Call Center Analytics Dashboard: Examples & Why They Matter

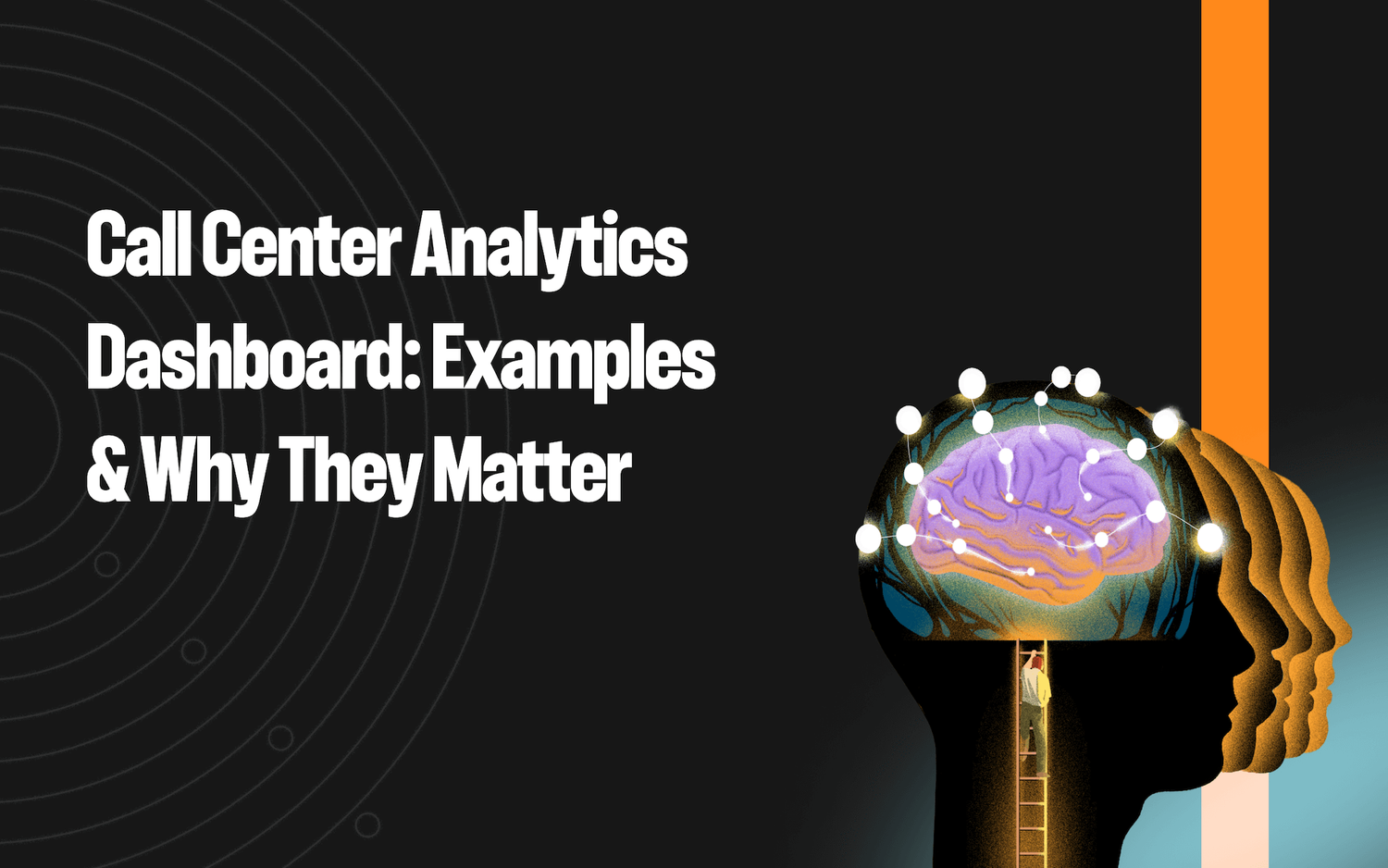
A call center analytics dashboard provides managers, QA teams, and agents with a centralized view of KPIs and other relevant metrics related to contact center operations.
It presents complex data in an intuitive, visual format by showing charts, graphs, and other representations to highlight trends, patterns, and outliers at a glance, allowing you to:
- Address issues during live interactions with real-time monitoring
- Automate reporting and reduce manual workloads
- Identify recurring issues to improve the customer experience
- Manage resources by analyzing call volume trends and identifying peak hours
- Improve coaching by identifying and addressing agent pain points
Most standard dashboards aggregate metrics and present averages. While this is useful for providing high-level overviews, they often lack the context needed to explain why these trends occur.
That’s a missed opportunity, since AI technology offers a scale and depth of analysis that uncovers hidden patterns, interprets customer sentiment, and identifies root causes of performance gaps.
We dive into the specifics of call center dashboards, explain what to look for, and highlight the effectiveness of AI-driven analytics to improve efficiency and overall customer experience.
We also show you AI dashboard examples from Level AI, our platform with secure and customizable Generative AI capabilities.
Standard vs. AI-Powered Dashboards
Legacy Call Center Dashboards
Traditionally, call center dashboards present useful but surface-level stats like call volume, CSAT, AHT, and call duration.
Much of this data is either gathered from post-interaction surveys, or from pulling information from call records, agent activity logs, and telephony systems.
Having this data in one place is beneficial to:
- Indicate whether or not your customers are getting a good experience.
- Show you where bottlenecks or inefficiencies are occurring.
- Identify peaks in call volumes so you can shift around resources to meet the demand.
However, it generally isn’t linked to the detailed context of conversations, such as customer sentiment, specific intents, or recurring themes that drive those outcomes.
And, it lacks the detailed qualitative insights needed to understand the underlying reasons behind customer behavior, agent performance, and operational challenges.
For example, during a particular call showing low CSAT, what emotion was the customer experiencing, and why?
You’d likely need to dig into the call to get to the reasons for the low score, but this can be time consuming, and is impractical at scale.
AI-Driven Call Center Dashboards
In contrast, dashboards driven by advanced AI combined with natural language processing (NLP) adds a contextual layer to the data revealing why metrics like AHT and CSAT fluctuate.
It takes the job of analyzing what’s behind trends off your plate so you can focus on what you do best: improving customer satisfaction and operational efficiency.
For example, an AI-driven system parses all your saved conversational data to detect recurring patterns of customer complaints, or highlight specific drivers contributing to customer churn.
By going straight to data sources to distill voice of the customer (VoC) metrics like CSAT, you’re able to skip surveys altogether. This also uncovers subtle trends like customer mentions of recurring product issues, knowledge gaps in agent responses, or opportunities for upselling based on customer interest.
Not only does it reveal the broad strokes of what’s happening in your business, it also pinpoints granular insights relating to individual conversations, like customer sentiment, agent performance, and consistent issues.
Such interaction-level granularity allows you to correlate the larger trends with individual customer experiences to take targeted actions, like refining agent training or addressing specific product issues.
8 Must-Have Capabilities for an AI-Powered Call Center Dashboard
1. Build Your Own Analytics Dashboards
Call center dashboards should be based on a unified view of data to offer accurate insights or be effective in the first place.
This means aggregating data from various sources, including call logs, ticketing systems, knowledge bases, and others, into a centralized platform for analysis.
Level AI offers integrations with most major contact center and telephony platforms, allowing you to mix and match data in interesting ways to build tailored dashboards and visualizations.
In particular, the platform’s Query Builder lets you easily import data from a multitude of sources that are both internal to Level AI — like conversation tags, InstaScores, and AHT — and outside of it, for instance, from your CRM, survey tool, and ticket system:
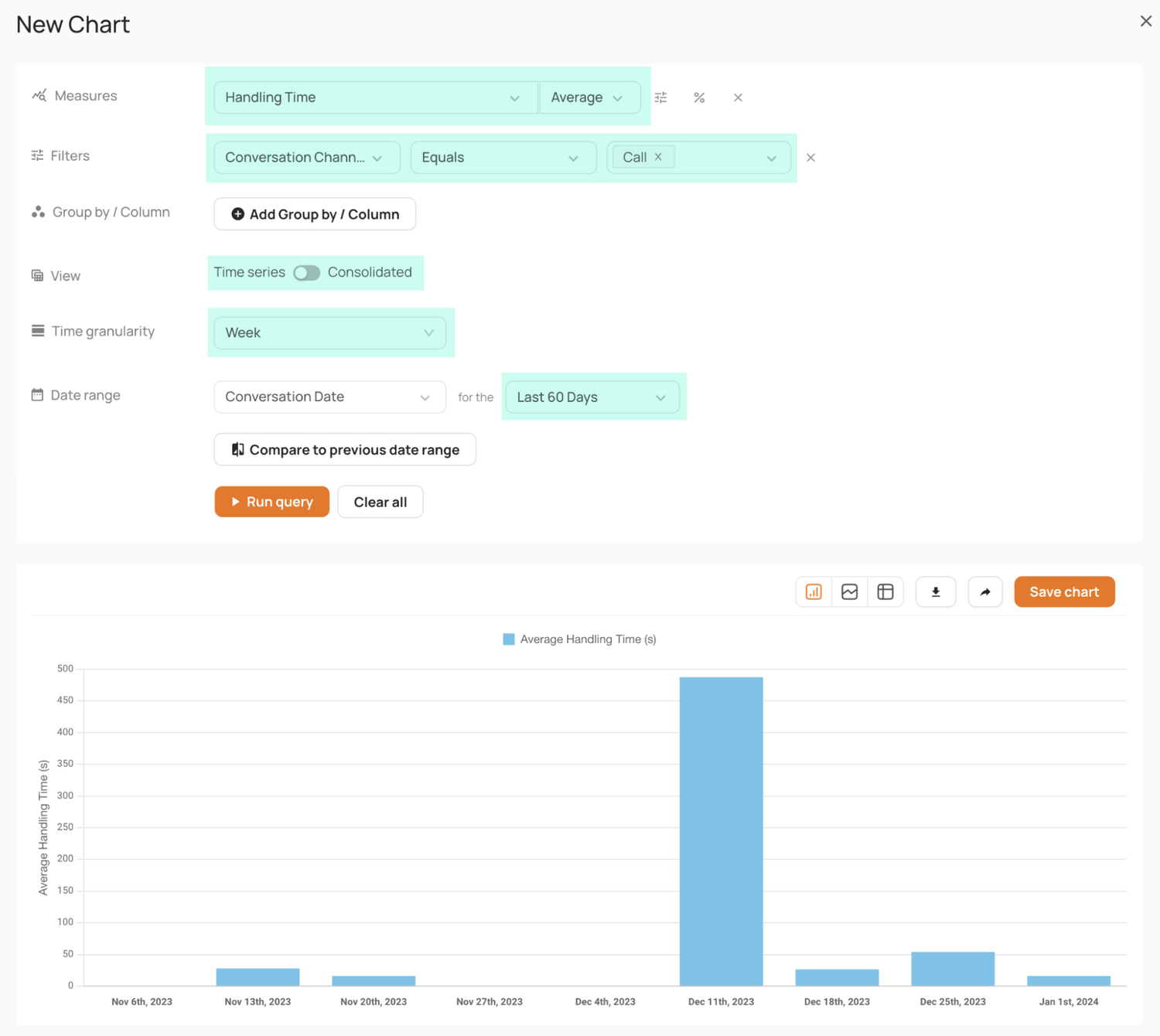
Combining data from these different sources lets you ask insightful questions such as:
- How does the presence of specific competitor mentions during calls correlate with follow-up actions logged in the CRM?
- What trends emerge when comparing sentiment shifts (e.g., sentiment scores changing from neutral to negative) with ticket resolution rates?
- How do agents’ usage of empathy-related phrases impact the likelihood of first call resolution?
- What patterns appear in customer feedback when comparing the average call response time (or average wait time) to specific customer sentiments expressed during calls?
The resulting dashboards you create are actionable and customized to your specific needs.
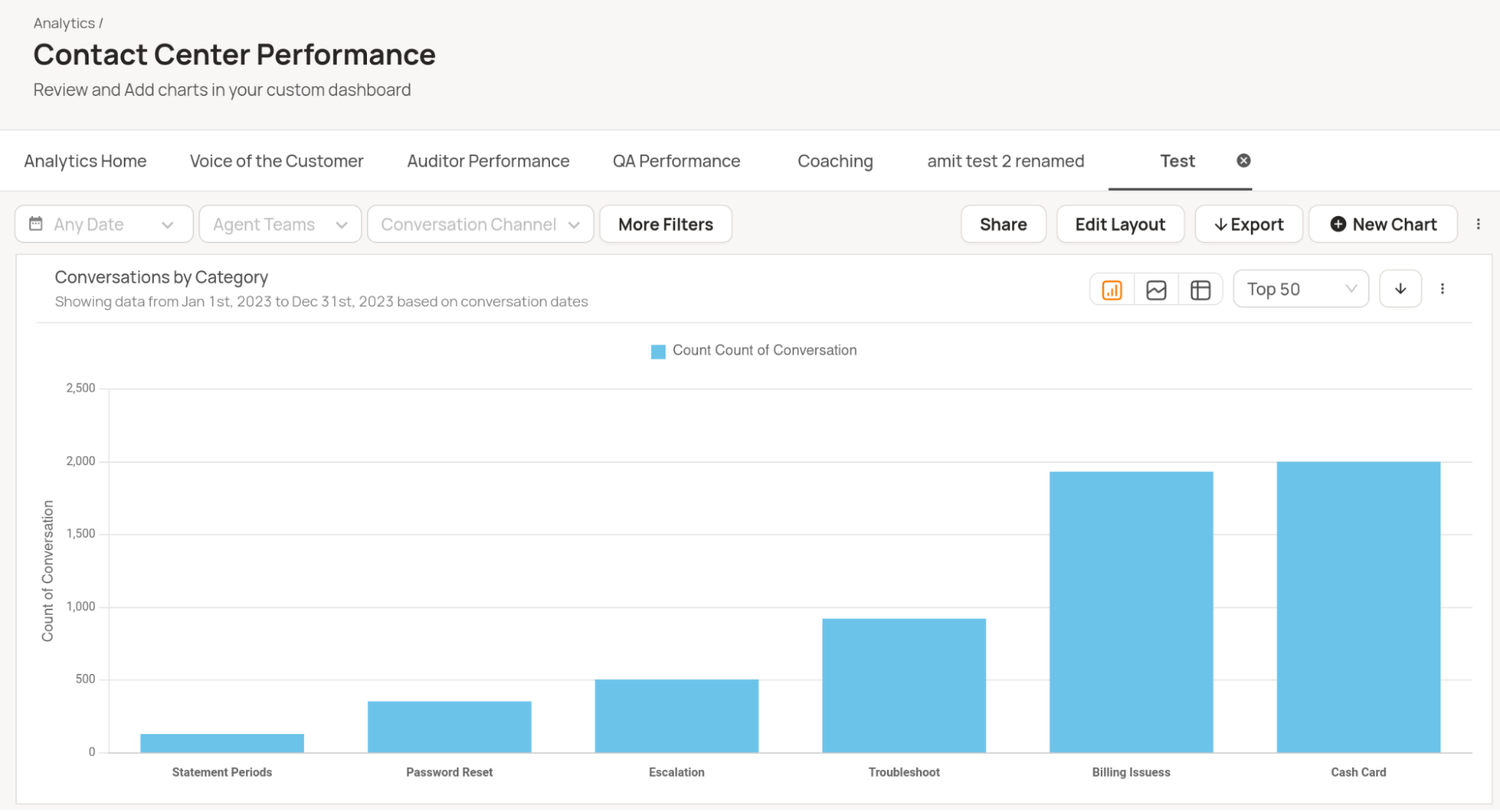
2. Identify Call Drivers Based on True Intent, Not Keywords
A dashboard that’s powered by AI comprehends word meanings and uncovers customers’ expressed concerns, indicating the reasons why customers are making contact with your organization.
Such a platform identifies and classifies customer needs based on conversation context, interpreting the subtleties of conversations, though many platforms using natural language processing (NLP) rely on keyword matching to interpret what’s being said.
Though keyword-based systems can detect specific terms, they often fail to capture the full context or subtly expressed intent of a conversation, leading to missed opportunities to understand true customer needs and sentiment.
Imagine a customer saying, “I don’t know how to assemble this [furniture],” but actually meaning, “Can you help me put this together?”
Keyword detection might misclassify such a statement as a general inquiry or complaint, rather than identify the underlying need for assembly guidance.
You can set up a system to detect most expressions of intent, but this requires entering numerous combinations of keywords in the system. This is both time consuming and prone to gaps in coverage, as it cannot anticipate all the ways that customers might phrase their concerns.
Level AI uses natural language understanding (NLU) and generative AI to overcome these limitations and ensure the system comprehends speech with near-human accuracy.
The Scenario Engine recognizes conversational moments and classifies intent as scenarios. A typical scenario might be “Refund Request” when a customer says, “I’d like my money back,” or “This isn’t what I expected.”
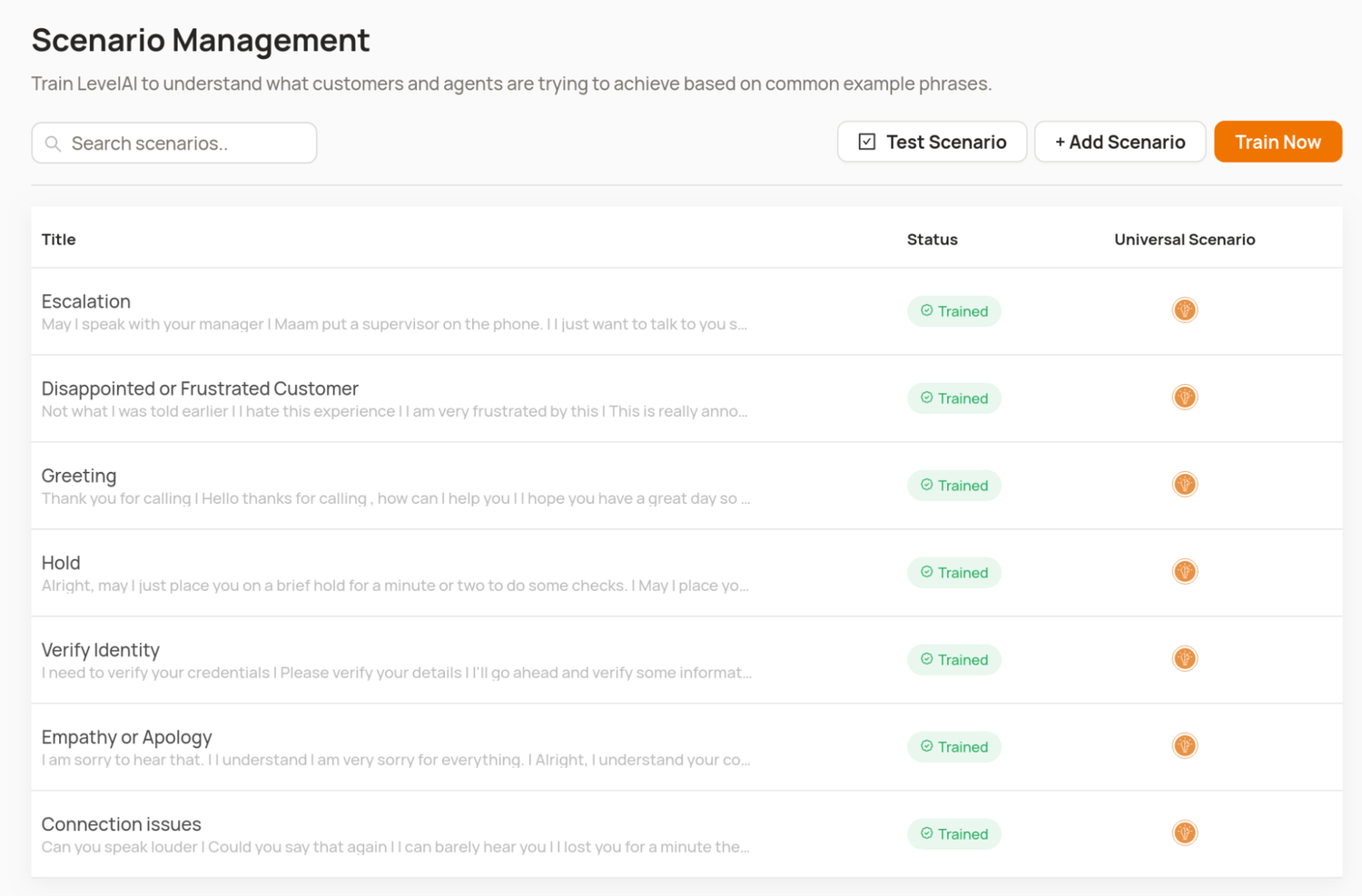
Level AI comes with a number of preconfigured scenarios, but you can define your own that are specific to your business by providing a set of seed phrases to train the AI:
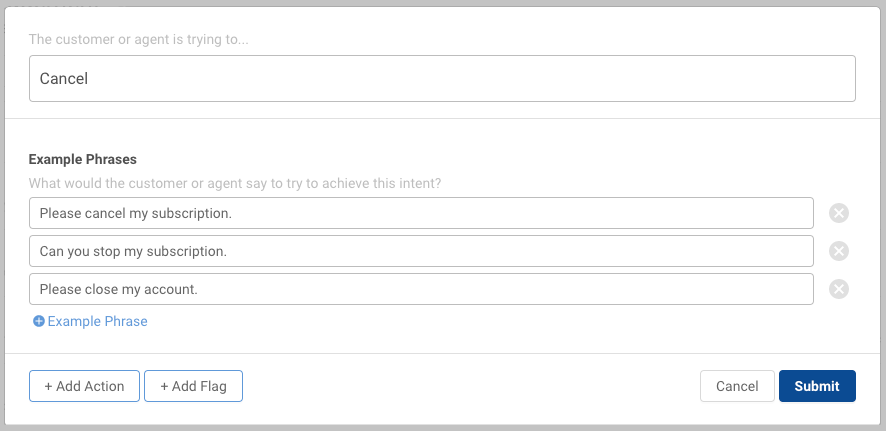
The platform also uses conversation tags to denote specific instances of intent in conversations.
For example: “I need my tracking number” might denote a scenario of “Requesting Shipping Information,” and a conversation tag would indicate where in the transcript this scenario is expressed.
Conversation tags are filterable and searchable in the system, allowing you to pull up lists of conversations curated according to a particular intent:
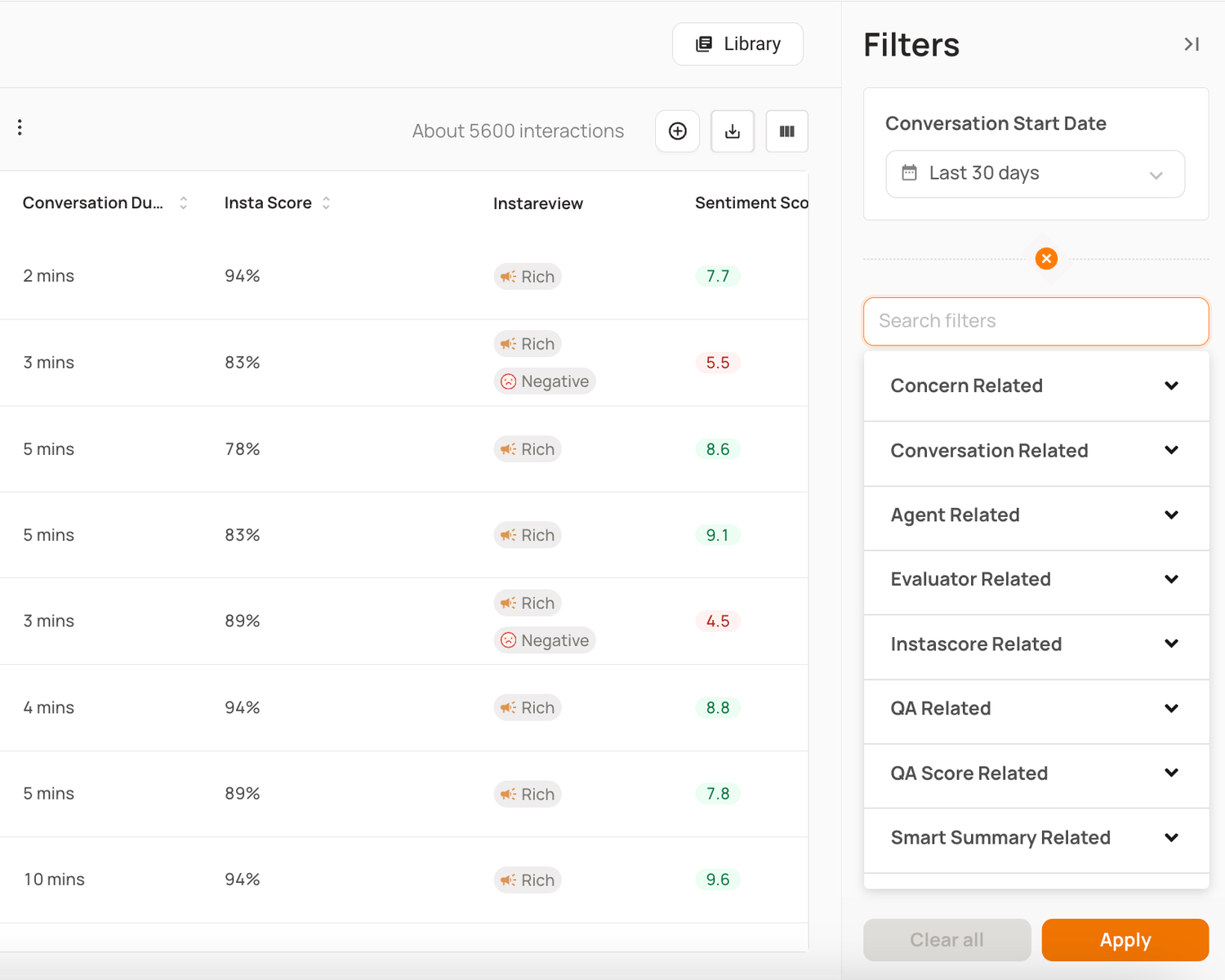
You can also report other metrics against conversation tags in Level AI’s reporting feature (see below) to correlate topics discussed with customer satisfaction, call resolution times, and more.
3. Automatically Summarize & Categorize Conversations
Next, look for dashboard features that display at-a-glance and concisely generated summaries of conversations, as well as resolution status. This lets you quickly track progress and identify areas for improvement in your quality assurance — without sifting through lengthy transcripts or raw data.
Our platform’s AI-powered Smart Summary auto-generates a concise snapshot of an interaction that includes:
- The reason why the customer contacted your organization
- The agent’s steps to resolve the matter
- Resolution status
- Any follow-up actions that were taken
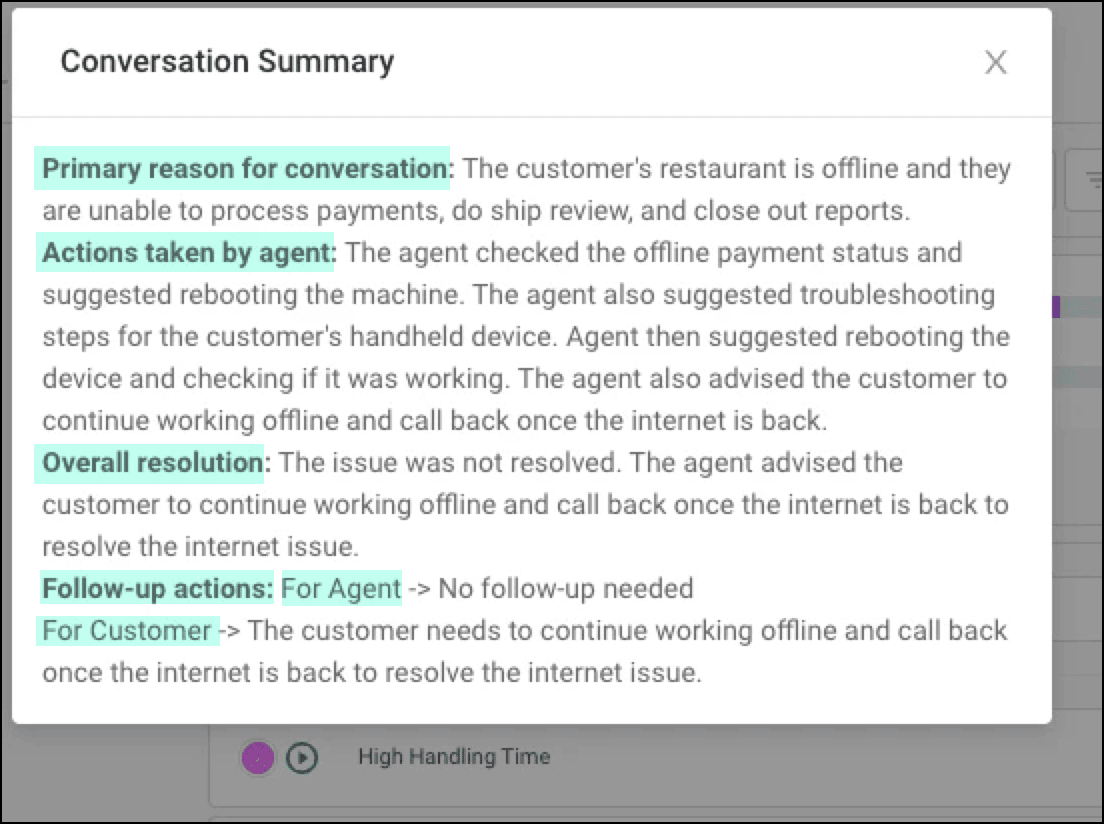
A dashboard that also classifies past interactions for automatic dispositioning saves your agents time and effort from having to manually categorize conversations, letting them focus on personalized customer support and other important tasks.
Level AI categorizes all conversations for you, assigning both a category and subcategory to each interaction to ensure accurate and consistent dispositioning:

The system automatically comes with a number of relevant categories, but your department can also define specific business-specific categories, tailoring the classification system to align with your unique workflows, priorities, and reporting needs.
To do this, you provide a few training terms for each category; the system then suggests some of its own (gleaned from the conversation history) for you to accept or reject.
Such terms presented by the system will either optimally match your category or be “near misses.” Near-miss terms are those that are contextually similar but don’t perfectly align with the intended category, allowing you to further refine the semantic intelligence model by accepting or rejecting them.
This iterative training ensures the model becomes increasingly more accurate and tailored to your organization’s specific needs over time.
4. Uncover and Classify a Wide Range of Customer Sentiments
Many speech analytics systems offer sentiment analysis dashboards but many classify customer emotions as either positive or negative.
While this is better than no sentiment indicator at all, it fails to capture the full range of emotions and underlying context driving customer interactions.
Other systems use voice analytics to measure caller emotions, but these can be prone to error, for example, misinterpreting changes in pitch and tone as agitation, when these shifts may actually indicate excitement or nervousness.
Level AI identifies emotions and can distinguish among seven different types, the highest number of any software in our category:
- Gratitude
- Happiness
- Admiration
- Worry
- Disapproval
- Disappointment
- Anger
This offers a more differentiated and granular understanding than any binary classification, enabling teams to better respond with empathy and precision.
The system also uses sentiment tags to identify and classify instances of expressed emotion in conversations. These tags are searchable and filterable just like conversation tags, allowing you to create curated lists of conversations where certain emotions are detected.
You can add specific tags to your reporting to correlate customer emotions with key metrics, such as resolution time, agent performance, specific product issues, and others.
Level AI also scores the overall customer sentiment of an individual conversation based on the aggregate of the emotions the customer had expressed, giving you a more precise idea of their experience.
This is Level AI’s sentiment score, and it allows teams to track sentiment trends over time, and identify areas for improvement. The score ranges from 0 to 10, with 0 being the most negative and 10 being the most satisfied.
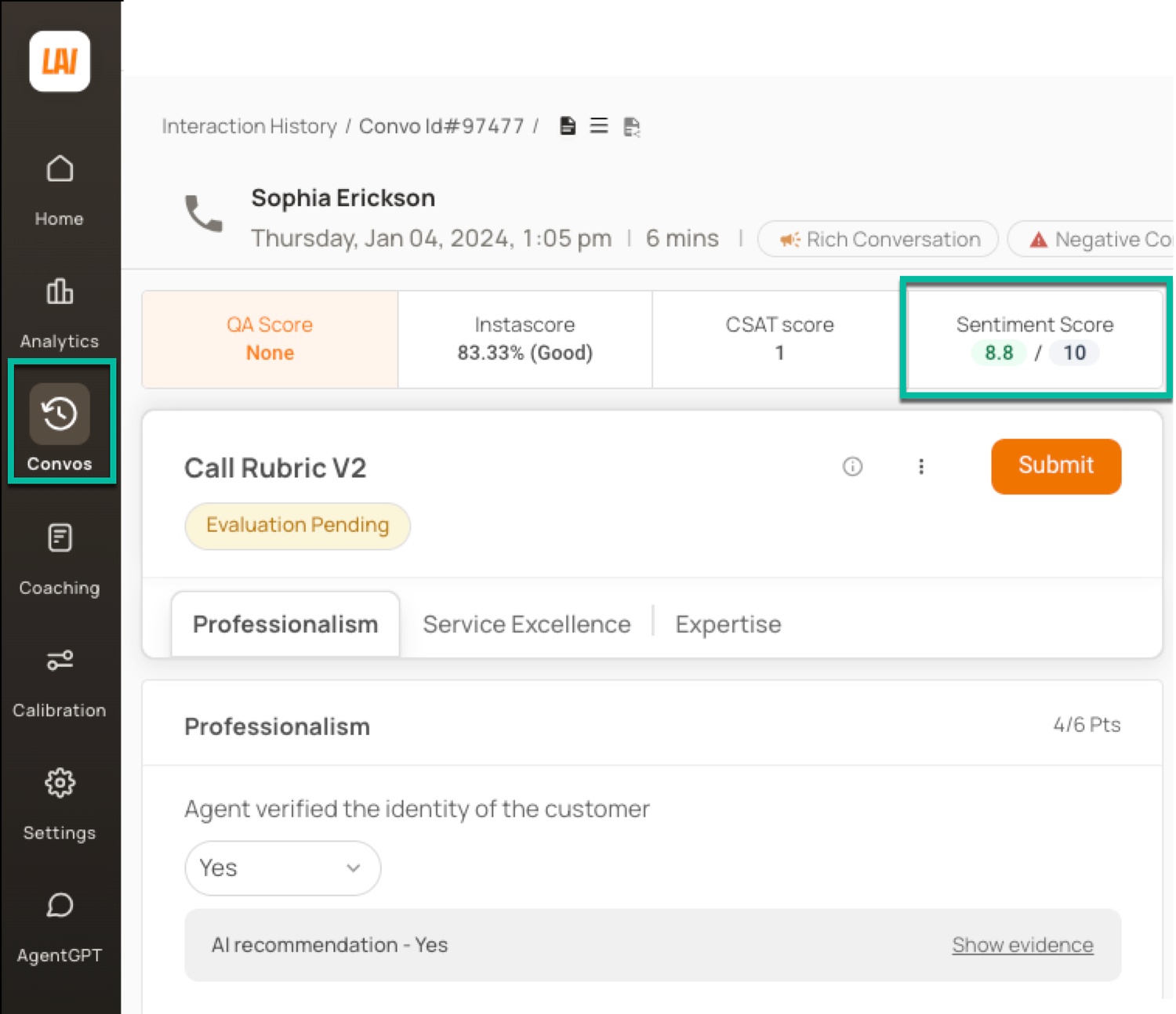
The score is based on a differential weighting of emotions according to their intensity (e.g., anger carries more negative weight than annoyance).
Emotions occurring at the end of a call are weighted more heavily than those occurring earlier since they reflect the lasting impression of the interaction (and your brand), which has a stronger influence on overall customer satisfaction and experience.
5. VoC Insights That Don’t Rely on Customer Surveys
As mentioned earlier, legacy call center dashboards display CSAT scores and other VoC metrics based on surveys sent to customers in the hope they’ll fill these in.
Unfortunately this isn’t always the case and results can be skewed towards the extremes of the spectrum (either very happy or very unhappy) while the experiences of the majority, who may have had moderate or neutral experiences, are underrepresented or entirely absent.
This makes it harder for organizations to gain an accurate and holistic understanding of their overall customer experience.
Level AI addresses the limitations of surveys by analyzing customer interactions directly, capturing insights from all conversations rather than a small, biased subset of respondents.
Thanks to NLU and generative AI, the platform detects all words and feelings expressed in 100% of conversations, ultimately capturing the statements and feelings of those in the “middle ground” who are usually less inclined to respond to surveys.
Level AI’s VoC Insights distill such findings into not only accurate customer satisfaction scores, CES, NPS, and FCR, but also show more subtle trends that may have previously not been so apparent, such as:
- Recurring frustrations with certain processes, expressed by customers as repeated mentions of delays in resolving their issues, or difficulty navigating self-service options.
- Emerging product feature requests or desires, like customers expressing interest in a missing feature that would improve their experience.
- Shifts in customer sentiment around pricing, such as increased mentions of lack of affordability or comparisons with competitor pricing.
Level AI aggregates this data into user-friendly dashboards to show at-a-glance insights into customer sentiment, trending topics, agent performance, and key patterns across all channels:
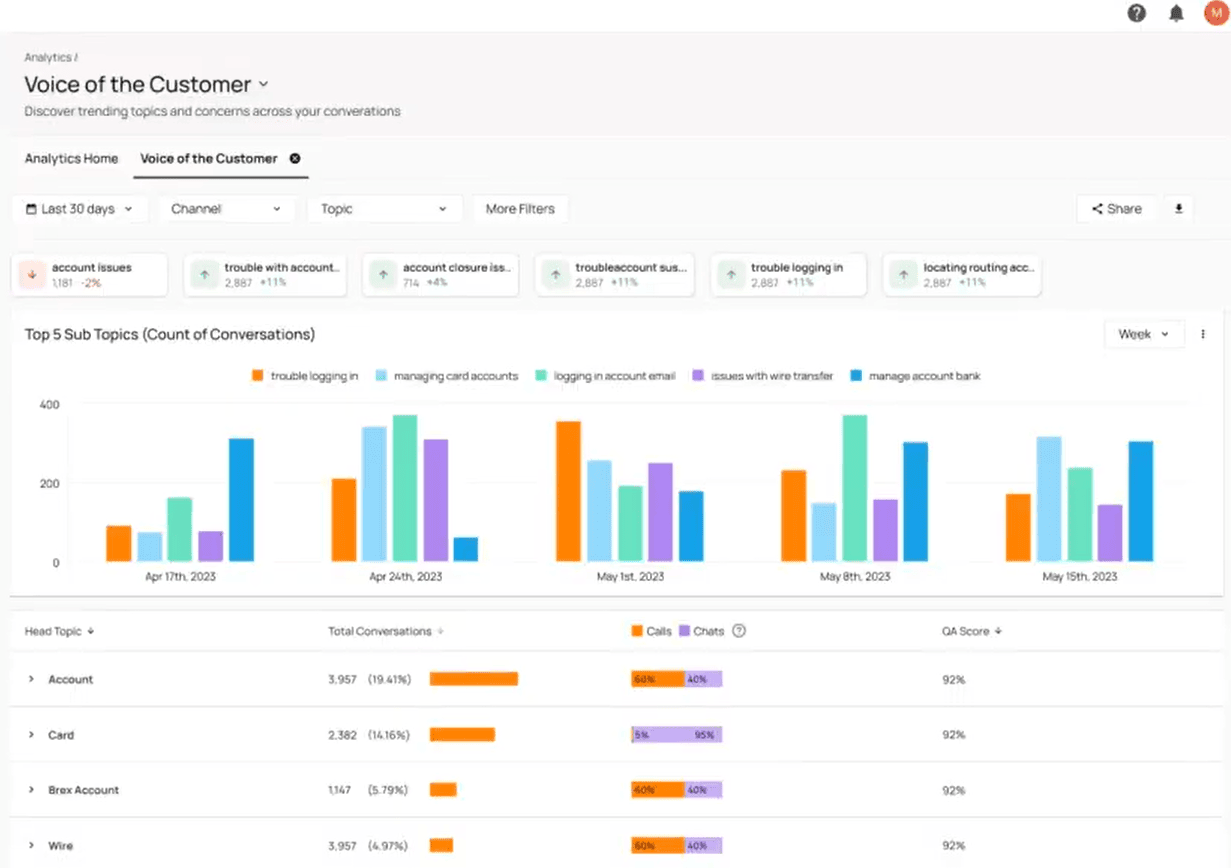
6. Auto-Score Agent Performance
The most useful performance dashboards go beyond basic call volume metrics to offer a unified view of individual and team performance, resolution effectiveness, and key drivers behind successes or challenges in meeting key performance indicators.
Dashboards should present data in clear, intuitive, and actionable ways to empower teams to make data-driven decisions regarding coaching and training, by letting them:
- Evaluate individual agent performance across a spectrum of relevant rubrics.
- Identify trends, patterns, and areas where agents excel or require additional support.
- Facilitate continuous improvement in agent performance and overall call center operations.
To simplify the evaluation process, Level AI auto-scores agent performance using a single score, InstaScore, which provides a snapshot of overall performance against predefined rubrics.
Examples of rubrics used by InstaScore include:
- Did the agent use the company’s greeting script?
- Did the agent follow company protocols for documenting the interaction?
- In case the customer’s issue wasn’t resolved, did the agent close the conversation with a summary of next steps or a follow-up plan?
The score is expressed as a single percentage reflecting how well an agent met the requirements as defined by your organization’s benchmarks.
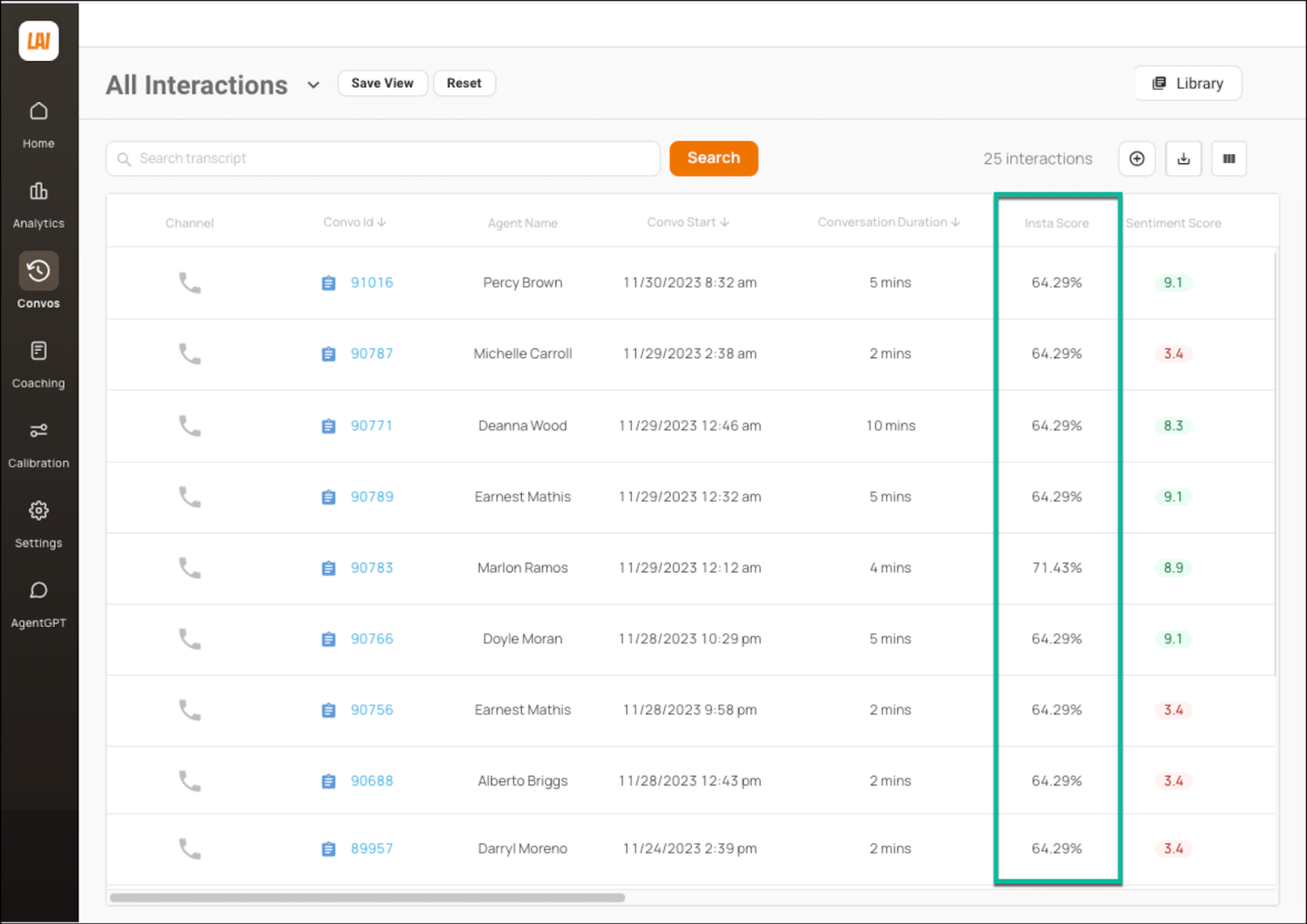
As you can see in the screenshot, an InstaScore is calculated for 100% of agent interactions and allows you to quickly sample performance metrics or flagged moments to decide whether you’d like to dig into more details of an agent’s performance.
7. Guide Agents During Live Calls
A system that understands what’s being said can assist agents on live calls by displaying contextual help when they need it.
Effective agent guidance saves them the time and effort of having to look up information while on calls — and experience the pressure of maintaining smooth interactions with the customer on the other end of the line.
Level AI’s Real-Time Agent Assist detects what customers are saying in real time and places the right information at agents’ fingertips, showing:
- Action hints, warnings, and FAQs.
- Articles and resources from connected systems like ticketing and knowledge systems.
- Agent scripts and general guidance.
As the conversation progresses, the information is continually updated and placed at the agent’s disposal, providing them with the space to focus on offering excellent customer service rather than needing to look up answers.
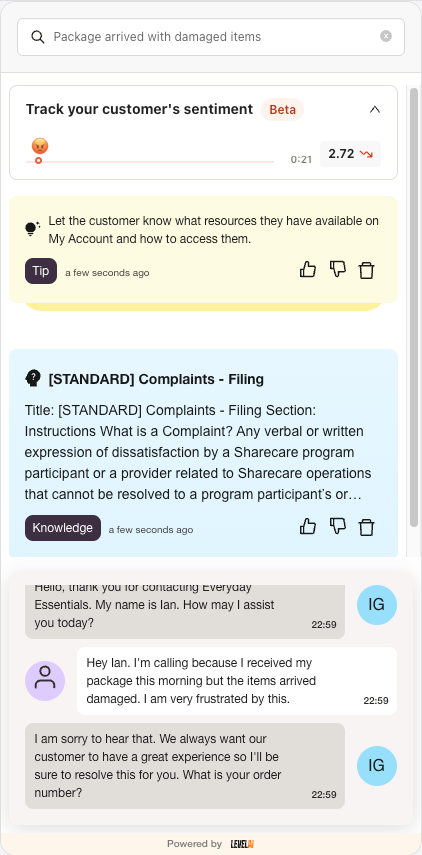
Real-Time Agent Assist’s knowledge cards also include thumbs up and down buttons for rating the information’s relevance and accuracy to train the AI to provide better and more accurate information.
The product also includes search functionality powered by AgentGPT, a generative AI model trained on your organization’s data, which suggests search queries and topics that are relevant to the topic under discussion.
This interface allows call center agents to engage in a conversational dialogue with their connected knowledge base, and also to refine searches, ask follow-up questions, and retrieve precise answers tailored to each customer interaction:
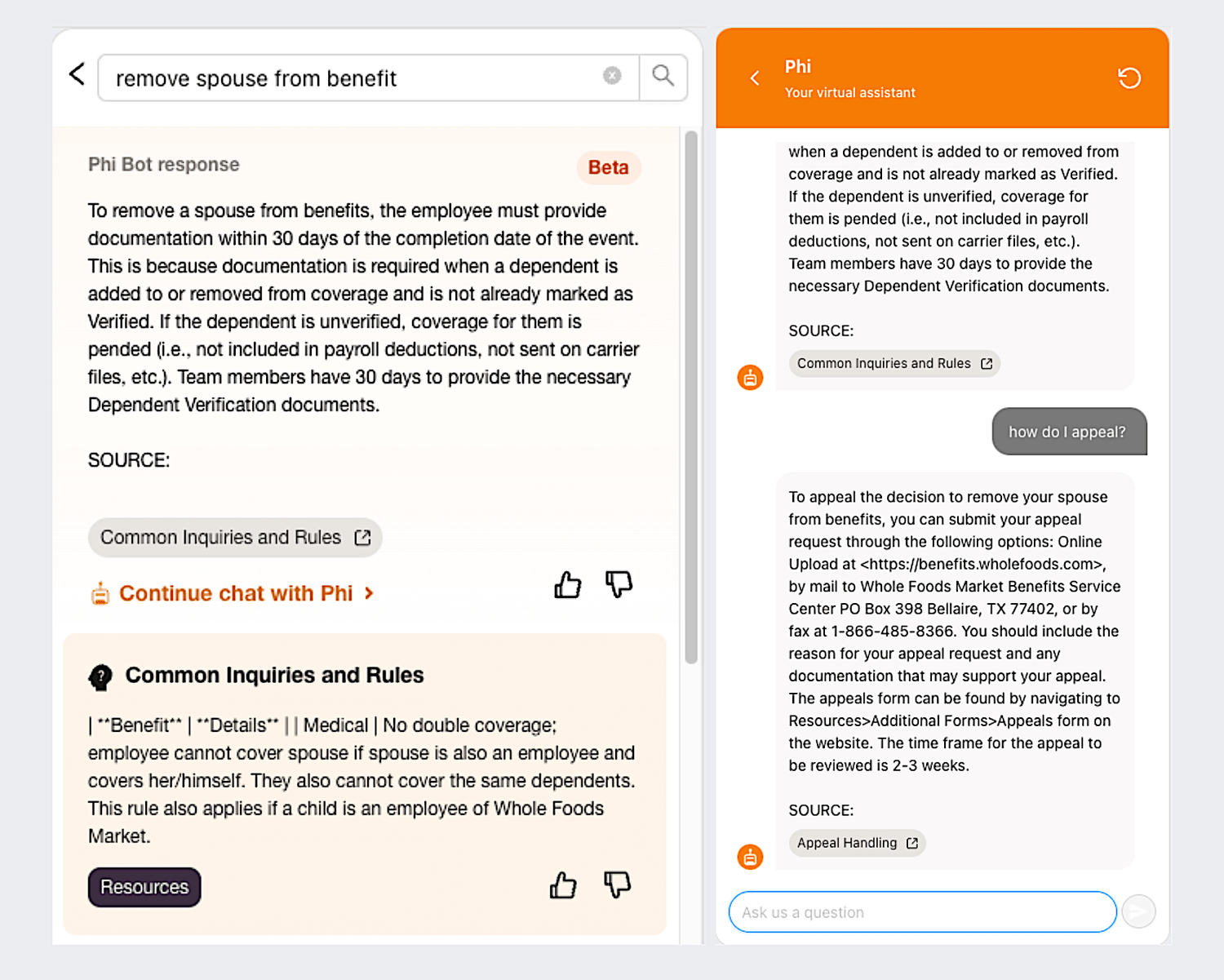
Customers using Real-Time Agent Assist are seeing significant results, such as a 13% drop in average handle time.
8. Monitor and Get Insights on Ongoing Conversations
Dashboards showing real-time KPIs and transcripts for ongoing calls allow call center managers to monitor conversations and identify issues before they become problems.
Real-Time Manager Assist provides an overview of key stats of live conversations, giving you a snapshot of interactions to help you prioritize which calls to intervene on:

The dashboard shows stats such as:
- Agent performance (InstaScore)
- Customer sentiment (sentiment score)
- Key characteristics of the call (Coachable Insights such as “agent recommended a good solution”)
- Current call duration
- Probability of a customer’s conversion resulting from an upsell
Clicking on any of the stats displays further details behind it, such as this screen shown for agent performance:
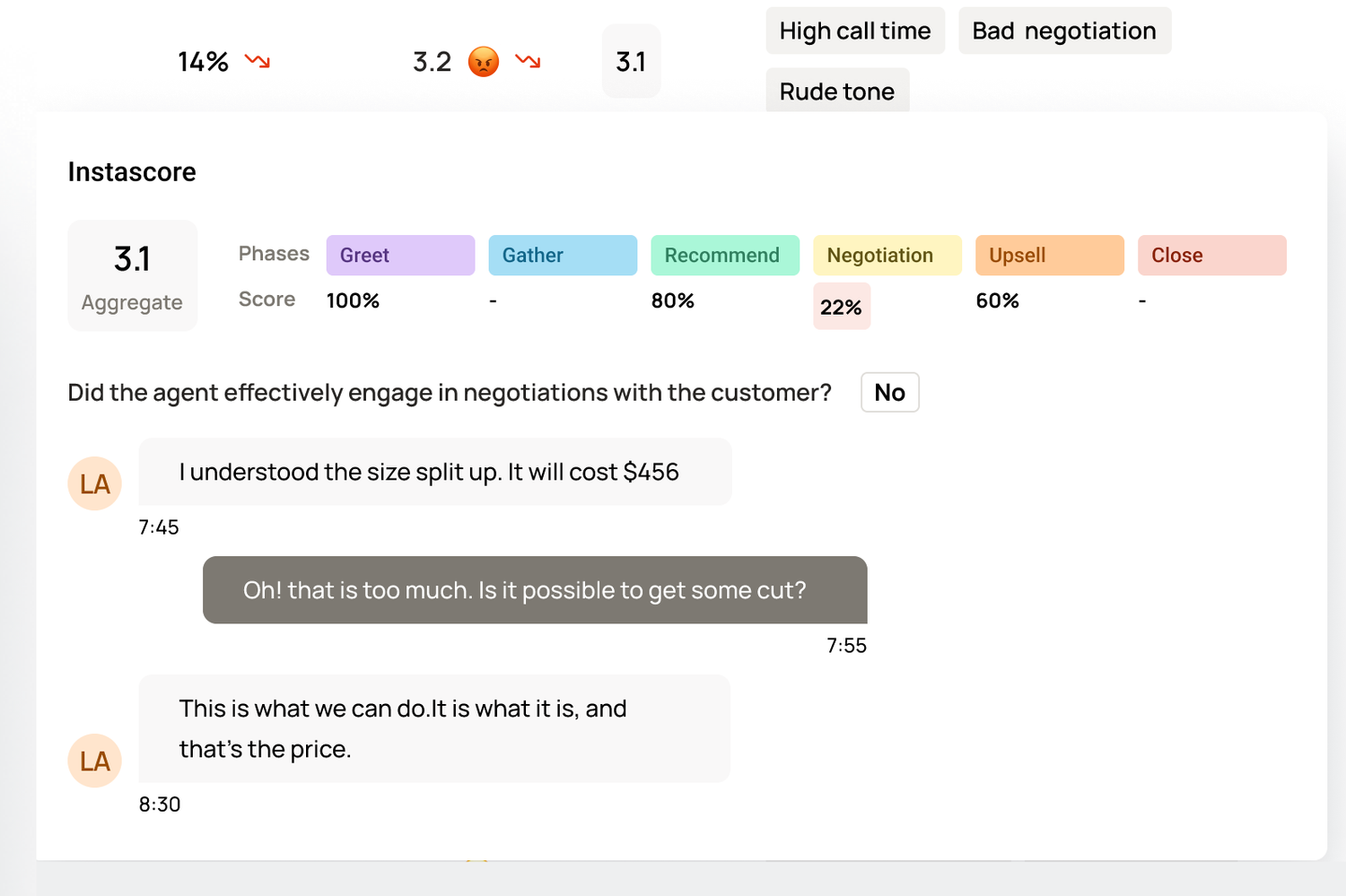
This contact center dashboard goes beyond traditional metrics like hold times and service levels to give you a richer situational awareness of live conversations, real-time insights, and a comprehensive view of call center performance.
Should you decide to intervene, the details provided minimize the amount of context you’ll need from the agent, so they can provide uninterrupted service.
Elevate Your CX Strategy with AI-Powered Insights
Level AI empowers contact centers with intelligent dashboards that transform raw data into actionable insights, helping to turn data points into opportunities for growth.
Schedule a demo today to learn how Level AI’s analytics dashboards optimize your quality management with AI-powered efficiency and precision.
Keep reading
View all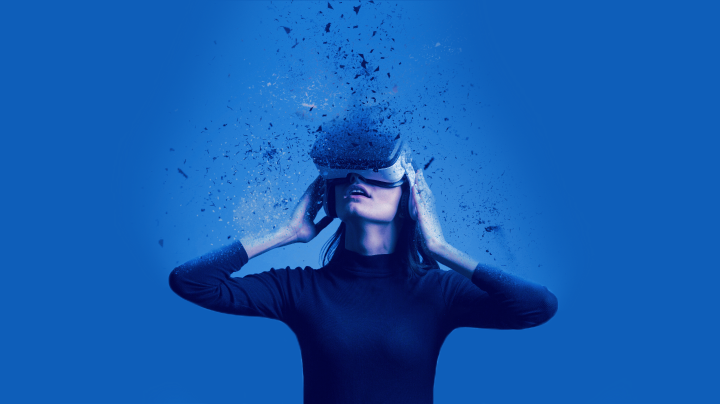
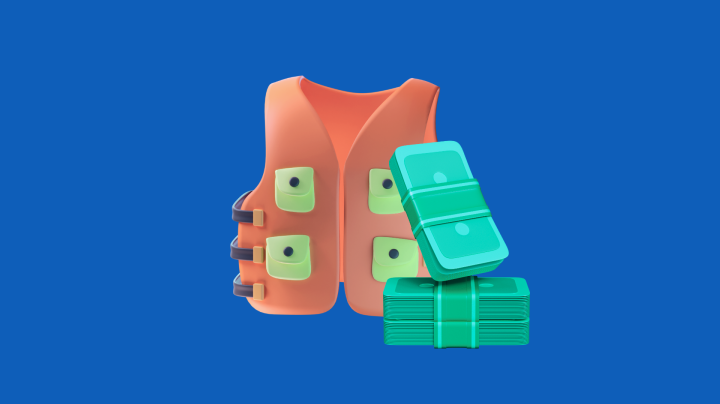
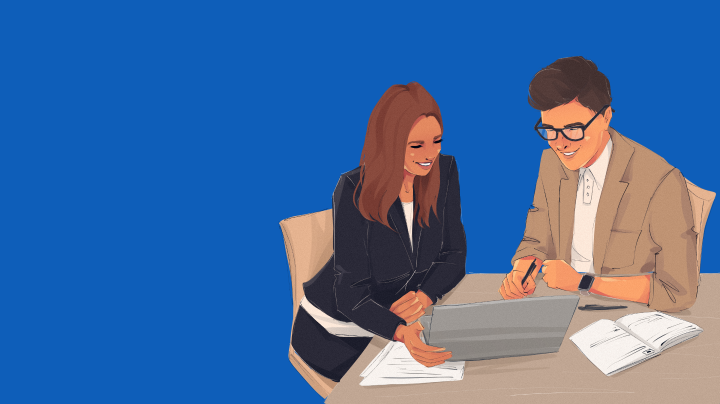