This contrasts with traditional QA software, which typically offers only a few metrics, like call duration, to decide whether to spend time reviewing the entire call.
InstaReview
In addition to auto-scoring agents and conversations, Level AI tags interactions where certain negative characteristics are detected and warrant a closer look.
InstaReview tags calls that have:
- A longer-than-average duration
- A high number of assists (agent requests for assistance)
- Negative conversational moments
- Low CSAT
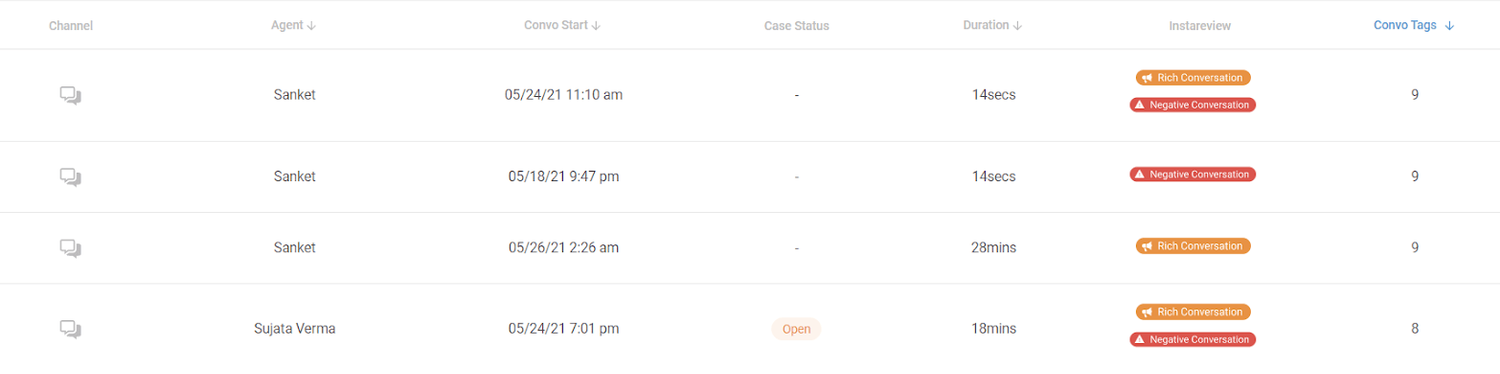
As with InstaScore, InstaReview helps you assess whether it’s worthwhile to scrutinize a call further. Both of these metrics are available in our reporting feature, allowing you to uncover deeper insights into how customer interactions went.
Monitor Calls for Smarter Decision-Making
So far we’ve focused on analyzing post-call interactions for QA purposes. However, real-time call monitoring enables managers to swiftly identify potential issues before they escalate — from signs of customer frustration to unresolved concerns.
A real-time solution continuously evaluates meaning, context, and sentiment as words are spoken, providing clear guidance on whether to intervene, offer support from the sidelines, or allow agents to continue handling the conversation.
Real-Time Manager Assist — a feature of Level AI’s Agent Assist — displays stats like InstaScore and Sentiment Score in an intuitive dashboard during live conversations:

The Real-Time Performance Dashboard aggregates key statistics for calls currently in progress across your contact center, providing a clear overview of:
- Call duration
- Potential deal size (based on the SKU price list of the products the customer is interested in)
- Probability of the customer making a purchase
- Real-time Sentiment Score and InstaScore
- Tagged Coachable Insights based on all tracked metrics
To get detailed context behind the data, you can click on any of the displayed stats. For instance, for an InstaScore, you’ll see the relevant conversation snippet and customer journey phase:
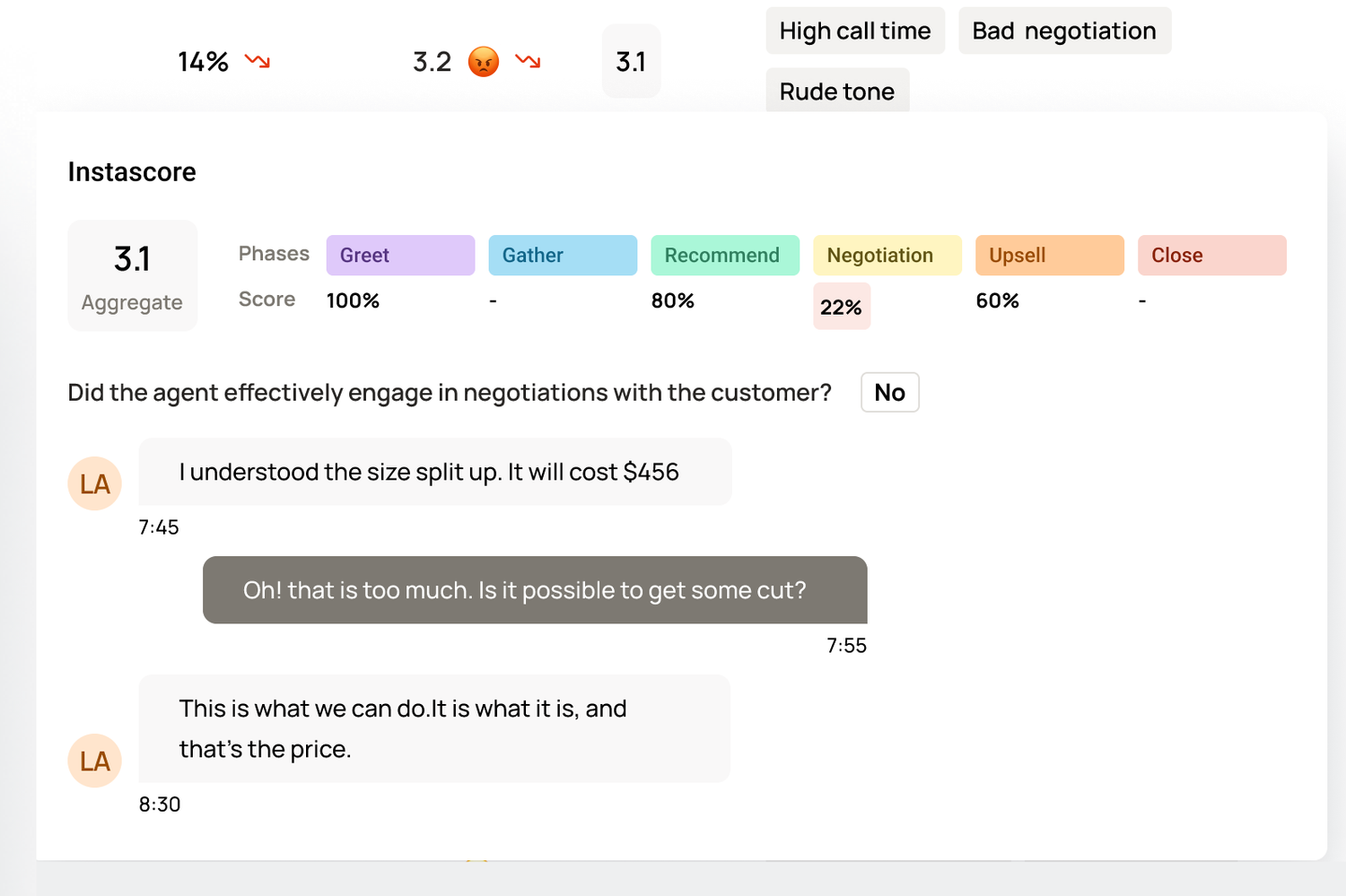
Such indicators provide a rich, layered understanding of how well a conversation is progressing, unlike traditional systems that offer limited insights, such as call duration, with minimal context.
With less context, interventions often require asking the agent to summarize the interaction, which can disrupt their flow and reduce efficiency.
For those with less time to monitor a dashboard, Real-Time Manager Assist also offers the possibility to send out alerts on Microsoft Teams or Slack when conversations have crossed some threshold, such as when a customer is churning:

The Real-Time Performance Dashboard displays Coachable Insights tags for each conversation, highlighting key features such as "High call time" and "Agent recommended a good solution."
These insights help identify areas where an agent may be struggling, offering a clearer understanding of the situation and allowing you to provide precise feedback.
Contextual Knowledge Is Delivered to Agents in Real-Time
One great benefit of using software that understands what’s being expressed is that it opens up many possibilities for increasing your call center’s productivity.
Another example is Real-Time Agent Assist, which proactively displays relevant information on agents’ screens based on the topic currently being discussed with the customer.
This solves the age-old problem of agents needing to look up information in a knowledge base while the customer waits, alleviating stress and reducing average handle times by minimizing or eliminating the need for agent multitasking.
At any given moment, the main feed of Real-Time Agent Assist shows:
- Relevant scripts and guidance
- Hints, warnings, and FAQs
- Recommended resources — retrieved from connected external sources like ticketing and knowledge systems
- AI search functionality, known as AgentGPT
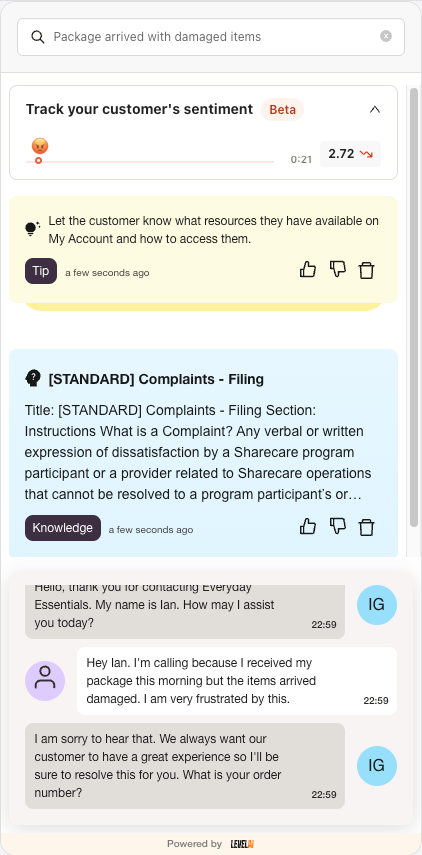
These features help boost agent productivity while allowing them to give their full attention to their customers.
The search function shown in the screenshot above is Level AI’s AgentGPT, which dynamically suggests search topics based on the conversation, speeding up the process of finding answers.
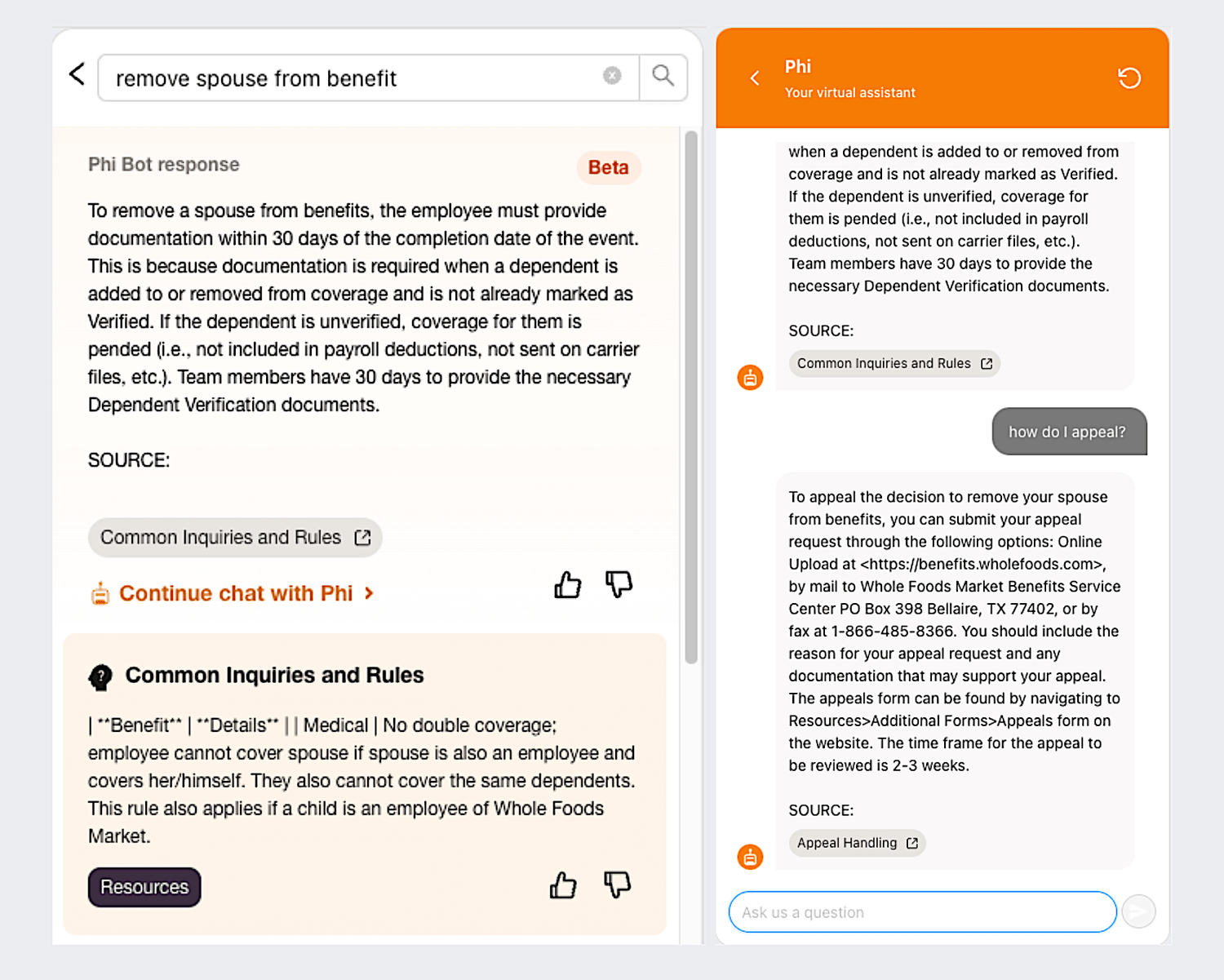
Note that agents can provide feedback to the system using thumbs up and down buttons to rate the content's relevance and accuracy, helping train the AI to provide better answers in the future.
AgentGPT also offers a generative AI chat feature, allowing agents to refine their queries for more specific and contextual answers.
Detects VoC Trends that Shape Customer Experience
The traditional method of gathering Voice of the Customer (VoC) data involves sending post-interaction surveys and hoping for high-quality responses.However, despite the effort in designing and sending these surveys, responses tend to be polarized, with only very satisfied or very disappointed customers typically responding. This often leaves out the valuable middle ground of customer experiences.
A customer interaction analytics solution should be able to recognize words and sentiments to accurately gauge a customer's feelings about your product, service, or brand, without the need for surveys.
Level AI’s VoC Insights detects patterns and trends in customer feedback across all interactions, providing stats like NPS, CSAT, and CES (without the need for surveys), while also uncovering patterns and trends in customer behavior and sentiment.
Some of these insights may have been too subtle to notice, such as customer statements about overly complicated processes for account changes, order modifications, or product returns, which can reveal friction points in the customer journey and opportunities for optimization.
Level AI displays these valuable insights in intuitive dashboards, making it easy to identify, prioritize, and address customer pain points for a smoother, more satisfying customer journey.
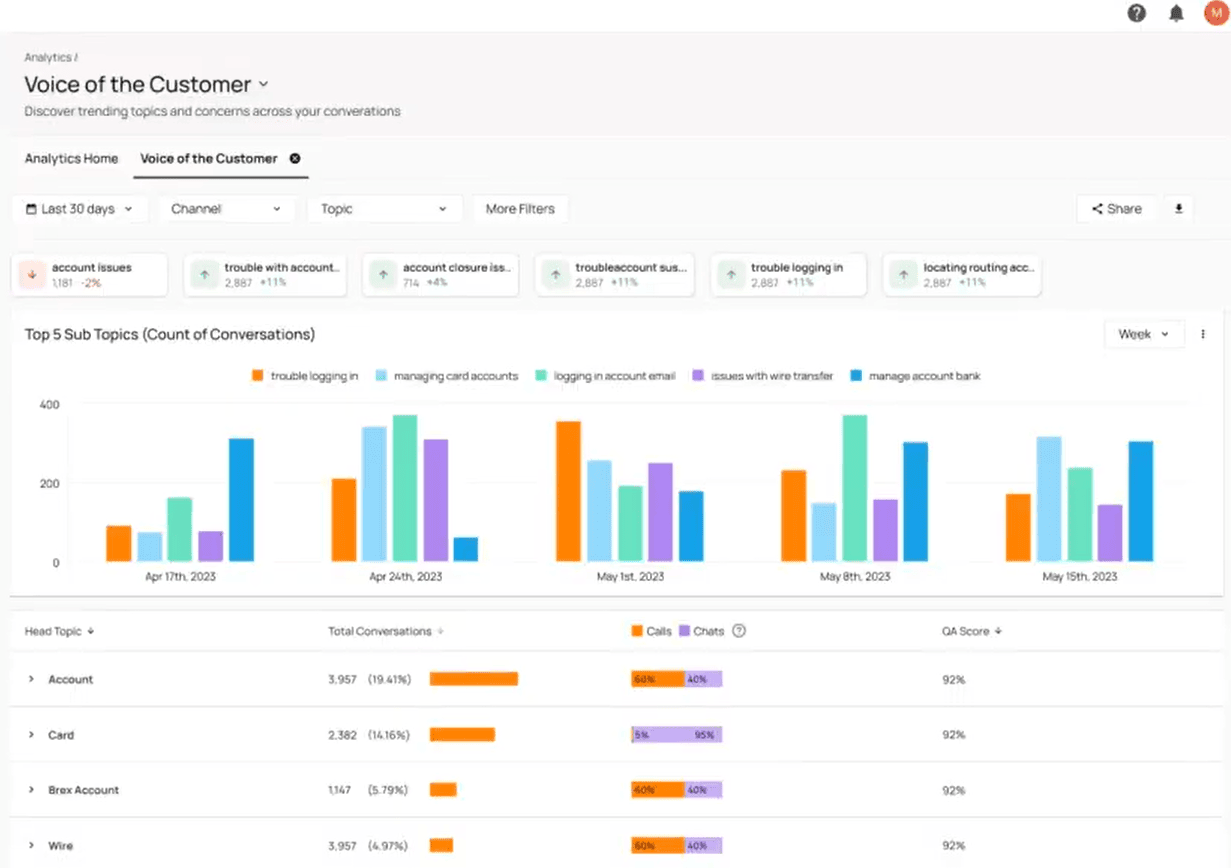
Combines Data Across Platforms for Flexible Reporting
Customer interaction analytics is only really effective if it draws upon data across many different tools, platforms, and organizations to create a unified view of customer experiences.
For instance, insights about customer complaints stored in CRM data may not correlate with observations from voice analytics, and agent performance data might be scattered across platforms, resulting in an incomplete understanding of customer satisfaction and sentiment.
Level AI allows you to pull together data from various sources in your organization to build reports that reveal comprehensive insights into customer interactions.
With its Query Builder, Level AI’s reporting analytics lets teams combine various data and file types — from Level AI and external systems like ticketing software, CRM, knowledge base, and more — to ask insightful questions about customer interaction data, such as:
- What types of inquiries or complaints lead to the longest average handle times (AHT) for agents?
- What proportion of calls involve requests for refunds or returns, and how does this impact overall customer satisfaction?
- Which conversation tags (e.g., “pricing inquiry,” “product complaint,” “feature request”) are most frequently associated with call escalations to a manager or specialized team?
- What types of issues or inquiries are resolved most efficiently through certain channels (chat vs. phone vs. email), and how does this affect customer satisfaction?
- How does an initial negative sentiment tag (e.g., “frustration,” “disappointment”) impact the likelihood of a call needing escalation?
Query Builder is easy to use and lets you create tailored reports and dashboards that consolidate actionable insights across multiple raw data sources.
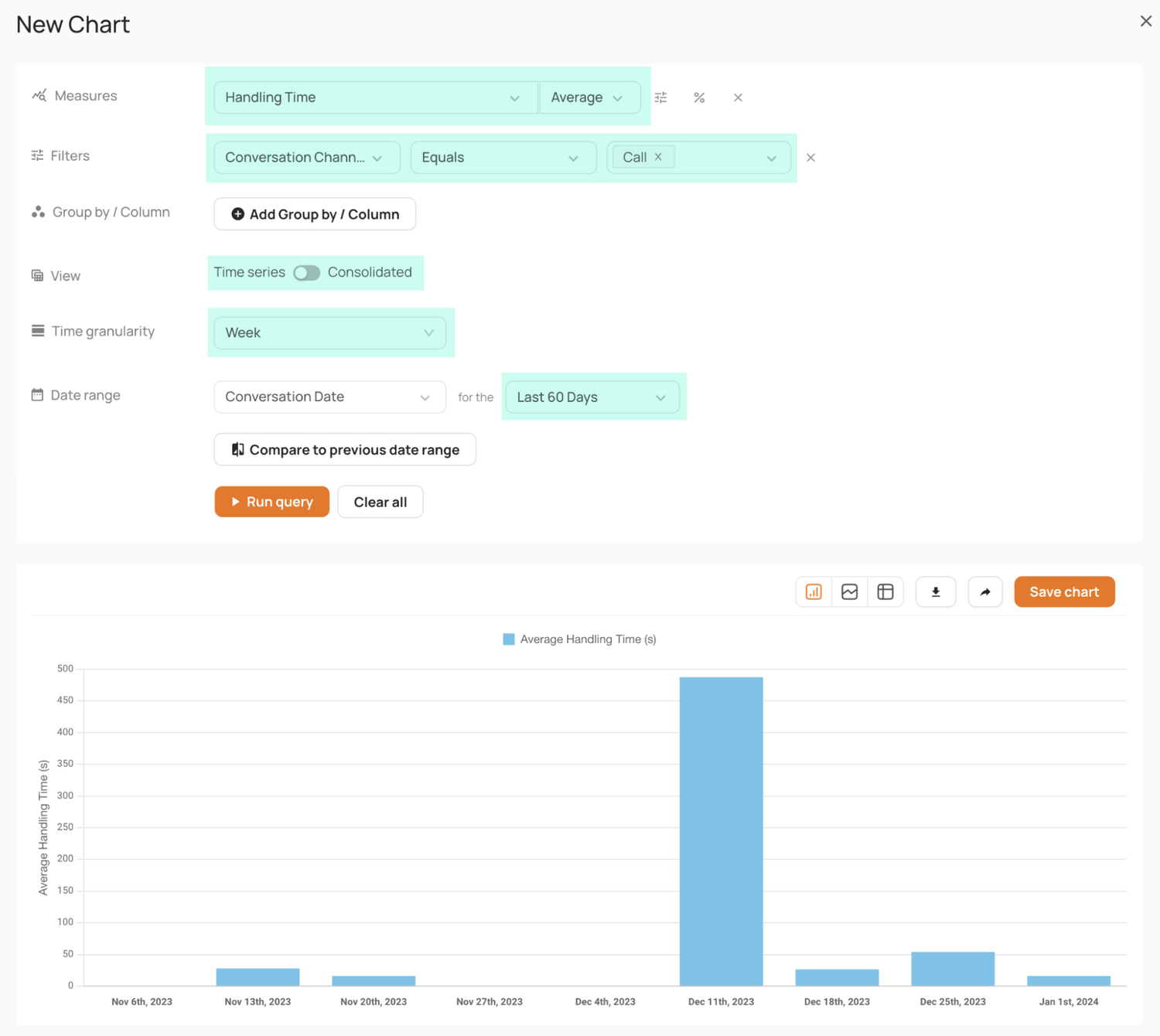
Discover Deeper Customer Insights with Level AI
Our omnichannel interaction analytics platform understands your customers’ words, capturing their sentiments and behaviors across all touchpoints. It delivers conversational intelligence by detecting sentiment shifts and escalation triggers, powered by secure, customizable generative AI capabilities.
Schedule a free demo to explore how Level AI’s customer interaction analytics can empower your team to deliver exceptional service.
Keep reading
View all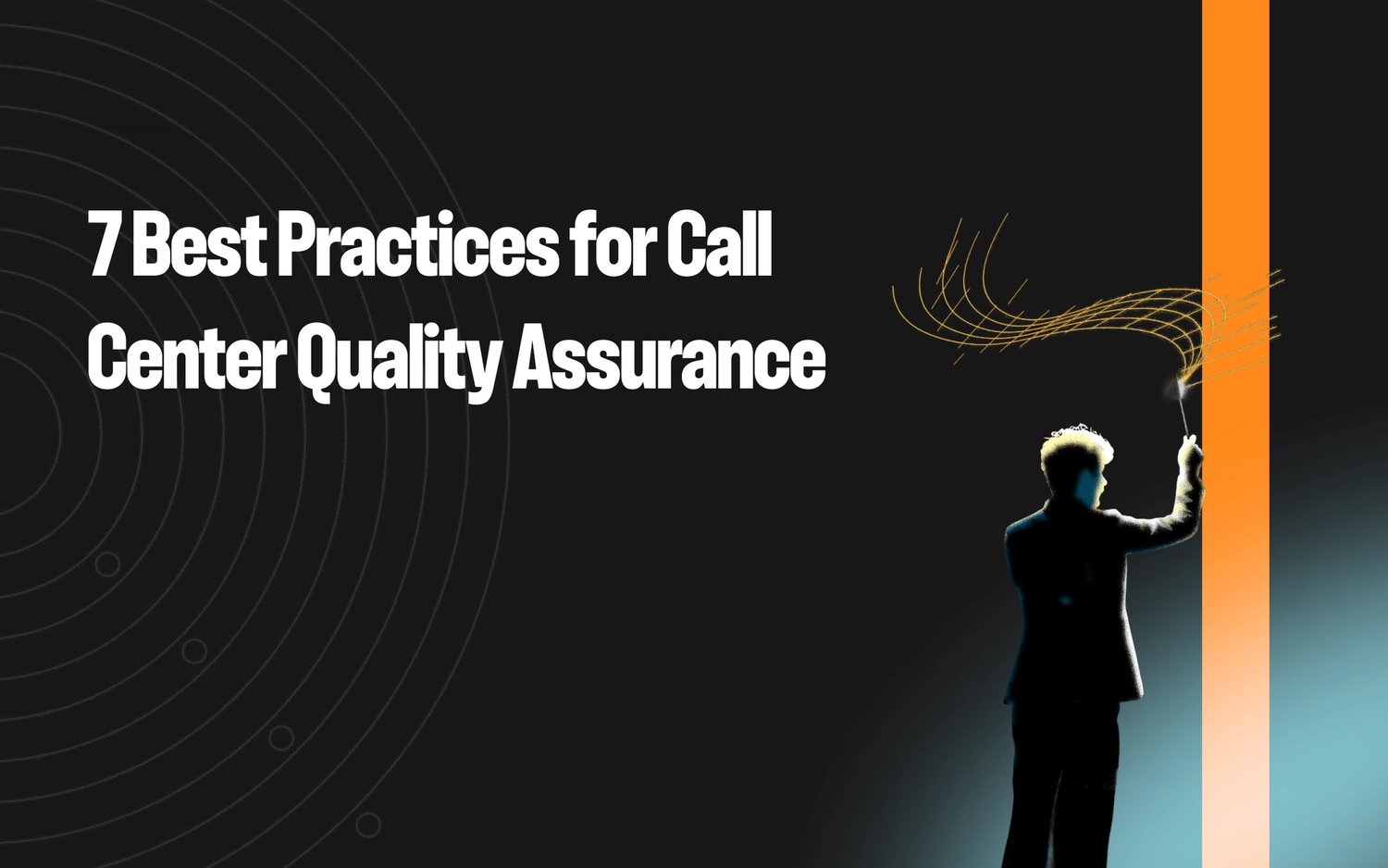
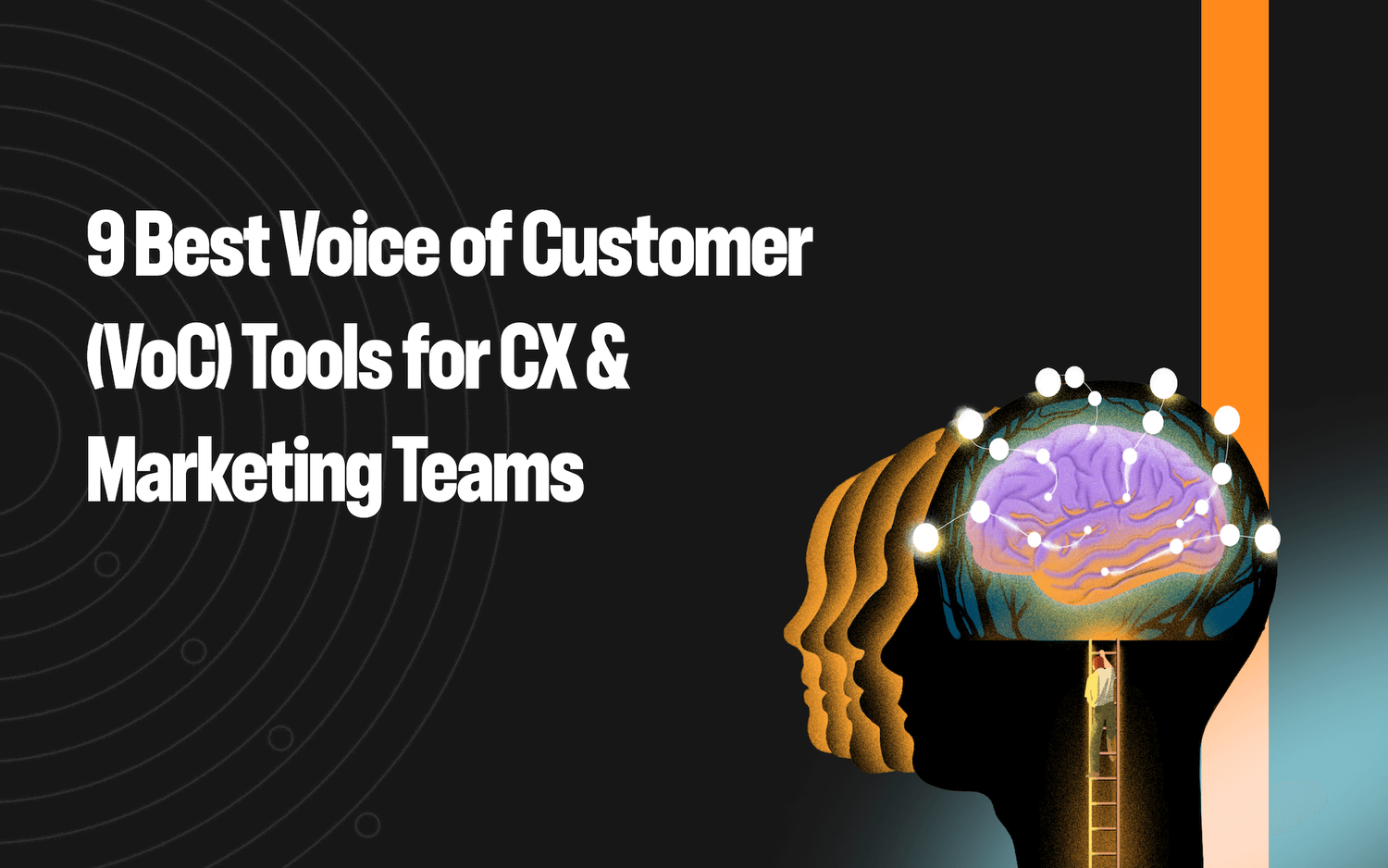
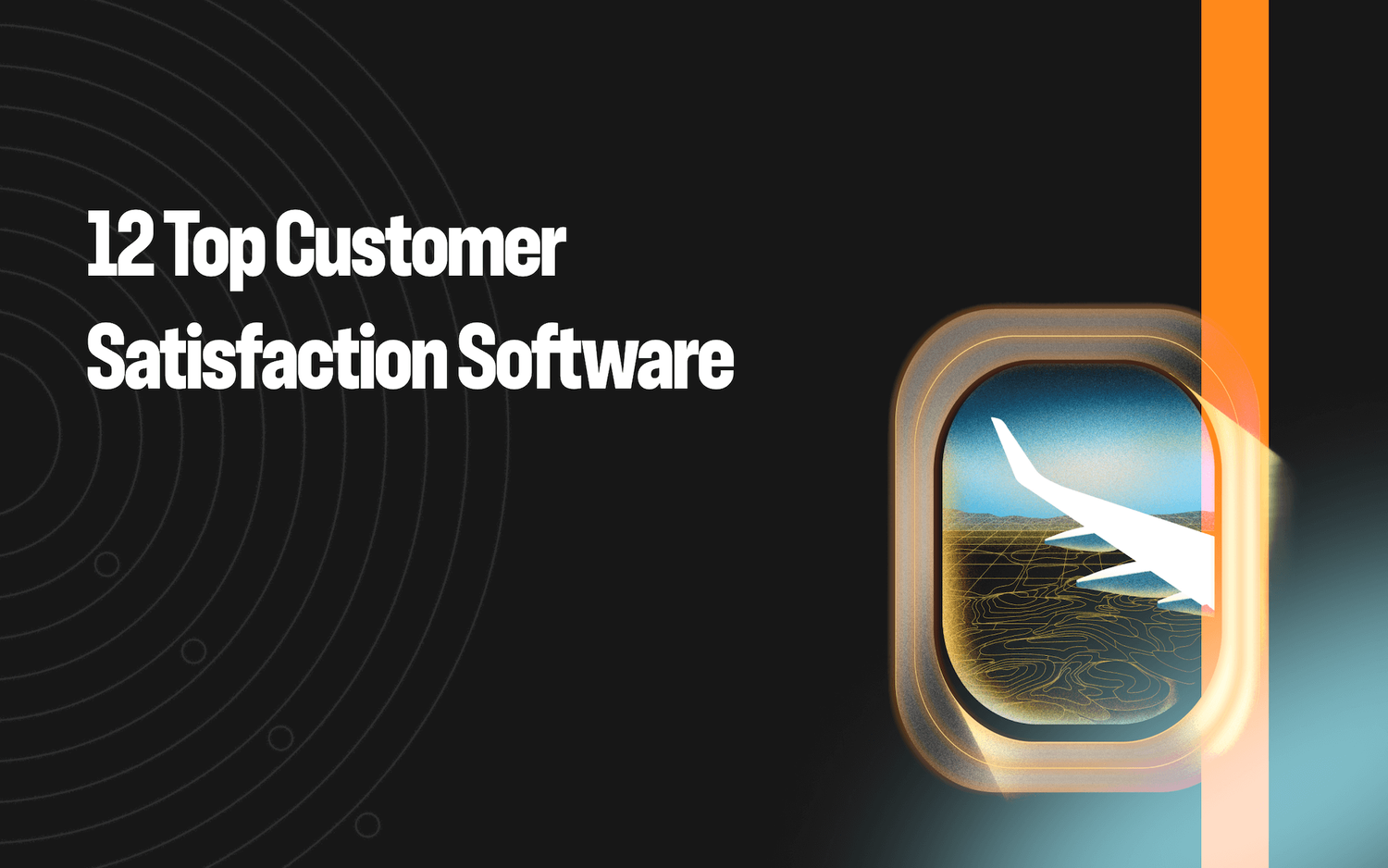