How Call Centers Can Leverage Voice & Speech Analytics

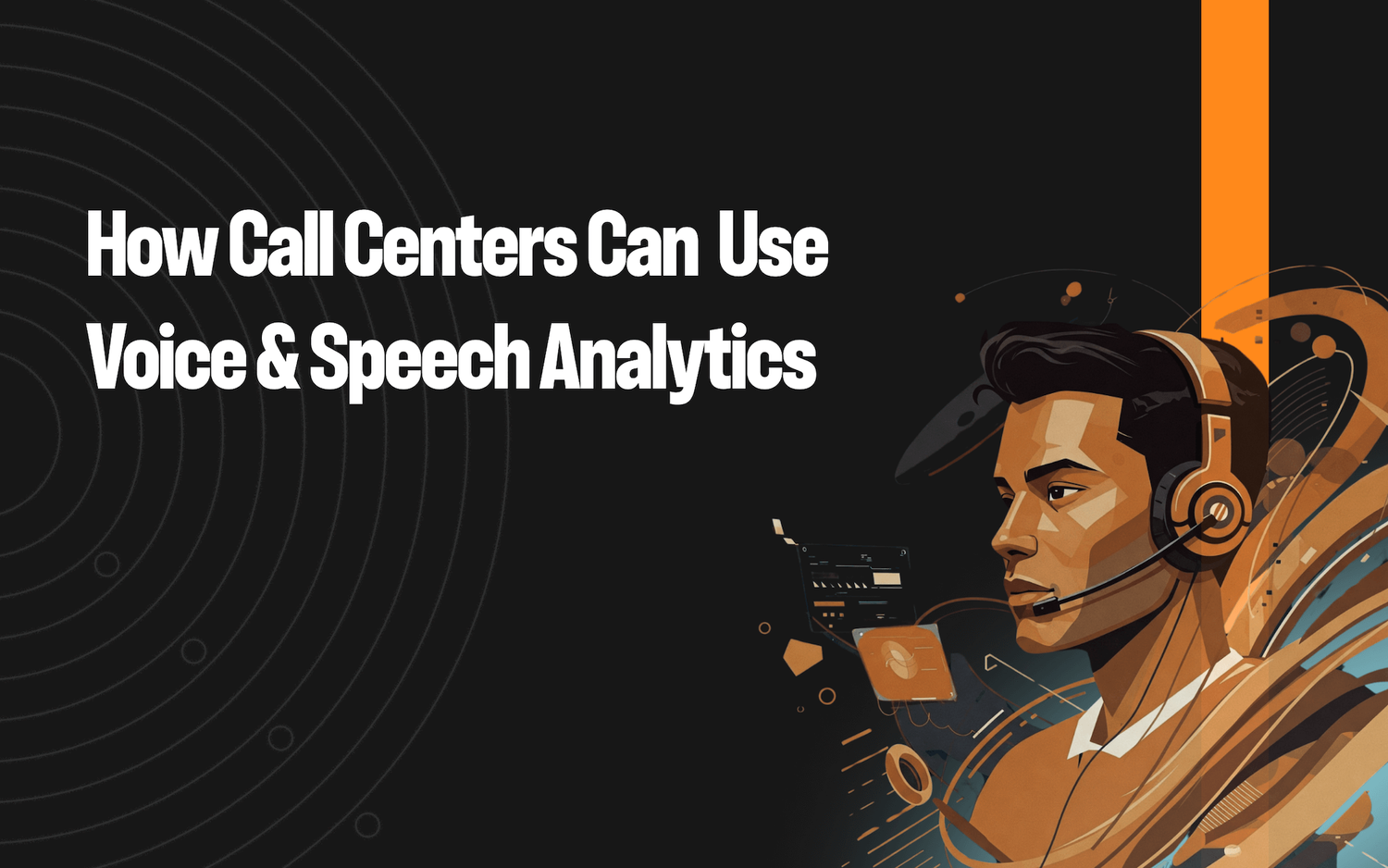
Voice analytics decodes speech to detect patterns in tone, pacing, and inflection that reveal insights into speaker intent, sentiment, and other elements that predict call outcomes and escalation risks.
Speech analytics solutions, on the other hand, analyze the content of what people are saying to deliver insights based on that information.
Speech analytics is useful for call centers because it captures the actual words and phrases spoken by customers and agents, allowing for precise issue detection, intent recognition, and trend analysis to improve customer interactions.
With speech analytics tools, call center teams can:
- Automatically capture call drivers for 100% of interactions
- Understand the “why” behind customer satisfaction scores
- Provide personalized training to every agent that goes beyond the limitations of manual quality assurance and incomplete feedback
- Increase agent productivity by automating repetitive, manual tasks
- Provide real-time support for agents & managers to improve customer satisfaction
In this guide, we explain how call center speech analytics works through various use cases, illustrate them with examples from Level AI — our customer experience software that leverages secure, customizable generative AI to analyze 100% of conversations, automate QA, and improve agent performance.
How Call Center Speech Analytics Software Works (And How AI Makes It Better)
Call center QA teams can typically only review 1–2% of all phone calls, meaning they may miss potential issues, emerging trends in customer call topics, and opportunities to improve customer experience.
Speech analytics solutions, on the other hand, allow them to evaluate customer calls at scale and deliver accurate insights into customers’ goals, emotions, and opinions.
These also help identify existing customer service gaps, potential self-service options to deflect repetitive calls, changes across customer query trends, and more.
Speech analytics solutions rely on automatic speech recognition (ASR) to do that. ASR transcribes spoken language by turning an audio signal into written text and uses speech-to-text (STT) transcription in real-time.
It also relies on advanced machine learning models to filter out background noises and accurately transcribe interactions with speakers that have various accents or use particular dialects.
In order to analyze those interactions after they’ve been transcribed, speech analytics solutions use natural language processing (NLP) and artificial intelligence to understand what’s being said, including intent (what speakers are trying to achieve) expressed by both customers and agents.
AI-powered solutions are able to capture context and meanings more accurately than rule-based algorithms that look for keywords in text.
For instance, for product returns, keyword-based solutions may accurately identify “return” and “send back,” but miss “mailed back” because it wasn’t included in a predefined list of keywords (and because of the lack of understanding of context).
AI solutions, on the other hand, understand both context and intent in conversation, automatically giving call center teams actionable insights about customer pain points and sentiments, and uncovering existing service gaps.
They also help call center agents and managers work more efficiently to improve the customer experience by surfacing the relevant information in the moment they’re needed.
We outline the use cases below, detailing what call center speech analytics enables you to do:
Detect What Customers Really Mean & Feel
Analyzing speech to understand what customers are saying and how they’re feeling allows call centers to detect frustration, confusion, or dissatisfaction in interactions. This allows teams to pinpoint common issues customers face, such as product defects, service inefficiencies, or gaps in agent knowledge.
Detecting negative sentiments expressed by customers early on allows customer support teams to take corrective action, for example, reaching out proactively with personalized offers or support to increase retention.
Speech analytics solutions also allow contact centers to detect recurring themes in customer conversations, such as common inquiries, interest in particular product features, or preferred communication channels.
Insights from sentiment and intent data can also be used to optimize call routing, reduce AHT, improve FCR by matching customer needs with the right agents, and generally promote call center quality assurance best practices.
Below, we show examples of how an AI-powered speech analytics solution uncovers customer intent and emotions from Level AI.
Capture & Analyze Call Drivers
Level AI’s Scenario Engine captures intent (what customers are trying to achieve) and refers to these as scenarios. It recognizes phrases spoken during call center conversations as indications of certain scenarios, and tags them accordingly with conversation tags.
This approach is based on natural language understanding (NLU).
NLU can classify calls and intent expressed by customers with near-human accuracy, regardless of which specific words are used to express their goals or frustrations.
Level AI comes with a number of predefined scenarios out-of-the-box but you can also define your own business-specific scenarios with example phrases that customers are likely to use in conversations, and by adding them to the list of available scenarios:
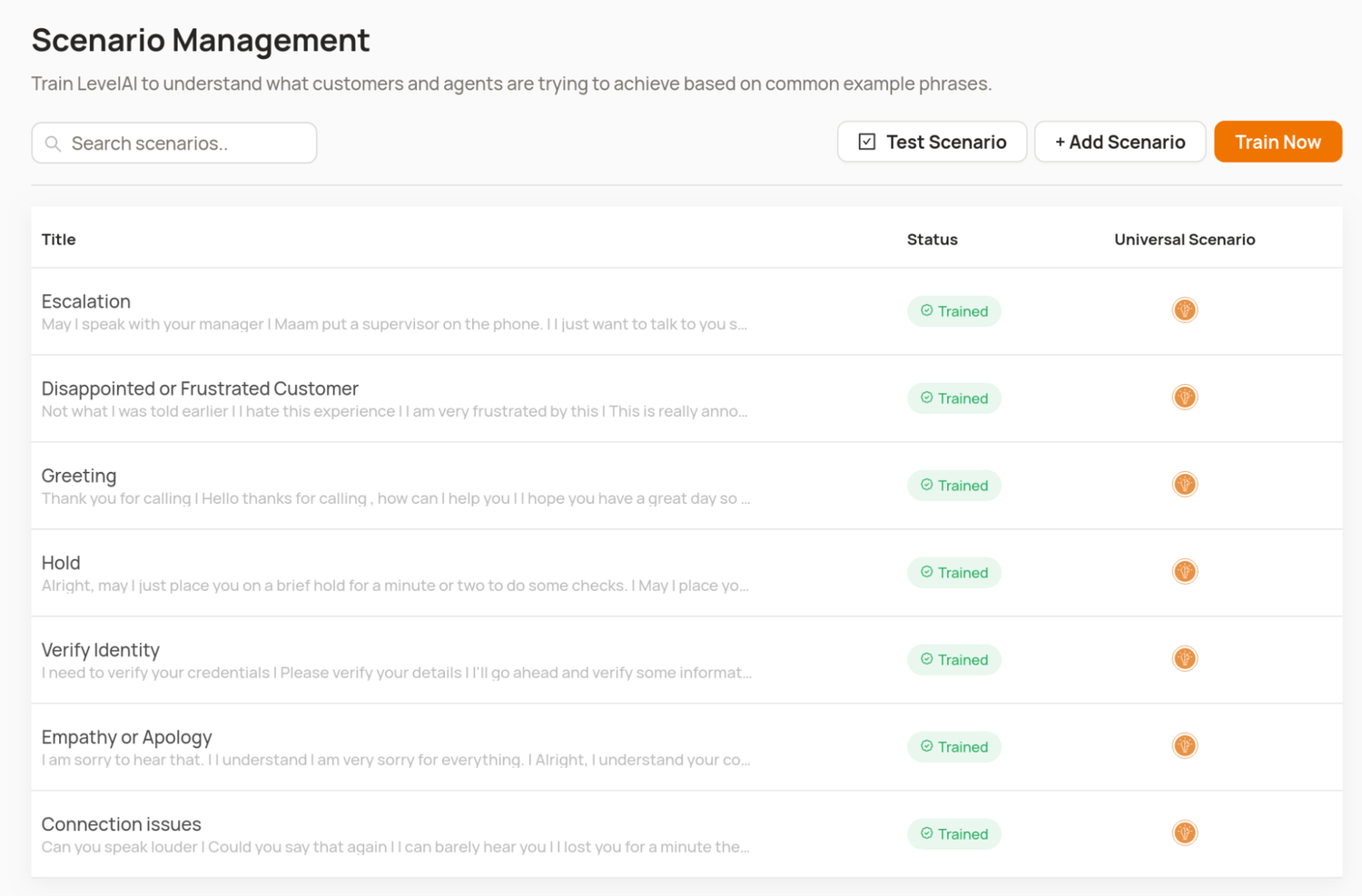
Every conversation analyzed by our Scenario Engine is tagged with multiple conversation tags to indicate text sections that are relevant to specific scenarios.
These conversation tags are searchable, allowing you to display all customer interactions where a particular customer intent was expressed. By running those searches, you can find and review all calls containing the same conversation tags.
This can help you find ways to improve customer experience. For example, reviewing calls where customers are asking for assistance with installing software can help determine the best way to reduce the volume of those calls through better documentation, a new knowledge base entry, or a more comprehensive training for agents.
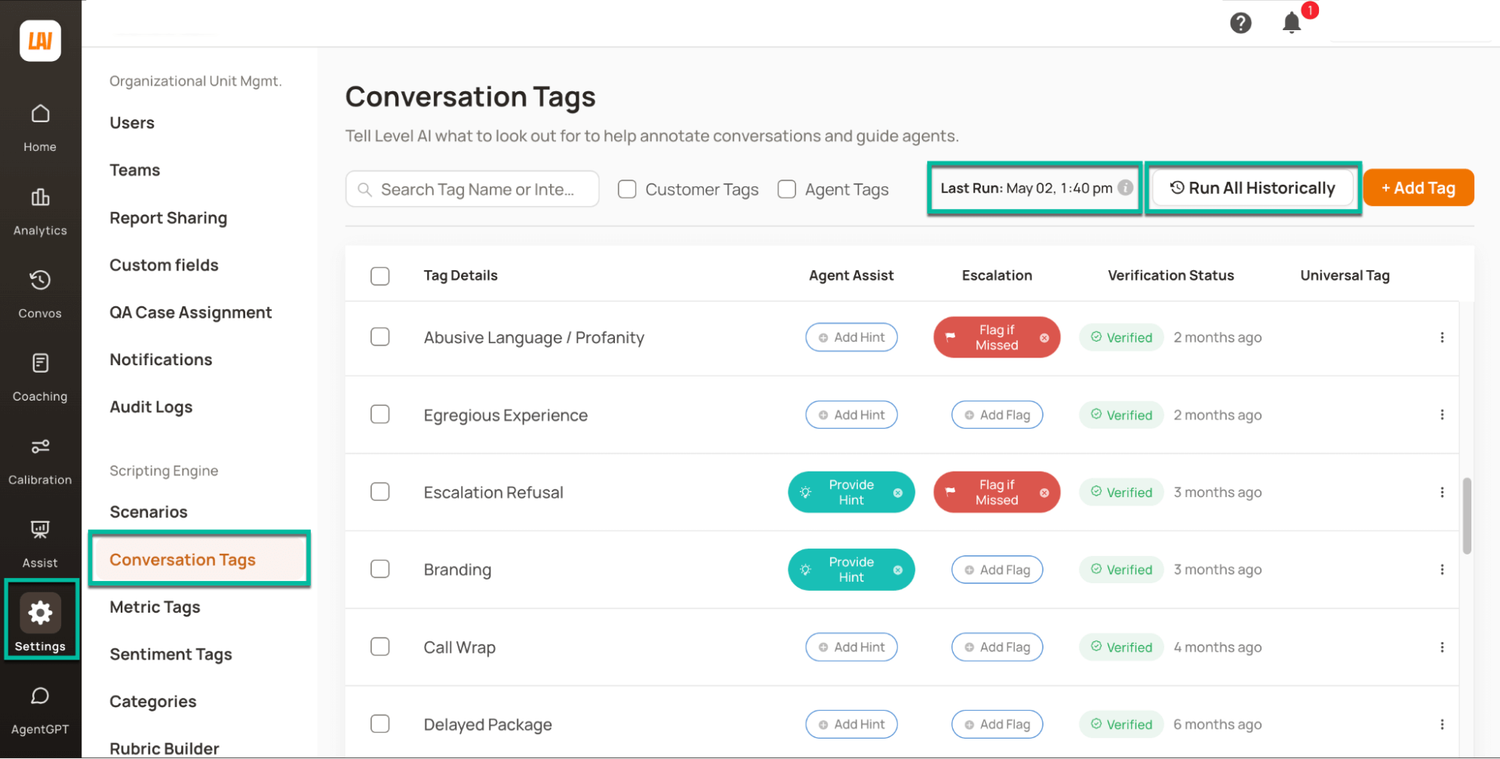
Understand Customer Emotions Through Sentiment Analysis
Level AI also identifies sentiments, expressed by customers and agents during the call and tags those instances with sentiment tags.
Our sentiment recognition captures the highest number of emotions across available software solutions in this category. These emotions include: happiness, worry, disapproval, disappointment, annoyance, anger, gratitude and admiration.
Applying sentiment tags helps understand customers’ reactions in more detail than simply recording their intensity, or whether the feelings of a customer were positive or negative, as a result providing more in-depth insights.
To make call analysis easier, Level AI also assigns an overall Sentiment Score to each conversation, based on emotions expressed by the customer throughout the call:
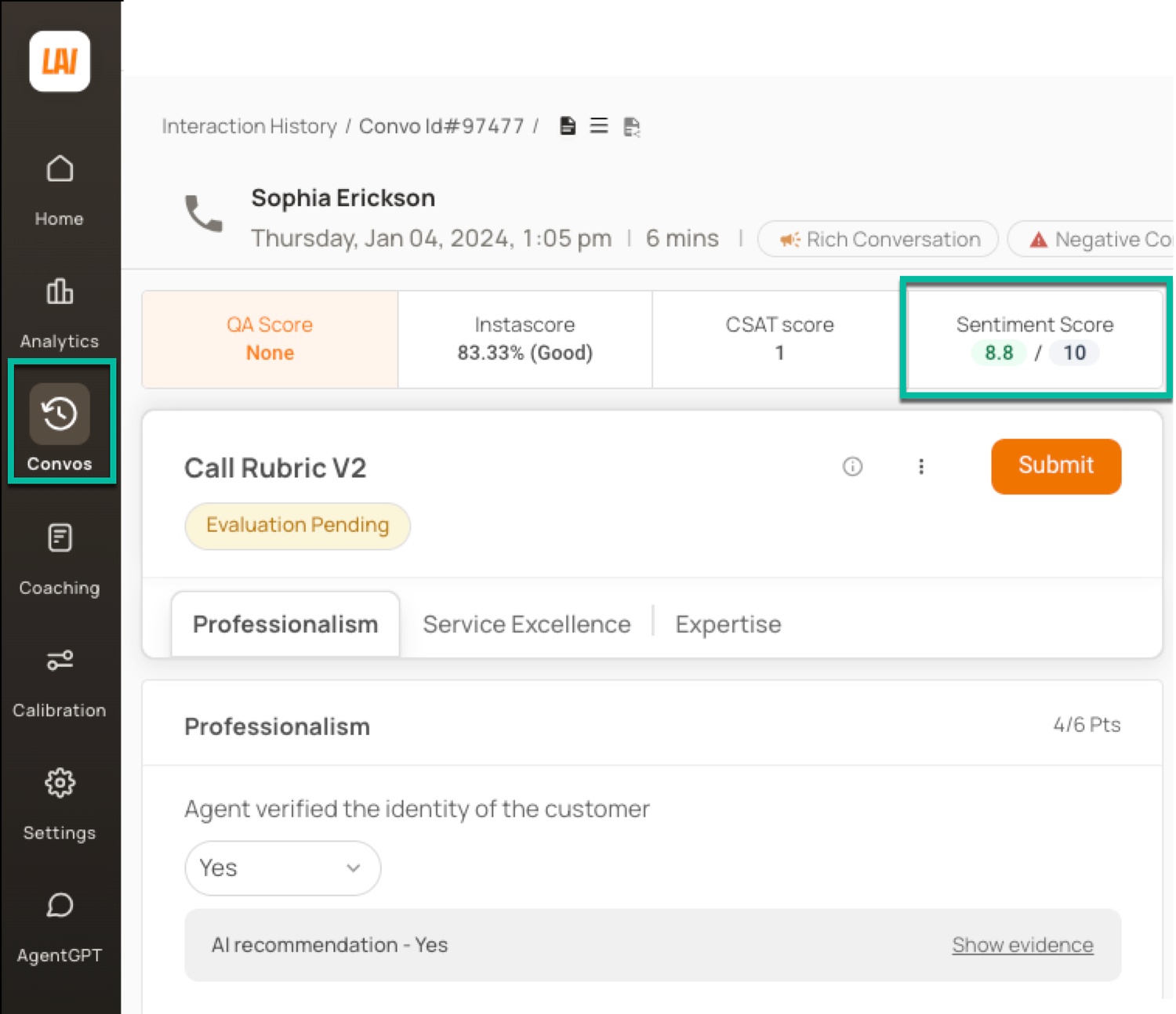
The Sentiment Score can range from 0 to 10 (the higher, the better) and reflects both the intensity and the type of the sentiment.
Emotions expressed at the end of the call are weighted more heavily than the ones expressed at the start of the call, since satisfaction or dissatisfaction with a call outcome is more likely to influence the way a customer will think about a brand in the future.
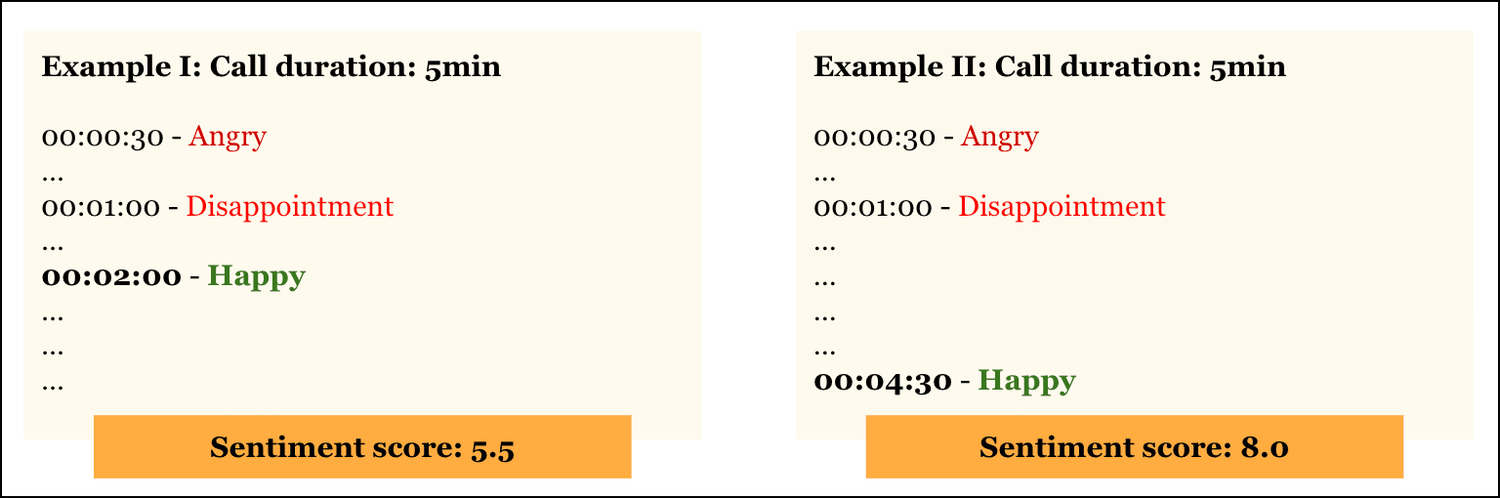
Understand Customer Satisfaction & the “Why” Behind It
Speech analytics solutions also allow call centers to compile high-level data on performance indicators like call volume, CSAT, AHT, and call duration — collectively known as voice of the customer (VoC) analytics.
VoC analytics typically incorporate both explicit feedback (such as surveys or customer reviews) and implicit signals (based on the call recordings and sentiment analysis) from customers.
While metrics like call volume, AHT, and call duration can provide a baseline for understanding call center performance, they’re rather coarse measures that don’t make it easy to find specific improvement opportunities.
This is where NLU and generative AI come in, as we explain below using several examples:
Uncover Customer Pain Points & Trends with VoC Insights
Level AI’s Voice of the Customer Insights (VoC) identifies subtle and emergent trends in customer interactions before these escalate into churn. Such trends may be spread across multiple conversations in ways that aren't immediately obvious without being surfaced by AI.
An example might be customer complaints around payment issues.
VoC Insights might reveal a pattern of customers being confused about the existing payment process. Without fully analyzing all conversations, such issues may be individually perceived as routine billing questions.
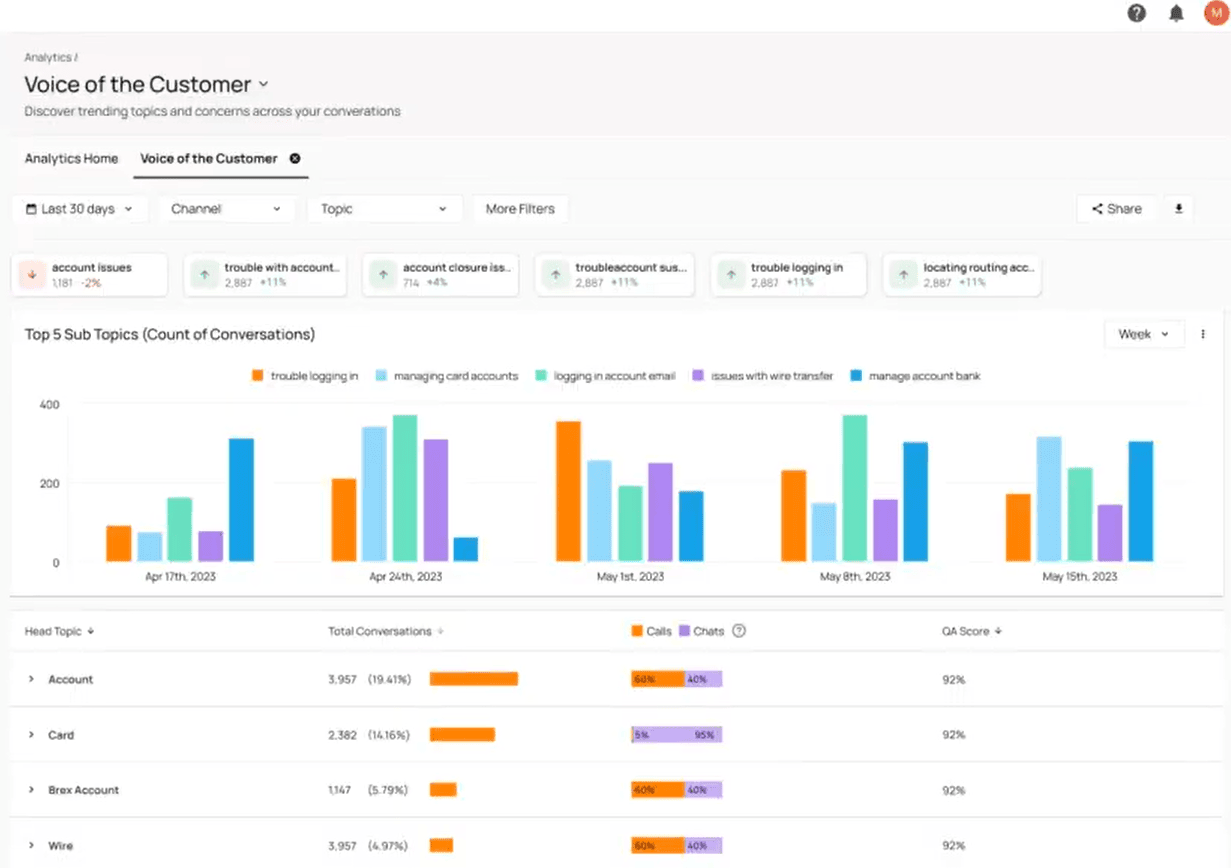
Besides extracting standard metrics like CSAT, NPS, CES, and AHT from customer interactions, VoC Insights displays and groups individual patterns that it detects in interactions as topics of interest.
It displays these in a number of intuitive and easy to use dashboards.
Fully Capture Customer Satisfaction with iCSAT
Our VoC Insights also includes iCSAT, or inferred customer satisfaction score.
While standard CSAT directly ties to the way customers feel about their experience, it’s nonetheless typically limited by sample sizes and its association with a single moment or conversation.
As a rule, not all customers will respond to a survey after their issue has been resolved. Customers that were either very happy with the outcome, or disappointed with it are more likely to respond to surveys, skewing the results.
(Read more about survey software in our latest 8 Top SurveyMonkey Alternatives.)
iCSAT is a more holistic measure of satisfaction, as it’s based on 100% coverage of customer interactions and takes into account data across three disparate areas:
- Sentiment Score, to detect the emotional tone of the conversation, taking into account the way customers’ feelings change throughout the conversation.
- Customer effort score, to evaluate how hard it was for the customer to resolve their issue, for example, whether or not they had to be transferred, were put on hold, or repeated themselves multiple times.
- Resolution score, to indicate whether or not their issue was eventually resolved (either fully or partially).
By combining these three factors, Level AI provides a more comprehensive and accurate view of customer satisfaction:
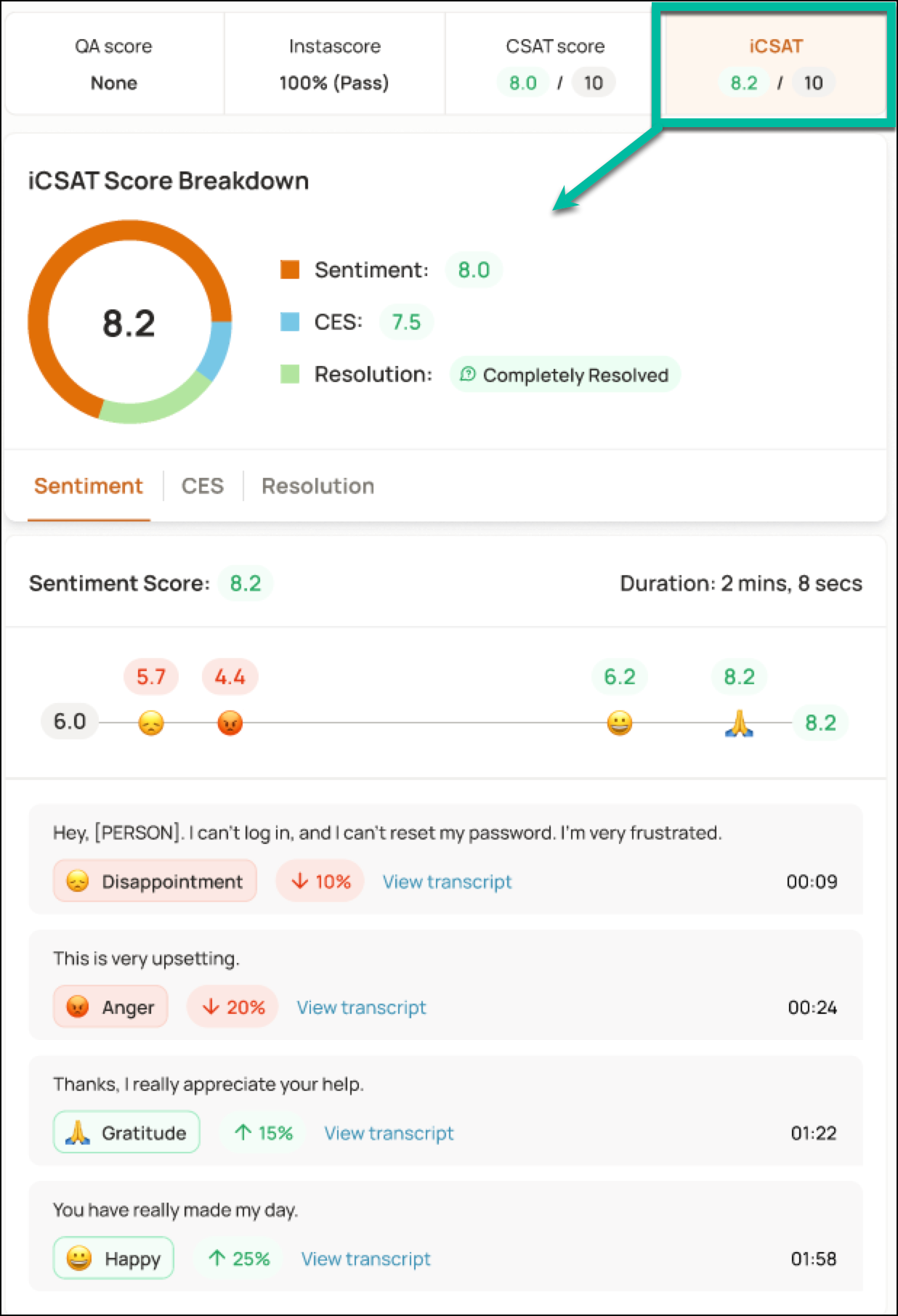
Unlock Insights & Performance Gaps with In-Depth Reporting
To help call centers understand the “why” behind customer satisfaction scores and improve customer experience, Level AI reporting capabilities combine both agent conversations and data imported from other sources, like chat transcripts, ticketing systems, or your CRM.
This way teams can better understand customers and their issues on the basis of unified data, rather than from incomplete, siloed information.
Here are some examples of questions that you can answer with Level AI reporting capabilities:
- Are there any recurring themes in call topics with high levels of customer dissatisfaction, for example, delays in shipping or complicated self-service flows?
- Are there any specific agent behaviors that correlate with higher levels of customer satisfaction at the end of a call?
- Are there any specific issues frequently left unresolved that require follow-up?
With our Query Builder, you can create custom views to answer these questions:
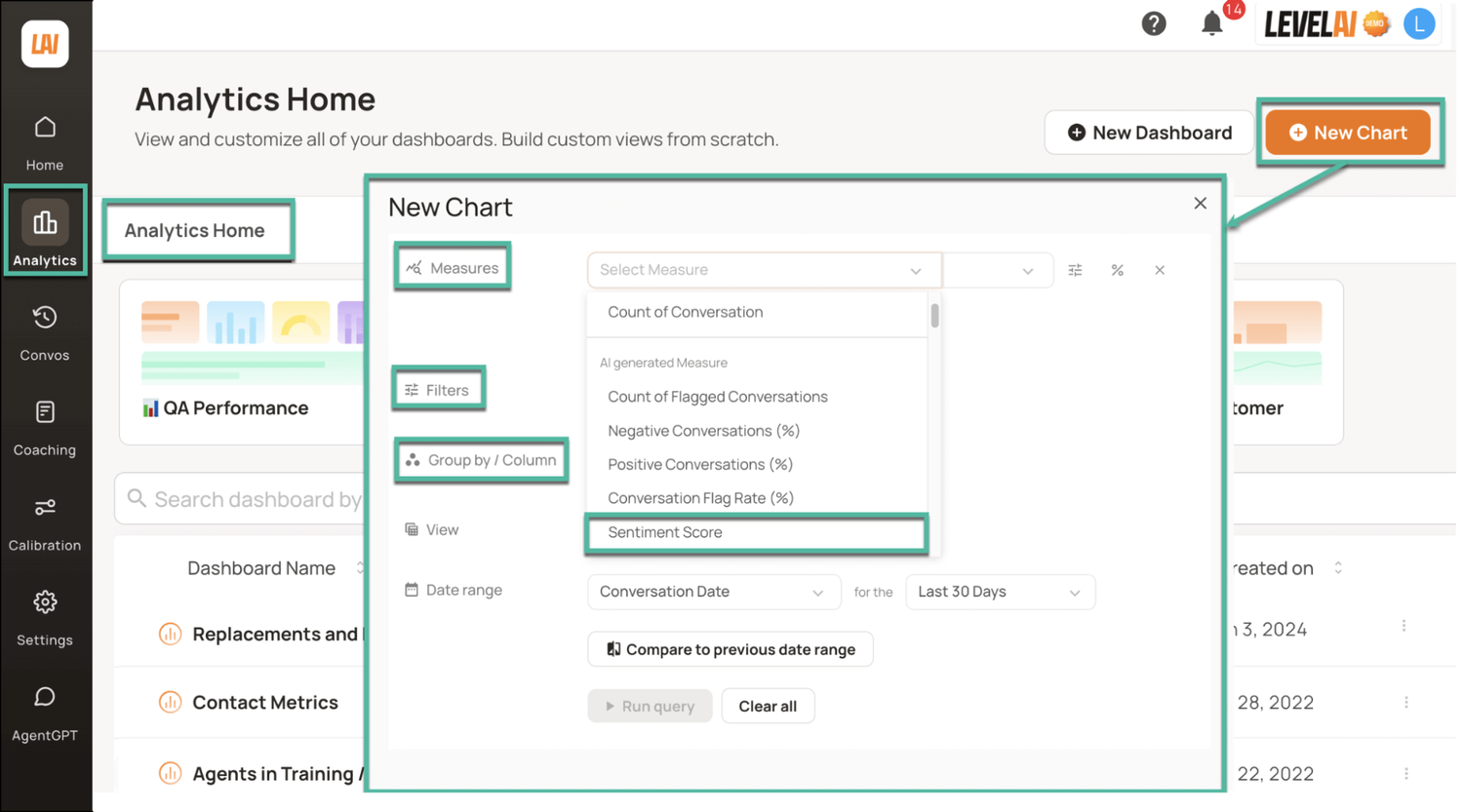
The resulting custom dashboards can be saved and shared with anyone in your organization — via role-based or user-based access.
Getting granular insights and uncovering customer frustrations directly from customer calls has helped Level AI customers improve their self-service options to deflect over 500,000 over a holiday weekend peak call time, reduce call resolution time by 20% and increase first-call resolution rates by 15%.
Provide Personalized & Effective Training for Every Agent
Speech analytics identifies patterns in agent performance, customer interactions, and overall conversation dynamics to provide insights into agent behavior, sentiment analysis, and conversation outcomes. This includes:
- Performance scoring and benchmarking: Agents are evaluated based on predefined metrics like resolution time, customer sentiment, use of compliance language (or other rubrics), and empathy.
- Identifying training gaps: Speech analytics solutions can identify customer complaints and link them to root causes like gaps in agent knowledge or emotional skills training.
- Detecting compliance issues: Speech analytics can flag issues with script adherence or compliance, such as missing critical phrases or not following a certain script in general.
- Progress evaluation: Agents’ performance is tracked over time, enabling call centers to monitor the effectiveness of coaching and training.
In addition to uncovering instances where agents need additional support, coaches can use speech analytics to highlight positive examples of calls that follow best practices.
Coaches and call center managers can identify high-performing agents with high satisfaction scores and extract insights from their interactions, which can be a more effective way of sharing best practices.
Below, we show two examples of how our platform supports AI use cases in the contact center by providing effective and personalized training to agents.
Automate Agent Scoring Based on Rubrics
Level AI’s InstaScore automates call review and agent performance scoring, so that QA teams and managers can identify each agent’s weak points and help improve their performance.
Without speech analytics, QA teams and managers usually train agents on issues they personally found in a tiny percentage of reviewed calls.
This paints an incomplete picture of agent performance and likely misses many coachable moments. It also means many agents may not be aware of better ways that have been found to resolve certain customer issues.
InstaScore evaluates agent performance during every call based on predefined rubrics, helping track their performance over time.
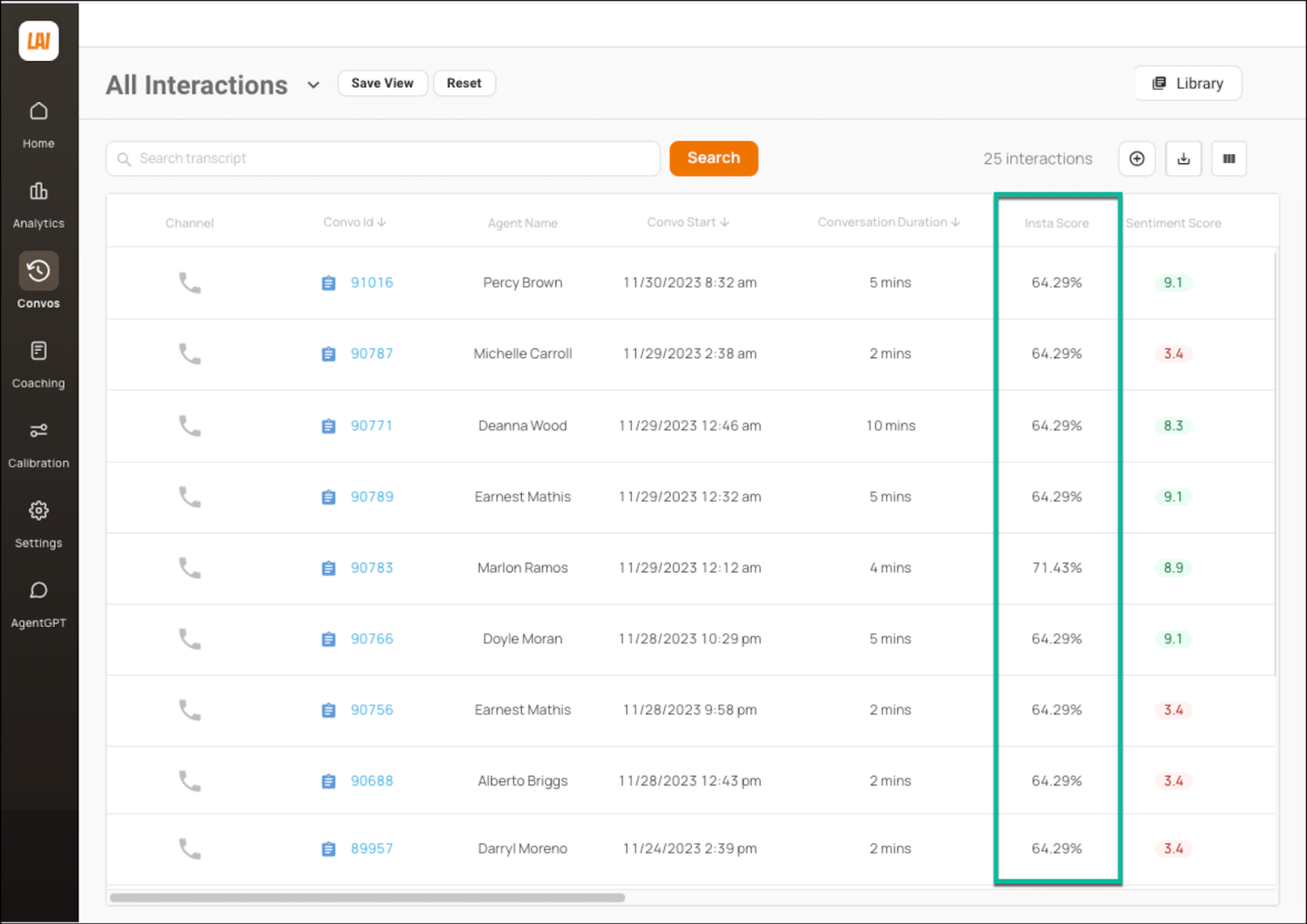
InstaScore is calculated based on these 3 factors:
- Verification of specific required behaviors (defined in rubrics), like greeting customers
- Quantitative measures based on voice analytics, such as appropriate tone or sentiment
- Metrics and parameters, such as long silences during the call
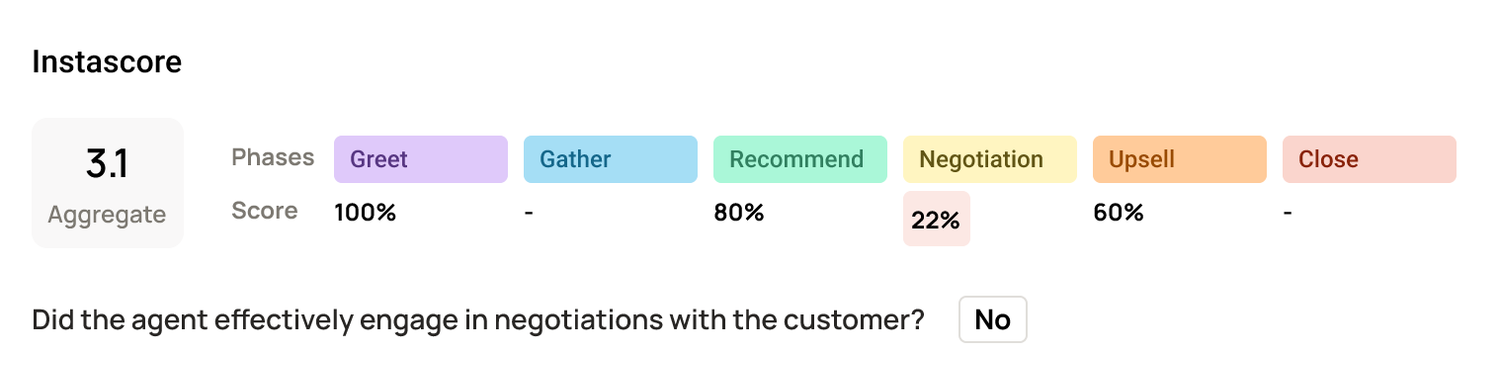
Highlight Calls That Need to Be Prioritized for Review
InstaReview highlights rich calls and negative conversations with a high number of flags (e.g., instances where agents don’t follow existing rules for issue resolution) as potentially crucial for your review.
This means QA team members and managers don’t need to waste time on reviewing the calls that are unlikely to yield any important takeaways, and can instead focus on conversations that are either problematic or have highly positive characteristics.
Rich conversations are selected based on the handling time overall, AHT, number of utterances and a high number of assist cards. These can reflect either positive or negative sentiment overall (with a Sentiment Score over or under 6 respectively).
Interactions that the system deems worthy of review are appropriately tagged, as shown below:
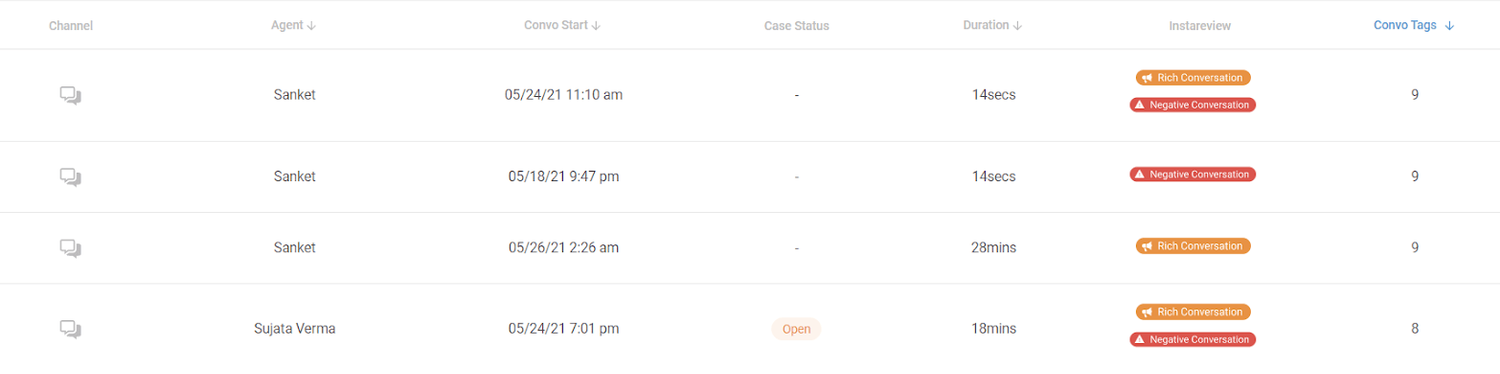
Increase Agent Productivity by Automating After-Call Work
Post-call work for call center agents normally involves completing call notes and categorization tasks, which can be time consuming.
AI tools for customer service use transcription and conversational analysis to improve agents’ productivity through post-call work automation. These extract key details of calls, such as customer issue, resolution, and next steps, and generate concise summaries automatically.
This includes identifying call outcomes and automatically classifying them into predefined categories. Below, we show how Level AI automates those tasks for call center agents:
Automate Summary & Resolution Status Generation
Level AI’s Smart Summary automatically generates call summaries, including follow-up actions, resolution status and overview of the interaction:
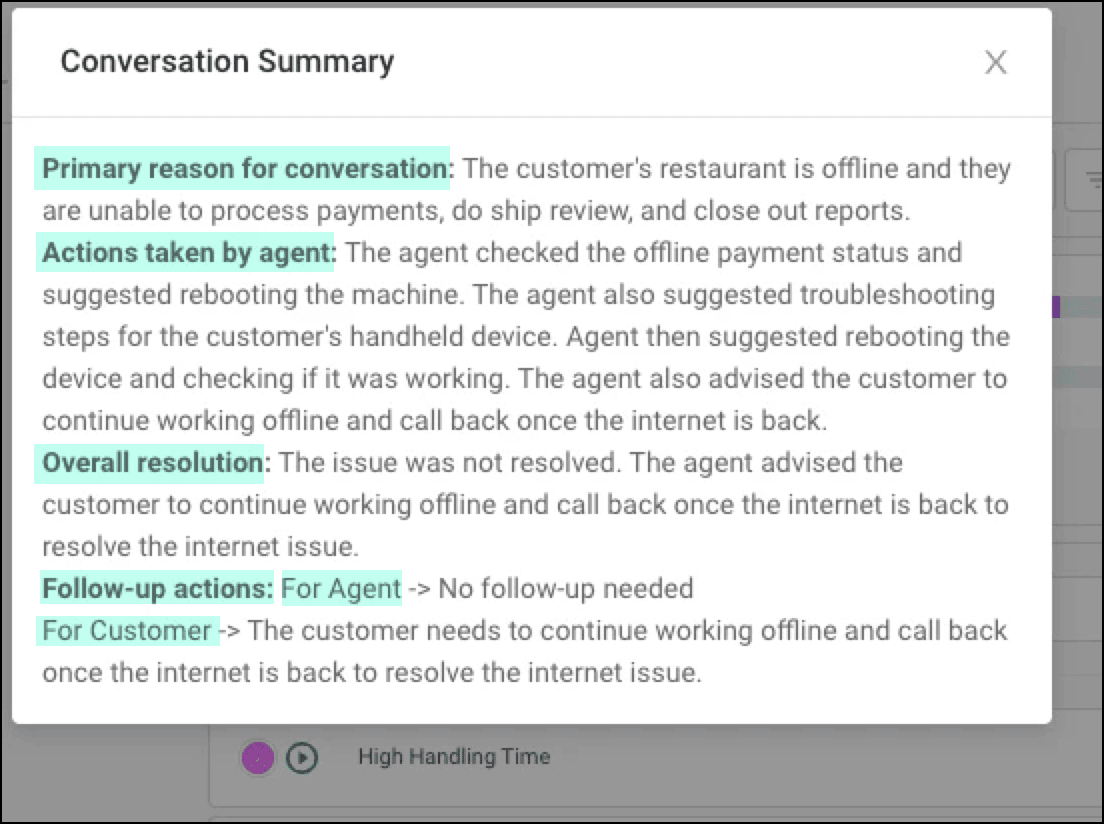
This reduces agents’ mental load, eliminates the need to complete post-call notes, and decreases wait time for the next caller.
Categorize Calls for Automated Dispositioning
Level AI assigns both categories and subcategories to calls for higher accuracy and displays past calls — already classified based on the type of interactions:

While Level AI includes out-of-the-box categories, you can also add business-specific categories by providing example phrases to train AI to recognize them.
Our system also suggests example phrases from past customer interactions that are “near misses” in terms of what it understands your category is about. You can either accept or reject these, further improving AI accuracy over time.
One of Level AI’s customers was able to decrease after-call work for agents by 75% by using these features.
Assist Agents & Managers in Real-Time
Real-time speech analytics help call centers improve agent productivity and prevent situations where customer satisfaction scores suffer.
Because speech analytics tools capture customer intent in real time, this data can be used to display relevant hints and prompts for each conversation.
Speech analytics solutions can also prompt alerts in cases where agents need support, so that customers can have their issues resolved faster.
Below we’ll share examples of Level AI features designed to support agents and managers in real time.
Provide Real-Time Assistance to Managers
Real-Time Manager Assist uses real-time AI speech analytics data like sentiment analysis, live speech recognition, and supporting metrics such as InstaScore to alert managers in real-time about calls where they may need to intervene.
Traditionally, managers needed to rely on simple metrics like call duration to determine whether a call was going well, to decide if they needed to step in or not.
However, call duration is not necessarily an accurate way to understand whether a call is actually going well (or if an agent needs help).
Sometimes customers have multiple issues they need help to resolve. And sometimes agents face new or complex issues they need to research in order to find a solution or workaround.
To make it easier for managers to know where they need to jump in, the Real-Time Manager Assist main dashboard shows an overview of ongoing agent conversations:

If necessary, managers can click on the metrics of the call center analytics dashboard to see additional information about the call:
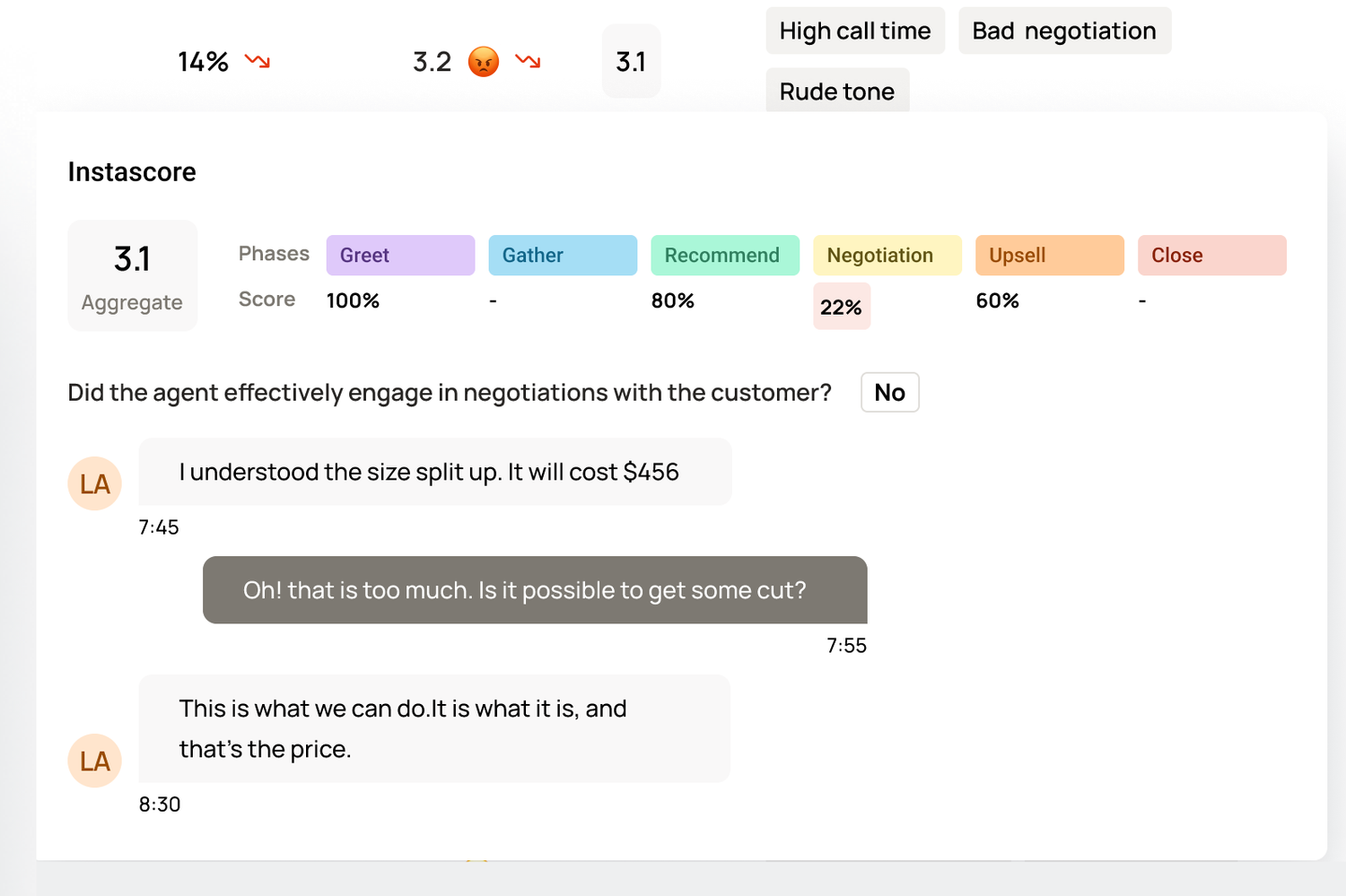
After that, managers can either use call whispering or call barging to intervene.
Support Agents in Real Time for Faster Issue Resolution
Level AI’s Real-Time Agent Assist uses natural language understanding and GenAI capabilities to guide agents through customer conversations by displaying relevant information throughout the conversation.
Having continuously refreshed information at their fingertips during live calls, agents can minimize putting customers on hold while they research or ask for help.
Real-Time Agent Assist displays a variety of data (Including action hints, warnings, and FAQs), and recommends resources from internal sources (for example, ticketing and knowledge systems) in real-time.
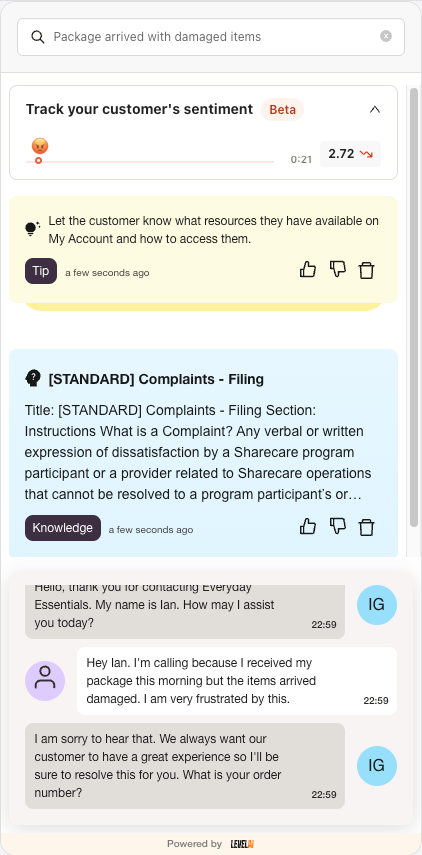
Real-Time Agent Assist also has a search feature — AgentGPT — that predicts which information agents will need based on the conversation, and automatically pulls in and summarizes relevant articles from multiple sources.
It also proactively fills the search tab with queries based on topics discussed during the conversation to help agents resolve issues faster:
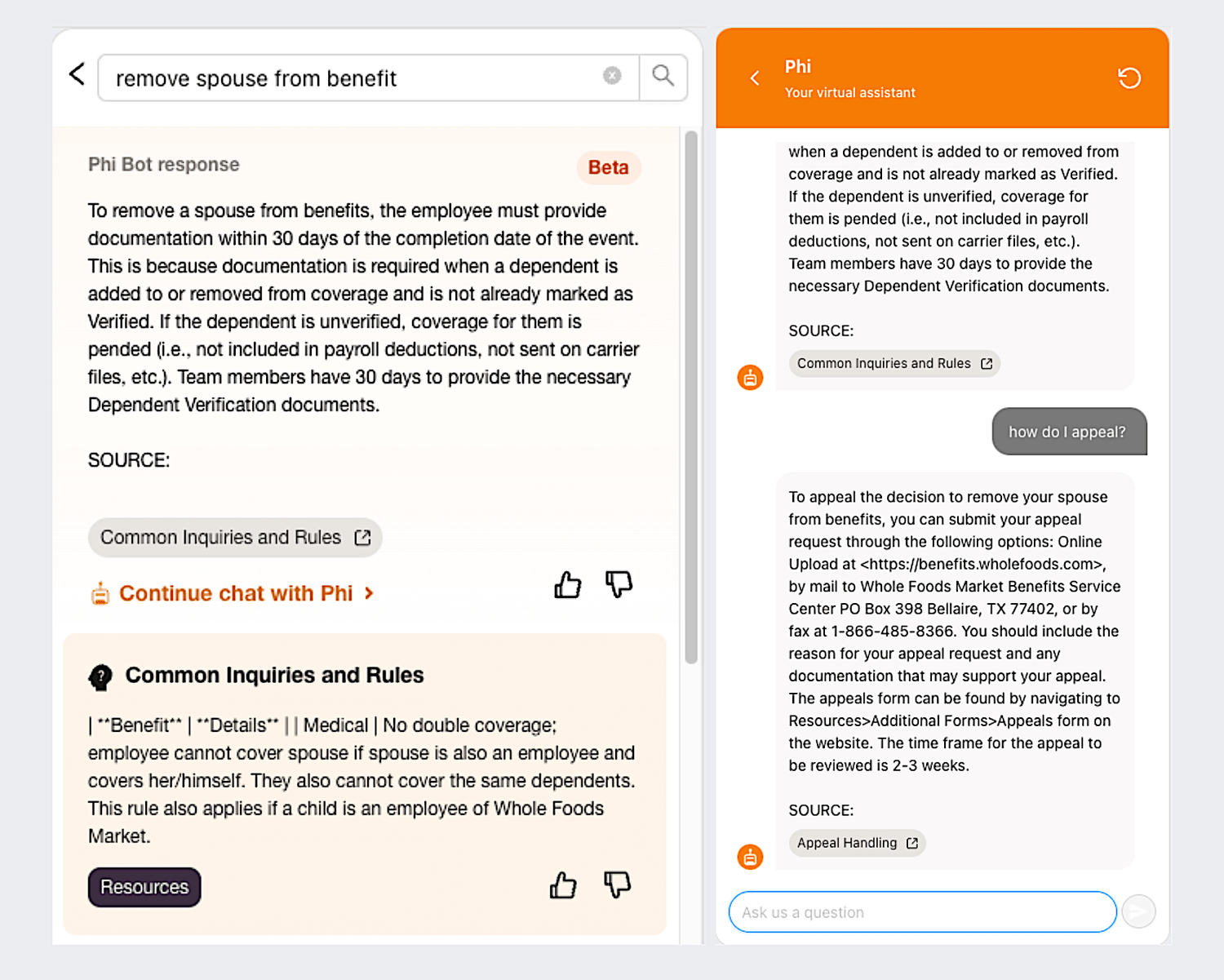
Level AI’s Call Center Speech Analytics Improves Performance
Automatically capturing both the meanings of conversations and the emotions that customers express during calls resolves many issues, like low agent productivity and limited visibility into customer call trends.
Schedule a Level AI demo to see how our platform provides better agent support in real-time and helps you achieve higher efficiency and customer satisfaction.
Keep reading
View all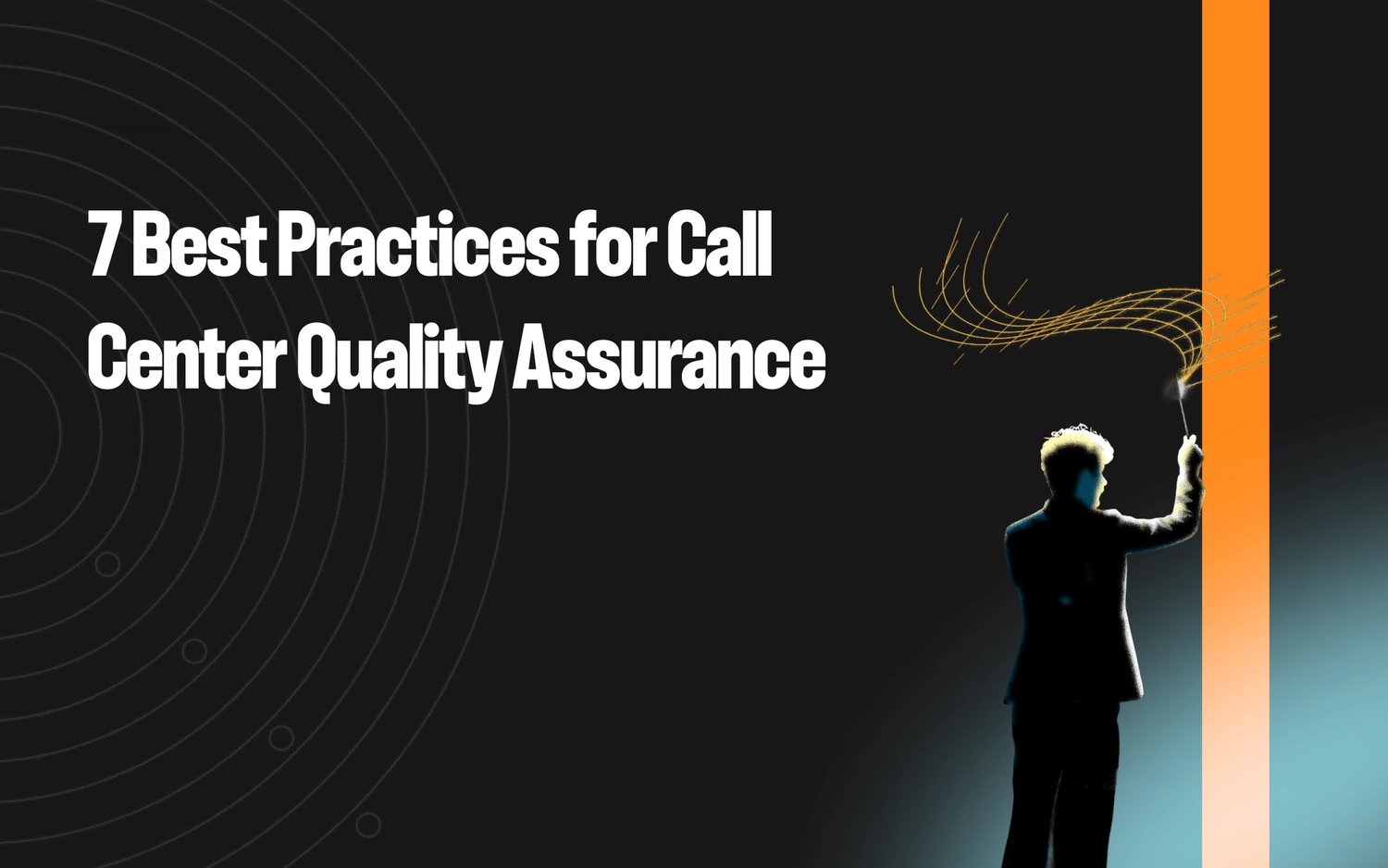
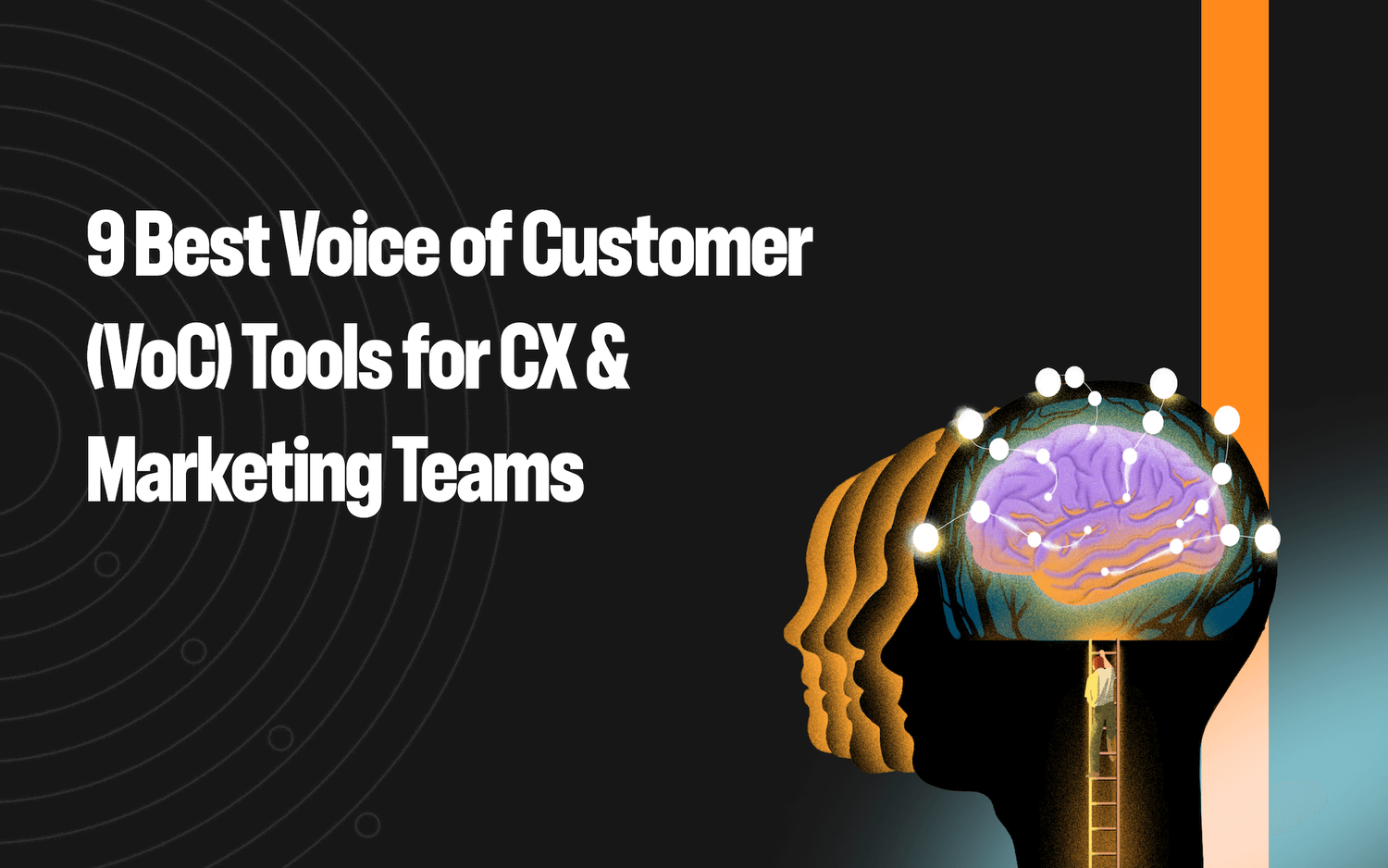
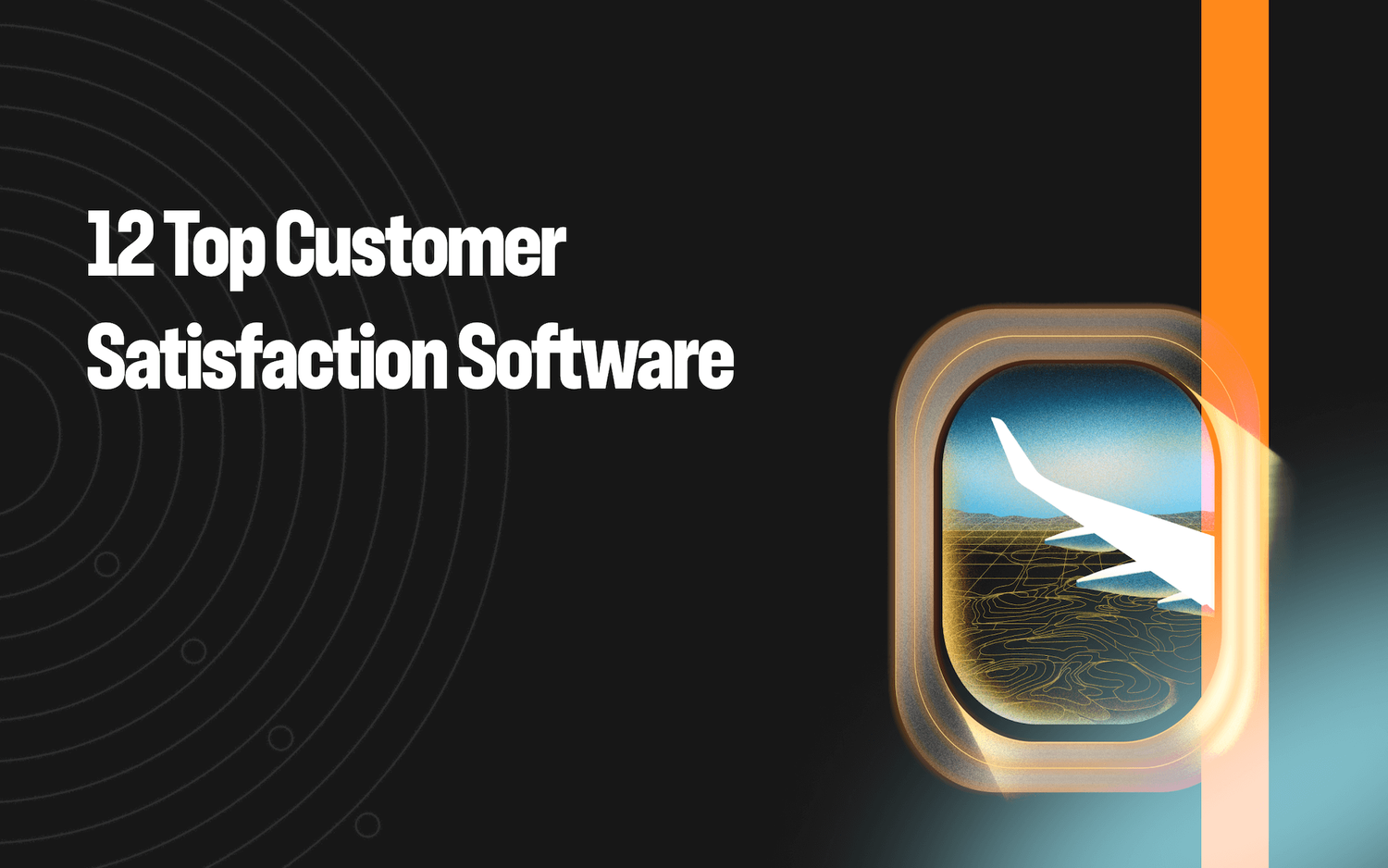